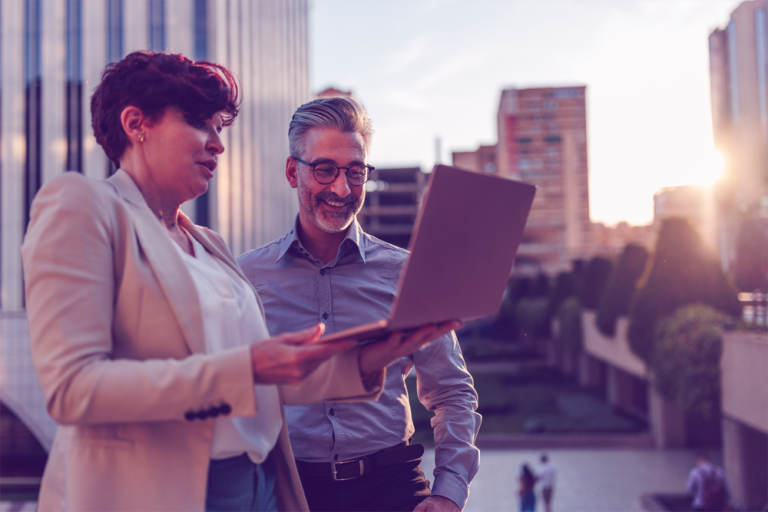
What Is Data Observability and Why You Need It?
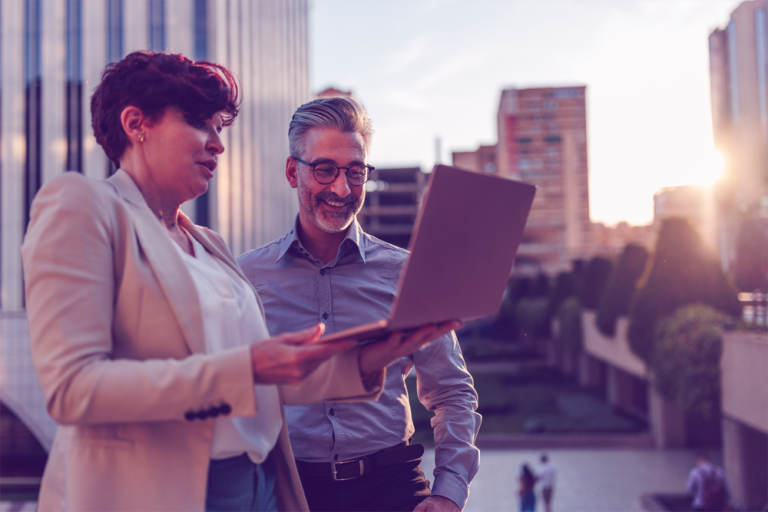
Experts estimate that the world is generating 2.5 quintillion exabytes of data every day. That information resides in multiple systems, including legacy on-premises systems, cloud applications, and hybrid environments. It includes streaming data from smart devices and IoT sensors, mobile trace data, and more. Data is the fuel that feeds digital transformation. But with all that data, there are new challenges that may prompt you to rethink your data observability strategy.
The most recent Precisely Data Trends Survey found that over two-thirds of organizations experience negative effects due to disparate data. According to the Harvard Business Review, nearly half of newly created data records contain at least one critical error. It’s no wonder that 84% of CEOs doubt the integrity of the data on which they base their decisions.
Systems and data sources are more interconnected than ever before. The resulting interdependency often leads to new problems. Complexity leads to risk. A seemingly simple change can have devastating downstream ramifications. A broken data pipeline might bring operational systems to a halt, or it could cause executive dashboards to fail, reporting inaccurate KPIs to top management.
For some time now, data observability has been an important factor in software engineering, but its application within the realm of data stewardship is a relatively new phenomenon. Data observability is a foundational element of data operations (DataOps).
Briefly stated, data observability is about monitoring and attending to the quality and availability of enterprise data as it is moving. Observability extends beyond mere monitoring insofar as it provides deeper insights into what is happening with the data and why. Observability includes alerts, but it also incorporates powerful real-time machine-learning intelligence that detects outliers and anomalies in your data as it moves throughout your enterprise.
For over 100 years, observability has been a key element of numerous process methodologies, especially in the manufacturing industry and software development. The application of this concept to data is relatively new. It ensures the reliability of your processes and analytics by alerting you to potentially problematic events as soon as they occur. This enables the user to visualize data processes and quickly identify deviations from typical patterns. The best data observability tools incorporate AI to identify and prioritize potential issues.
At its core, data observability can be broken down into three primary components:
- Discovery, profiling, and monitoring: Collecting information about where data is located, what it contains, and who uses it, and then monitoring that data proactively and continuously.
- Analysis: Processing the information about your enterprise data, assessing historical trends, and detecting outliers – all with the use of AI/ML for automated intelligent analysis.
- Visualization and alerting: Providing key users with dashboards to visualize real-time data activity, sending proactive alerts to notify users of outliers, and providing additional context that informs them whether the data is ready to be used for decision-making.
Analyst Report
TDWI Checklist Report: Succeeding with Data Observability
Succeeding in modern business requires comprehensive visibility into your data’s quality and into the health of your enterprise’s processes for ingesting, transforming, cleansing, and delivering it into production applications. Learn more here.
Many experts also find it useful to describe data observability in terms of the key attributes that it monitors:
- Distribution tests to determine whether values fall within a normal or acceptable range.
- Volume watches for unexpected numbers of new records. Several years ago, for example, Amazon reportedly received hundreds of unintentional orders when a local news anchor repeated the phrase “Alexa, order me a dollhouse” on-air, and viewers’ at-home devices dutifully responded to the request. By monitoring for unexpectedly high volumes of orders, companies can spot these issues early and address them proactively.
- Schema refers to the way data is organized or defined within a database. If a new column is added to a table within your customer database, for example, it can have powerful implications for the overall health of your data. Records that pre-dated the change may contain a null value for the new field or may be set up with a default value. In either case, the change can affect analytics. Many customers have a data catalog solution today that documents the schema, and this should be leveraged as part of a data observability solution.
Data observability identifies potential data issues early, enabling users to proactively solve problems at their root. That prevents further issues from occurring and eliminates the need to go back and fix data quality problems after the fact. Old-school methods of managing data quality are no longer sufficient. Manually finding and fixing problems is too time-consuming, given the volume of data organizations must deal with today. Data observability helps you manage data quality at scale.
Data Observability vs. Data Quality
It’s important to understand that data observability with data quality are a part of a complete data quality program. Data quality tends to focus on clearly defined business rules, analyzing individual records and data sets to determine whether or not they conform to the rules. Customer records, for example, should be consistent across the various systems and databases that include customer information. Furthermore, customer addresses must be valid and complete. If the city name is missing, or if the address includes a non-existent postal code, then it does not conform to business rules. Data observability, in contrast, focuses on anomaly detection. If the volume of data changes suddenly and unexpectedly, for example, it’s important to know that, as well as to understand why it’s happening. A sudden spike in certain values could likewise indicate an upstream issue with the data. Longer-term trends in the data often merit attention as well.
Data observability helps to answer the question: “Is my data ready to be used?” That might have different meanings to different users. For operations managers who rely on downstream analytics to drive key decisions, it means having confidence in the information they need to do their jobs effectively and efficiently. For a data scientist building machine learning models for an important AI initiative, data observability helps set the stage for long-term success. For a top executive who wants that big-picture view of how the company is performing, it means knowing you can trust the data.
Imagine that your development team is making some changes to one of your core operational systems. They change the data type for several key columns in a table that holds customer order information. Unbeknownst to them, that information feeds into a self-service portal that allows customers to inquire about order status. Because of the upstream change, the downstream application might no longer work. A data observability tool would identify this change and alert the users to take action.
Next, imagine there is a sudden and unexpected drop-off in orders from your UK division. A data observability tool identifies this anomaly and alerts key users to investigate. The root cause turns out to be a problem with the data pipeline feeding UK orders into the main system. By resolving the problem quickly, the team can ensure the timely processing of those sales orders.
Data Observability versus Data Monitoring
Initially, it may be tempting to think of data observability as a kind of monitoring system that simply watches for anomalies. Observability extends further than that, providing insights that help data stewards assess the overall health of their enterprise data.
Consider the following medical analogy. When the nursing team at a hospital records their patient’s vital signs every few hours, they are recording some basic (but important) facts about that person’s health status. That is akin to monitoring – watching for any indications that something might be wrong.
When the same patient is connected to diagnostic tools that collect data continuously, in contrast, their medical team has access to a constant stream of data that provides insights to help understand any problem that occurs and determine the correct course of action. Visual analytics and other tools offer deeper insights into the patient’s health and provide clues as to what may be happening.
The best data observability tools use advanced technology to apply machine learning intelligence, watching for patterns in enterprise data and alerting data stewards whenever anomalies crop up. That enables business users to proactively address problems and potential problems as they happen. The end result is healthier data pipelines, more productive teams, and happier customers.
Why Is Data Observability So Important?
Trust in data is vital for today’s enterprises, which are using analytics to identify strategic opportunities, as well as to support line-of-business users in making tactical decisions and feeding AI/ML models that automate routine tasks. Data observability plays a powerful role in ensuring that data is trustworthy and reliable, providing these key benefits:
- Ensure trustworthy data for accurate reporting and analytics. By detecting anomalies and automatically alerting the appropriate users to possible problems, data observability empowers organizations to be proactive rather than reactive, addressing data issues that have the potential to disrupt the business and create costly downstream problems.
- Reduce costs and time to resolution for operational issues. Data observability provides vitally important information that helps users quickly determine the root cause of an issue. That means solving problems before they can do significant damage.
- Reduce risk, supporting successful transformation initiatives. Digital transformation is a top priority for many businesses, but it inevitably involves more data and more rapid change than ever before. Data observability empowers data engineers and other users with a critical understanding of what’s happening to your data.
Time for a New Data Observability Strategy
Data observability helps organizations understand the overall health of their data, reduce the risks associated with erroneous analytics, and proactively solve problems by addressing their root causes.
To get the most possible value from a data observability solution, look for a technology solution that includes an integrated data catalog. That provides a single searchable inventory of data assets and allows technical users to easily search, explore, and understand their data. It enables key users to visualize the relationships among various data sets and clearly understand data lineage. An integrated data catalog also provides collaboration tools such as commenting capabilities. It enables auditing, certifying, and tracking data across its entire lifecycle. To learn more about developing a data observability strategy for your business, start by downloading the TDWI Checklist Report: Succeeding with Data Observability.
At Precisely, we offer a suite of technology solutions that work together seamlessly to ensure that your data provides a complete, accurate, timely, and contextual view of reality. Data Observability is just one of the seven services in the powerful Precisely Data Integrity Suite – an integrated, interoperable suite designed to deliver accurate, consistent, contextual data to your business – wherever and whenever it’s needed.
To learn more as to why organizations should make data observability part of their data management practice, read this TDWI Checklist for Data Observability Report.