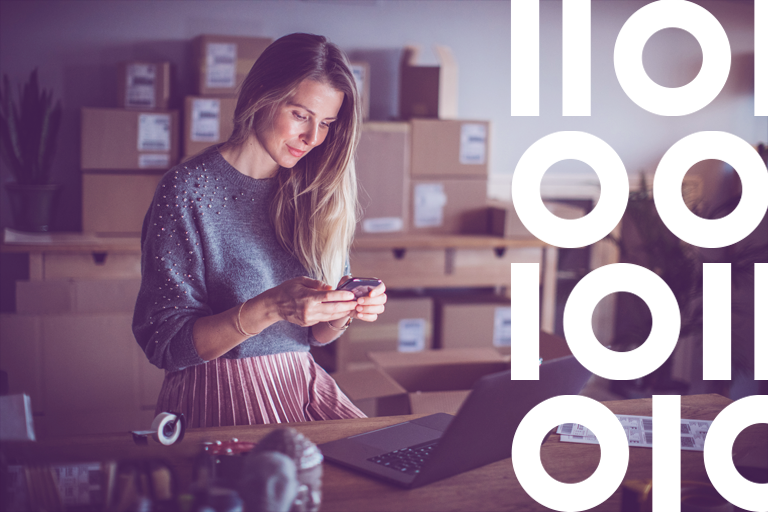
Top 10 Tips for Successful Retail Site Selection
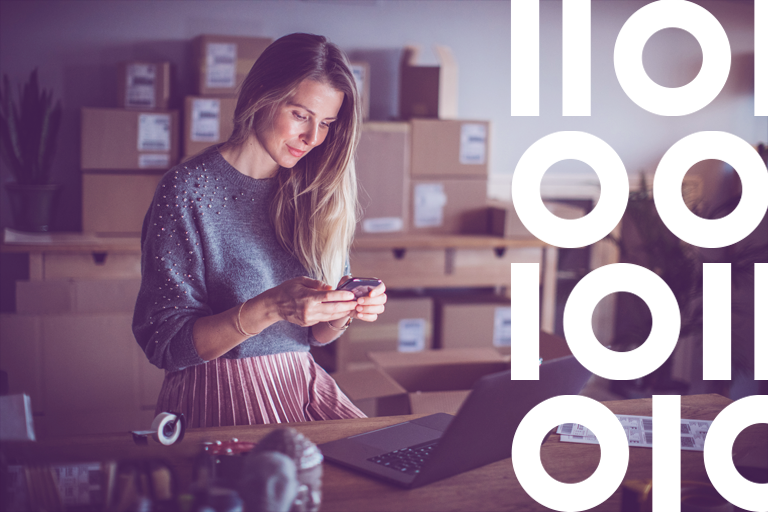
Assessing your retail network and generating an expansion plan requires diligence, data, and expertise. Here at Precisely we bring all three of these things together. As part of that journey here are 10 Tips for Successful Retail Site Selection from our experts in demography, location intelligence, and data science.
1. Verify the quality of your location data
Whether it is part of a robust sales forecasting model or to just display on a map, data location accuracy is critical in any retail site selection exercise. At Precisely we recommend running all location-based data through our geo addressing solutions. This gives our clients a level of confidence in the location data we are utilizing to build complex models. The reality is, whether you have 4 locations or 400, there is probably at least one location in your database that does not have the correct coordinates. This could mean missed opportunities and other impacts in spatial modelling.
2. Know your data vintages and align accordingly
Not all demographic data is created equally and often there is a mismatch in data releases and corresponding boundary releases. COVID further enhanced this through delays in census data capture, on a country-by-country basis. Make sure you ask any provider of demographics data what is the base year of their data and what set of boundaries the data is tied to. Our team of data experts alert our clients of any potential impacts and align data accordingly to its release date and the best corresponding boundary set.
3. Classify your locations by a density measure
Consumer behavior differs significantly within a market. One of the key factors driving this are changes in population and household density. By bucketing all your locations by density class (a measure of household density within 5 kilometers of any point) we can start to see patterns emerge such as how far a customer is willing to travel to your location, what kind of cannibalization is expected, and the sales forecasting implications. Here at Precisely we can classify your locations based on our seven density classes and let you know what that means for your network going forward.
4. Data harmony, leverage datasets across the business
Often our clients have disparate location datasets across their organization. Our team of retail location experts can take these datasets and make them actionable not just for Real Estate but also for marketing, operations, and other internal teams. Usually, the first place to start is a consistent view of the customer across your organization through one segmentation system or customer profile. Here at Precisely, we can verify the characteristics of your in- and out-of-profile customer and provide deep insights on how that can be used across your organization.
5. Generate ongoing store survey data
As any retail professional knows, location matters. This is especially evident when considering site specific characteristics (e.g., access, signage, visibility, store size). We have seen in our analyses that site characteristics can often impact a location’s sales potential by as much as +/- 20%. With that amount of impact, it is critical to consistently capture data at the store-level and regularly update for changes. We recommend consistency in any site survey questionnaire. We also advise that each site survey question has a limited number of responses to easily bucket stores for future modeling techniques and to also limit confusion for the respondent. Finally, when generating store survey data, it’s best to have a training session on expectations and the goals of the survey beforehand.
Read our eBook
Retail Channel Optimization in a World of Digital Transformation
This eBook explores how retailers rely on data science and analytics to enable decisions that bridge both the physical and digital and inform an omnichannel approach.
6. Understand your store maturity before building any models
You probably have a mix of store locations that are old and new. By comparing newer stores against stores that have been open longer, we can get a sense of the difference in growth and what defines a “mature store” within your network. This is critical as you think about building retail site selection models with this data and knowing the distinct differences between these two groups so you can provide multi-year sales estimates.
7. Understand where mobile trace data fits into the customer data pyramid
Mobile trace data has come a long way over the last 10 years. It has replaced many antiquated ways to collect customer data and is a very reliable and accurate way to understand the behavior of your customer to your location. However, mobile trace data often lacks the scale of a customer loyalty program and can’t tell you what a customer purchased or how much. Given the choice, we would recommend a robust customer loyalty program or point of sale capture, augmented with mobile trace data.
8. Set realistic expectations on cannibalization
Cannibalization can be very situational and often challenging to model. Our years of research has given us insights into some of the mysteries of cannibalization and the patterns that we have been able to identify by density, by market, and by retail type (convenience-based vs destination-based retailers). While a cannibalization model is often only as good as the data supporting it, our goal is to accurately represent cannibalization +/- 2%. This can be achieved through an abundance of historical observations and a consistent approach to analyzing the results.
9. Understand your modeling options and their strengths and weaknesses
There are many modeling options when it comes to building retail site selection and sales forecasting models. Ultimately, the data and the store observation count will dictate the best retail model to use. Also, it is important to know that you may not need AI or machine learning if it means you can build a superior model without it. While AI is gaining traction in the industry, it is still somewhat unproven and has its potential flaws. We consult with our clients to understand their expectations and goals for any retail site selection model. Our goal is to provide the best model with the best data to provide the best output.
10. Utilize multiple methods to access new sites
There will be different perspectives and expertise when approving and forecasting sales for a new site. The best approach is collaborative, where feedback is generated from multiple sources, and any model is used as a guide. Knowing what drives that tool or model is critical to understanding gaps and identifying where other data sources and inputs may assist. A sales forecast model is another seat at the table, not an autocracy.
To learn more about how data analytics can help you make better retail site selection decisions, read our free eBook Retail Channel Optimization in a World of Digital Transformation.