eBook
Managing Risk & Compliance in the Age of Data Democratization
Data privacy and compliance, particularly around financial data, are key trends. Indeed, more governments across the globe are looking at ways to protect consumer and financial data, to ensure that information does not get into the wrong hands and is not used inappropriately.
Banks and financial services have two business imperatives: Comply with a growing list of regulatory measures aimed at preventing financial crime and pursue growth opportunities in a highly competitive market. With every interaction, these organizations strive to increase customer lifetime value by providing a more personalized customer experience while doing everything they can to make sure the data is used for its intended purpose.
This eBook describes a new approach to achieve the goal of making the data accessible within the organization while ensuring that proper governance is in place.
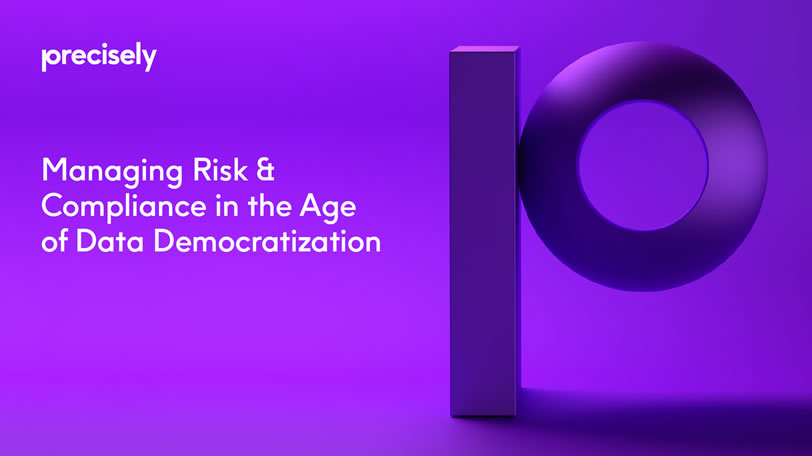
Two Sides of the Data Story
Because compliance and growth are often seen as competing imperatives, they represent two sides of the same data story based largely on how people typically work with the data.
Data producers:
Owners and operators of the systems where data is created within the organization. These systems may include CRMs, financial billing systems, IoT systems that feed into the organization from external sources such as social streams and devices. Data producers have curation, security, and ownership responsibilities for the various pieces of information that enable business operations.
Data consumers:
Business users who access the data generated by data producers. As owners of analytic projects, they need data for their analyses such as understanding relationships and patterns within the data. An example would be visualizing a graph of customer interactions to understand what customer journey drives additional business opportunities and value.
According to a recent Experian report describing the Top 10 Data Management Trends for 2020, 77% of organizations are actively working to put data insights into the hands of more people across the business. We have now entered the age of data democratization, which makes digital information accessible to the average non-technical user of information systems (data consumers), without requiring the involvement of IT (data producers).
Organizations are increasingly concerned with how data gets used, which works against the idea of democratizing the data. Having a proper data governance process, particularly one that is flexible, is crucial to the success of any kind of data democratization process.
A Project-Based Approach Falls Short
Organizations typically pursue initiatives such as analytics, business intelligence, compliance, customer experience, and digital transformation as one-off projects. For each project, teams map out a plan that includes data access, data integration, data quality, and data governance procedures. Ideally, these procedures inspire conversations about how to remediate the incomplete and inaccurate data that may require further review by data producers. This creates a situation where the people, process and technology are defined and used at the individual project level.
Such project-based approaches present challenges by creating environments where integration and governance processes become fragile. Oscillations between data consumer demand and the data itself, which is operated on by the data producers, causes a ripple effect.
The more involved the data sets and processes are, the more changes are required to manage overhead and ensure that each process runs correctly to deliver the right information. The additional complexity leads to more cost. Ironically, these types of issues challenge both the data producers and the data infrastructure, making it inflexible, especially when compared to the needs of the consumers on the front end.
The challenge is balancing the expansion of data use in the organization while working to reduce costs. Ideally, data consumers want to use the data to drive those front-end consumer processes. To do this successfully, however, requires that the data is fit for purpose.
There’s clearly tension between the ability to consume data and provisioning data in a scalable manner. To resolve this tension, companies must evaluate solutions that help reduce overhead and back-end complexity. Historically, this could be a data warehouse purpose-built to support business analytics; for more modern architectures it could be a data lake, a Master Data Management (MDM) solution, or a centralized repository where data producers offload the data for data consumers to access and work with it as needed.
The primary problem with a centralized approach is that the central repository seldom contains all the data across all the projects within the company. Alternatively, it’s not necessarily about one specific repository, but rather a structure of several repositories. In either case the data is never fit for purpose, because it was assembled by different people, using different processes and technology, working on different projects designed to fit a variety of needs.
A Modern Data Management Approach
Data consumers require swift insights to deliver quality customer experiences. For data consumers to drive better results, they really need to access all the data across every project. For analytics based initiatives in particular, data consumers must be able to drill down into data details. When they start looking for insights, data consumers don’t know exactly where their exploration will take them. Therefore, having free access to data is paramount to success.
From the data producer perspective, reducing complexity is about maintaining fewer mappings into the source data. What does that mean? Data producers must decide what bits of information they will make available, where that data exists, and define what processes and security are associated with it.
Here, they are not necessarily mapping to the end. Data consumer use cases often drive complexity on the data producer side as they drive to reveal the data the data consumer will use as required for their processes. Data producers choose what data to make available, who can access it, and what governance and processes to implement around it. This information needs to be available in a manner that allows each side to work with the data and be able to meet respective needs with greater simplicity.
Materially, what are the three parts of this equation?
1 – Simplicity:
Data producers have a targeted result that they strive for in terms of quality and structure. Data consumers know where to go to get access to data they need
2 – Scalability:
Reduce the number of redundant integrations between front and back end systems allowing both sides to work more effectively.
3 – Quality:
There are core data quality standards that should govern the definitions of most, if not all, data within an organization. However, data quality is often contextual, because it is based on business rules that vary from group to group within the business
How executive leadership looks at a list of customers may differ from how sales or marketing looks at a customer. As an example, when working with a large bank, is the customer the corporate entity or the local branch? Both could be correct depending on who is asking. By allowing data consumers more flexibility to define these rules on a project-by-project basis, data quality becomes more closely aligned to the use case. A related point is to enable the data consumer to consume data in a manner appropriate for the type of project at hand (and this could take more than one form).
You can have something that’s more like MDM or a Golden Record, just not necessarily from an operational case. This supports the ability to identify specific entities within the business, look at relationships between those business entities, and run analysis or other processes. For front end customer experience projects, this may also be around single view of the customer or Customer 360°.
You might approach a solution through a data warehouse, which is a more traditional approach that’s particularly well adapted for a traditional BI and reporting infrastructure. This works when the aggregates of data needed are already known. The dimensions of BI exploration fields and tools are already defined, as it’s usually a specific set of questions that need to be addressed. A warehouse is really an optimal opportunity for building these data aggregates up and feeding that out into the BI environment.
Another plausible solution could include a data lake fed by almost all transactional or detailed data into an environment based on Hadoop where an analyst can quickly run, analyze, and process data within that specific environment. A data lake approach usually has a corresponding federated view layer in combination with the data lake, the MDM, or the data warehouse. The federated view enables access to the front end and backend systems to answer additional questions that might come up as the project progresses, without overly taxing either the integration or data quality processes.
These structures trigger the need to organize and build a business glossary to help data consumers understand the data present within these environments, how it was curated and how it’s organized in terms of the business entities and the models available for use.
On the producer side, it becomes an exercise of mapping into the specific structures that align to critical project needs, integrating with the MDM environment, running data quality processes against it and promoting through the data federation environment– all while establishing appropriate security and access controls. This provides the data consumers with confident data which is what they need to support their modeling and analysis exercises.
Organizations Need a Transfer Point
Data Democratization Enables Better Customer Experiences
Most of these core processes have been around for a while. What’s driving this deeper need is the emphasis on customer experience.
Customer experience involves delivering the end consumer a sense that you not only understand their needs, but that you also understand how and why they want to interact with your business; knowledge that is honed through analytics and data.
Many of our clients are dealing with omnichannel approaches, whether web to store, storefront service through the web, or service through other mediums like a call center. These factors together encourage organizations to consolidate the complexity of channel into a single cohesive experience for their customers. This triggers internal focus and attention around understanding who each individual customer is, what their optimal customer journey should be, and how to improve their experiences throughout their journey.
Accomplishing these goals requires an approach that facilitates the access and synthesis of customer information and interactions from all channels to build a single view of each customer across the entire business. The use of analytics is a core differentiator within organizations as they compete in the marketplace. Interestingly, analytical tools haven’t changed much in terms of the algorithms used or the way people perform the analysis. The real evolution in recent years is the increased access to data and the requirements of data quality and trust.
Throughout this ebook, our objective has been to describe a new approach that achieves the goal of making data accessible within the organization while ensuring that proper governance is in place to comply with an increasing number of regulations. We outlined the two sides of the data story based on perspectives of data producers and data consumers and their needs. We discussed the challenges with the current approaches today and outlined the components of a new solution—simplicity, scalability, and quality. At Precisely, our modular and unified solution is built on proven technology used by more than 12,000 customers worldwide.