eBook
Exceeding Expectations: Four Ways Data Quality Promotes Customer Loyalty
This eBook looks at four ways to leverage data quality to facilitate and enhance opportunities to improve customer engagement and loyalty in support of B2Me personalization and digital transformation.
Introduction
Customer loyalty is at the heart of any business and its brand strategy. Positive customer experiences help retain customers, increase the chance that those customers will buy further products or services from the business, and improve the likelihood that those customers will recommend the business to others. Studies consistently show that the revenue generated by maintaining and enhancing customer loyalty and retention far exceeds that from new prospects.
Yet customer dynamics and expectations continually change at the same time that advanced analytics and machine learning are changing how organizations compete for customer attention and loyalty. Digital transformation is driving significant changes across industries from retail markets to the hospitality and transportation industries and even to financial services including banking and insurance. This transformation is even blurring traditional vertical boundaries where “up to 40% of revenue in the next decade will come from new services”1 that span across a business ecosystem.
A “B2Me” focus (beyond the traditional B2C or B2B view) that delivers individualized or “hyper-personalized” experiences through digital channels is central to this change as new brands disrupt traditional industries. Whether through social and digital outreach, dynamic real-time information, “B2Me brands are in the business of experiences versus just a product or solution.”2
Initiatives to support such transformation and individualized focus to gain and retain customer loyalty are only as effective as the data that underlies these efforts. To connect with customers and offer products and services of interest, businesses must know their customers including where and how to connect with them; how these customers have already engaged with the business; what these customers may be interested in; and how to continually improve the experience of these customers.
1 Atos Look Out 2020+, “Building the intelligent business platforms of tomorrow”, June 2018.
2 Chitra Iyer, “How Hyper-Personalization Takes CMOs from B2C to ‘B2Me’”, MarTech Advisor, May 22, 2018.
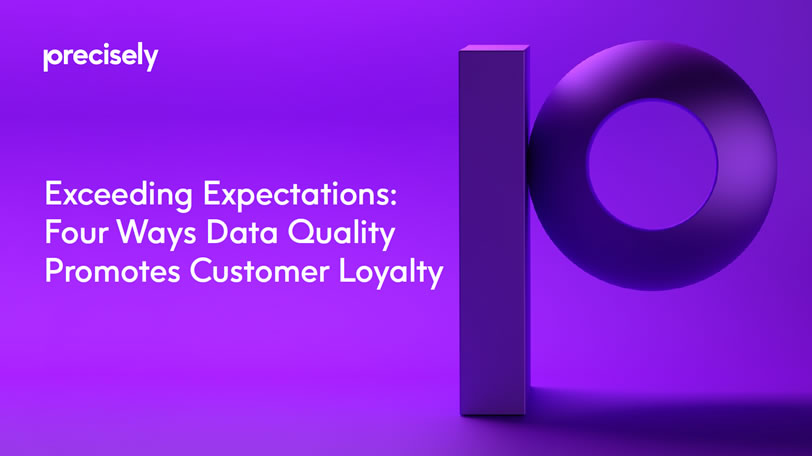
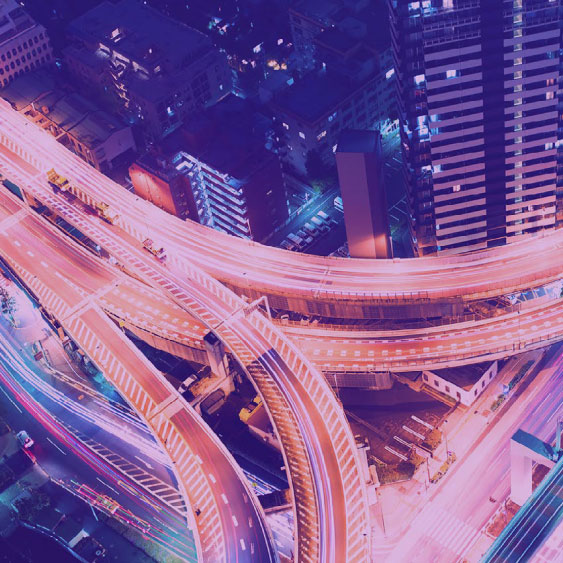
This eBook looks at four ways to leverage data quality to facilitate and enhance opportunities to improve customer engagement and loyalty in support of B2Me personalization and digital transformation.
We will explore the criticality of data quality techniques to:
- Capture accurate information in multiple channels upfront
- Connect the right data about the right customers together
- Enrich content downstream with timely and relevant insights
- Utilize the right sets of data to inform advanced analytics and machine learning
All of these are key to delivering experiences that delight the customer and retain their loyalty.
Customer experiences start upfront
Whether through websites, customer portals, customer care centers, or mobile apps, customer experience starts at these upfront touchpoints. Not every touchpoint will necessarily lead to information, but when a customer enters an account number, they expect the organization to know who they are and have the right information connected. Even without account numbers, many customers expect an Amazon-like experience where IP address or device ID provides a breadcrumb connecting to the customer and their prior experiences.
While not every business needs to know all details of every customer they engage (and many will want to keep their touch very light to provide the right customer interaction), there are both traditional pieces of data (name, address, email, phone) and additional data (account # if it exists, IP address, device geolocation, possibly device ID or browser) that organizations collect to handle many common transactions. Every piece of data that requires manual entry increases the possibility of downstream issues due to keystroke errors, misheard information, etc. The more data capture that can be automated, the higher the reliability and quality.
Capabilities such as type-ahead addressing minimizes data entry errors made by customers making online purchases, service representatives in the call center, and sales associates at the point-of-sale. Type-ahead addressing can also immediately tag geolocation data in or attach geolocation data to customer information.
LexisNexis, a leading global provider of legal, regulatory and business information and analytics, sees high quality address data as critical to all their customer interactions. By incorporating precision address validation, they get standardized physical addresses necessary not only for effective correspondence and delivery, but also to ensure that customers have the correct tax rates applied to their transactions.
For Iceland Frozen Foods, one of the top ten grocery chains in the UK, introducing their Bonus Card expanded the opportunities for upfront data collection. “Our strong data quality process means that at every Bonus Card swipe, we are capturing home delivery address, shopper and basket details immediately,” said the Business Systems Manager. “We are capturing data at the tills without slowing check-out times, whilst at the same time improving our customer intelligence base and the relevance and success of our direct marketing by personalizing offers to each individual’s profile.”
There is a balance needed here to ensure that the experience is not a negative one. By capturing data once with loyalty program information, Iceland Frozen Foods can provide a faster, value-add experience further along that also generates high quality data that contributes to better data analysis.
Single view of customer
Point of contact data can only go so far if the varied points do not connect. Customers are demanding that their likes and needs are understood – personalization of a kind that demands accurate, timely data and a single view of each individual customer.
Building an accurate, single view of customers (and potentially their households), their buying behaviors, channel preferences and purchasing history in indirect distribution channels is a complicated undertaking, particularly when operating globally. In part, this is due to key customer information often being limited, flawed, out-of-date, and held in different systems and formats. Where a business operates on a franchised basis, there is often a lack of common, consistent policies and practices to even enforce a level of uniformity. Consequently, putting data quality processes in place to standardize, cleanse and match incoming data becomes a critical part of the broad picture to support effective customer engagement and ensure customer interactions have all data at hand.
Canon Europe has about 500,000 records in their customer data hub but receives data from retailers and distributors from across Europe in multiple languages and formats with a varying degree of duplication from country to country. For some countries up to 60% of the original addresses are duplicates. In addition to historical information, Canon also receives data each week from its distributors via some 200 data feeds from 18 countries. Canon’s European Customer Intelligence Manager noted, “When our best customers buy tens of millions of euros of product per annum, we have to be able to trust what our systems are telling us when using that information to build and launch strategic customer management and distribution plans.”
For British Airways, carrying more than 33 million passengers a year, data is truly essential to the company’s competitiveness and it makes certain that its systems capture commercial data such as customer reservation and passenger information, effectively. Both marketing and customer relationship management processes require an accurate and timely single customer view as well as a household view of families and businesses to be effective and relevant. To achieve such a single customer view, not just at point of creation, but ongoing, requires blending historical customer data as a foundation and coupling that with live data feeds such as bookings and passenger lists with 100s of millions of records. Applying single, consistent rules and standards across all of these data sources is the only means to ensure high quality data that is standardized, cleansed, deduplicated and matched.
“Applying single, consistent rules and standards across all of these data sources is the only means to ensure high quality data that is standardized, cleansed, deduplicated and matched.”
British Airways’ Head of Business Intelligence indicated “Using Trillium DQ, we are building a data-quality firewall. This ensures only clean customer data resides in our data warehouse and reaches our business processes and systems around the world…. Trillium DQ is integral to the creation and accuracy of BA’s single customer view. It’s essential to customer service, marketing, retention and loyalty and to business decision making.”
The consistent holistic view of a given customer provided in a Single Customer View allows organizations to put the foundation in place to engage each and every customer with the right information at the right time.
Extending the view
Whether to extend understanding of the individual customer or to get predictive insights into customer behaviors, the traditional sets of data elements are often no longer enough. Consider the pace of change, even in the B2B sector: every hour there will be 211 businesses that move, 429 business telephone numbers that change, and 743 new businesses; every year 2% of all businesses change addresses and 5% of corporate phone numbers change.3
Enriching existing data content such as name, address, or phone number with additional elements provides data that facilitates this next level of analysis. Further, additional sets of associated data, particularly from outside third party or open data sources, add to what is available internally. For individual consumers this may be validation that phone or email information is real, or whether the individual has changed address. For business customers this may be insight into the corporate hierarchy, the industry, or even the location of the corporate headquarters so that diverse and disjointed information can be linked.
Geolocation data in the form of latitude/longitude coordinates is a typical starting point. This may simply be the coordinates of the given customer’s address but might also be coordinates for a device such as a mobile phone or an IP address. Addresses, postal codes, and geolocation may all connect to further demographic data, or these elements may highlight nearby points-of-interest. Even insight as to whether an address is for a residence or business helps an organization discern how and where its customers are interacting with them.
In the logistics industry, accuracy is everything. FedEx needed to identify customers reliably based on a wide variety of data elements, including business names, office suite numbers, and other address and location elements. Cost effective delivery requires them to identify and distinguish between companies on different floors of an office tower or divisions within a geographically dispersed corporate campus. Their systems must link customers based on detailed analyses of abbreviations, nicknames, synonyms, personal names, street addresses, and other location information. At the same time, speed to capture and apply this additional content is a mandate – and must be done at a global level. By ensuring complete, enriched data content, loyal customers would even forgo lower shipping rates from competitors, because they prized the ability to track their incoming and outgoing shipments so easily
Not all of this information needs to come upfront or be introduced into the Single Customer View. In fact, one of the strengths of the data lake is that analysts can continually bring in new, external content and assess its distinct value. If of sufficient quality or usability, that content can be standardized and matched to the Single Customer View to yield new evaluations and insights. In some cases, this will be experimental and adhoc with a goal of testing new hypotheses or evaluating machine learning algorithms. In other cases, these new sources become new ongoing data feeds, regularly checked for their own quality, and then applied to the Single Customer View to generate ongoing analytics and reports.
3 Dun & Bradstreet, “The 6th Annual Marketing Data Report”, 2018.
Generating downstream value
Improvements in direct customer interactions upfront and in holistic customer views both have tangible value in customer engagement and loyalty. Benefits continue downstream, too, as organizations are able to bring together more data for more refined insight and targeted, relevant customer experiences.
For Comic Relief, they were able to model the value they could derive from investing in a data quality solution. That value comes from two sides: reporting and analytics, and outbound marketing communications. “With reporting and analytics, if you don’t pull all of your interactions and transactions into a single view, you get two different views on one person. You might think they belong in segment A, but when you combine that data, they should really be in segment B. Our outbound marketing could be confusing for supporters, because they might be treated as if they are two different types of supporter,” per Comic Relief’s Head of Data. Tests on data revealed that 25% of people were getting the wrong customer journey or getting elements of two different customer journeys – not the desired experience! Implementing the Single Customer View unlocked a return on investment of between £600,000 and £800,000 per campaign from their supporters, not to mention internal productivity gains.
Shop Direct is the UK’s second largest pure-play digital retailer, with annual sales of nearly £2.0 billion. Their initiatives focused on two core value propositions: achieving a complete and accurate single- customer view to enhance its home shopping business; and secondarily reducing bad debt. “Trillium DQ has enabled us to better control debt risk and improve our debt collection costs. The ability to know which accounts belong to the same customer is worth more than £100,000 a year to us in reduced bad debt costs. This savings alone more than justifies our investment” stated the database development manager.
For British Airways, data quality applied to customer data throughout the system helps achieve multiple downstream goals. Business intelligence used for commercial planning and decision making is only as reliable as the data behind it. Demand forecasting, pricing and route and capacity planning can all be affected by any decrease in data quality. British Airway’s Head of Business Intelligence reflected that, “Our data analysts can deliver more accurate analyses more quickly and that supports faster and better strategic and operational decisions. More relevant marketing communications are helping optimize response rates, and by understanding our relationship with each customer we can improve customer service.”
The latest trend for downstream value is the adoption of AI and machine learning. Gartner indicates that a surge of activity in the retail sector is pending and that by 2021, more than 75% of retail organizations plan to deploy AI (and some 50% already have such in production or in pilots).4 The two great drivers that Gartner notes from their survey are cost savings (88% of respondents) and improved customer experience (75%). Recommendation engines, virtual assistants, and personalization of content are three top areas of consideration. All require an understanding of the individual, how they engage with the organization, and what their interests are.
4 Robert Hetu, “Survey Analysis: Retail Use of Artificial Intelligence Expected to Surge”, Gartner, 2019.
Summary
Studies consistently show that the revenue generated by maintaining and enhancing customer loyalty and retention far exceeds that from new prospects. Yet customer dynamics and expectations continually change at the same time that advanced analytics and machine learning are changing how organizations compete for customer attention and loyalty.
As we’ve discussed, data quality is central to this effort to enhance the customer experience and maintain and expand a loyal customer base. There are a number of upfront touch points where the customer controls the quality of the data he or she enters and organizations create consolidated Single Customer Views to ensure all customer information is effectively brought together. Then there is the enrichment of internal content and inclusion of external content. And finally, there are downstream applications, including analytics, AI, and machine learning, where inserting data quality throughout provides the accuracy and confidence to trust the data and deliver value that delights the customer.