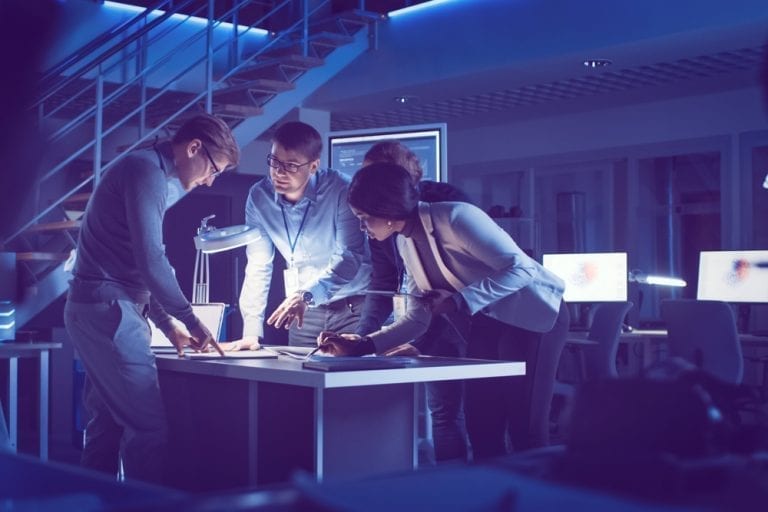
Building a Framework for Data Quality Management
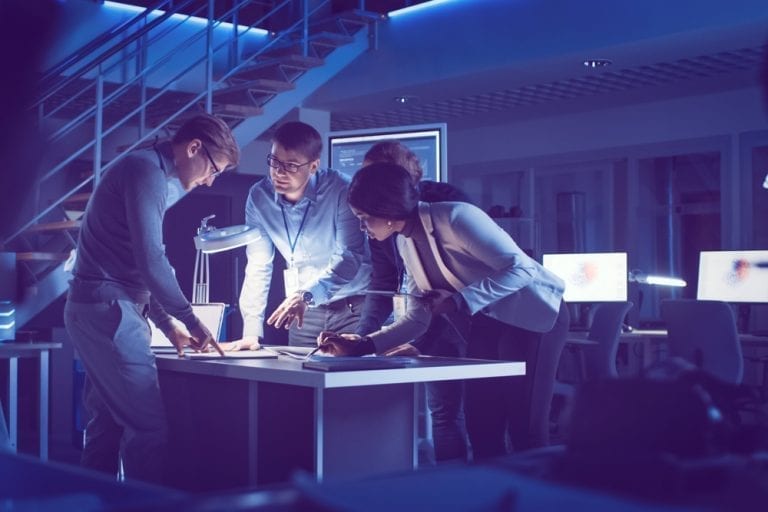
Data quality management has emerged as an increasingly important factor in determining the success of big data analytics and AI/machine learning initiatives. Even at a purely operational level, the quality of the data you maintain on customers, products, and suppliers is extraordinarily important. Evolving customer expectations around hyper-personalized experiences and consistent interaction across multiple channels (such as in-store, online, and customer service interactions) have highlighted data quality as a top priority.
Poor data quality has been a known issue for years. As the volume of data has skyrocketed and software systems to handle it have diversified and proliferated, the challenges associated with the consistency, accuracy, and accessibility of that information have likewise grown in both relevance and complexity.
To get a handle on data quality and to achieve the greatest possible benefits from data quality management software, business leaders should carefully consider their overall approach to the process. Precisely encourages companies to define a data quality management framework from which to begin building a more detailed strategy and tactical plan for achieving their data quality objectives. In doing so, leaders should keep in mind that data quality is not a “one-and-done” proposition. Rather, it is an ongoing practice implemented around a philosophy of continuous improvement.
Here is a four-part framework that many companies have successfully adopted as a starting point for continuous data quality management:
Step 1: Define goals and objectives regarding data quality
As best-selling author Stephen Covey often reminded his readers, it is always best to begin with the end in mind. What are the costs that can be attributed to poor data quality in your organization? Is your marketing department wasting money promoting your products or services to the wrong customers? Are shipments being delayed or returned due to address errors? Are products being misrepresented on your website, resulting in lost sales or dissatisfied customers?
The quality of customer data decays at a somewhat predictable rate. What is the impact of the resulting decline in data quality for your organization? Are you currently measuring the costs of poor quality? If not, how might you begin doing so? Until you have a clear understanding of what the problem is costing you, it is difficult to build consensus around a plan for improvement.
The negative effects of poor data quality are compounded as organizations begin to deploy advanced analytics to drive strategic business decisions. Artificial intelligence and machine learning are beginning to achieve critical mass as a mainstream tool for generating business value. But if algorithms are trained based on erroneous information, the long-term negative effects on critically important business initiatives aimed at building competitive advantage could be devastating.
A sound data quality framework must begin with a clear definition of the desired outcome, taking into account the current state of existing business data, potential future IT initiatives, legal and compliance issues, and the impact of poor data quality on customer loyalty.
Read our eBook
Exceeding Expectations: Four Ways Data Quality Promotes Customer Loyalty
Learn how you can leverage data quality to facilitate and enhance opportunities to improve customer engagement and loyalty.
Step 2: Identifying areas of opportunity
As business leaders come to conclusions about their data quality goals and objectives, it is likely that specific areas will emerge as priority needs for the organization. Customer data often rises to the top of that list, simply because the quality of such data erodes at a somewhat predictable rate and because customer data is central to the mission of any business.
Depending on the nature of your business, some other areas are likely to also emerge as priorities. Insurance businesses that write homeowners policies, for example, must have accurate and up-to-date information regarding properties they insure. Companies with a large catalog of products must be especially concerned with the consistency and accuracy of item master data and inventory quantities.
Once priorities have been established, companies should assess those priorities against their organization’s current capabilities. Which high-priority data quality problems might be addressed most easily? What is the proverbial “low hanging fruit” that could result in a high return on resources invested? As with any project, leaders must work with stakeholders to establish which activities and investments will result in the greatest benefit.
Step 3: Implement improvements
After the key opportunities have been identified, business leaders should develop a project plan for implementing those highest-priority data quality initiatives. To achieve that efficiently and at scale, organizations need enterprise-grade data quality management software. Although piecemeal data quality improvements may generate some value, more meaningful advances in data quality require a strategic and holistic approach to the problem.
An effective data quality improvement initiative also requires thorough documentation. Project leaders should establish a methodology for measuring data quality before and after the implementation of data quality improvement initiatives, including defining the desired end state and documenting the steps taken to effect positive changes in the organization’s data quality. This creates a clear agreement as to what to do, why it is important, and how to measure success.
Step 4: Monitor changes and plan further improvements
As noted earlier, the quest for data quality is a journey, not a destination. Organizations must view data quality management as an iterative process in which continuous improvement is the real goal. The previous three stages call for business leaders to define objectives and establish a means of measuring data quality both before and after the implementation of improvements. With the right data quality management software and a well-defined process for identifying and prioritizing key objectives, project leaders should be well prepared to measure data quality outcomes and assess the efficacy of their data quality improvement programs. That provides a starting point for a continuous improvement process, returning to step one of the framework to reassess the situation, establish objectives for the next iteration of improvement, and drive the organization toward a higher standard in data quality.
Precisely provides enterprise-grade data quality management software for some of the largest, most data-intensive organizations in the world. Read our eBook, Exceeding Expectations: Four Ways Data Quality Promotes Customer Loyalty, to learn how you can leverage data quality to facilitate and enhance opportunities to improve customer engagement and loyalty.