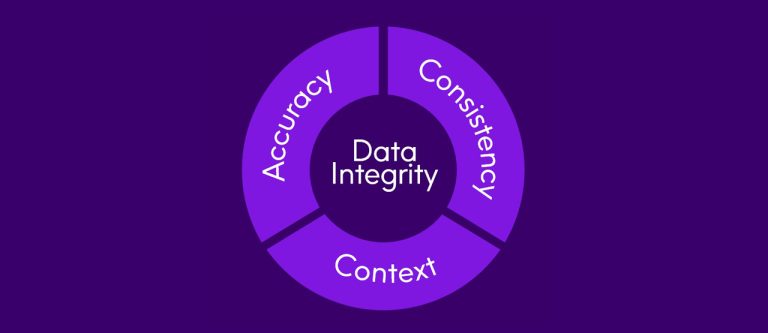
What is Data Integrity?
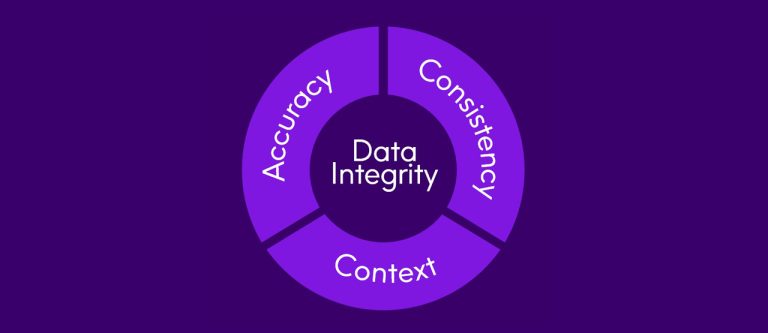
Confidence is everything. In successful businesses, it comes from the ability to make clear, timely decisions based on trusted data. But data is complicated, and 84% of CEOs are concerned about the integrity of the data on which they base decisions. This is despite significant investments their businesses have made in managing data more effectively.
When data is difficult to find, flawed, or misunderstood, it disrupts business. Decisions are made on instinct alone or not at all, destroying value. That’s why businesses need data integrity. But what exactly is it? Many proposed definitions focus on data quality or its technical aspects. But organizations must approach data integrity from a broader perspective.
What Data Integrity Is
Data with integrity is trusted because it has maximum accuracy, consistency, and context. It is available whenever and wherever it’s needed, empowering you to make fast, confident decisions that can help you add, grow, and retain customers, move quickly and reduce costs, and manage risk and compliance.
Accuracy and consistency are key data quality characteristics, but data integrity is also about data having rich context. In the case of customer data, for example, it means understanding what drives purchasing behavior. It’s about understanding demographics, lifestyle, and critical events impacting consumer needs. It’s also about location, knowing where your customers live, work, and play.
Data integrity also requires the entire tapestry of data sources throughout an organization to be woven together so that business users can develop a complete and meaningful picture of the things that matter most to them. That requires data integration to unlock the information stored in siloed systems.
Data quality issues often present a significant challenge to data integrity. Inaccurate, non-standardized, and incomplete data diminishes the potential of business analytics, artificial intelligence, and machine learning, even in a best-case scenario. In the worst case, it renders results invalid. A sound data integrity strategy includes data quality solutions capable of:
- Standardizing and validating data
- Identifying gaps or discrepancies
- Data observability capabilities to uncover data anomalies and trigger workflows and processes to correct those errors at scale
Finally, data integrity requires a practical framework for data governance to oversee all other aspects of integrity and ensure the organization complies with best practices for security and privacy and all necessary regulations.
Why Does Data Integrity Matter for AI Success?
What does AI-ready data look like? It needs to have data integrity – that’s maximum accuracy, consistency, and context. Think of it this way: without trusted data, there’s no trusted AI. Trusted data needs data integrity.
Without accurate, consistent, and contextualized data, even the most advanced AI systems can falter, leading to biased outputs, untrustworthy results, lack of contextual relevance, and, ultimately, a loss of trust in your AI. Whatever your use cases, you can’t take these risks lightly. Your investments will only pay off if your AI systems are built on a foundation of trusted data.
It’s worth noting, though, that many organizations face data integrity challenges, like:
- struggling to integrate data fast enough
- understanding and governing responsible use of data
- observing and improving data quality
- enriching with third-party data and spatial insights for deeper context
- guaranteeing security and privacy
Do any (or all) of these challenges ring true for you? You’re not alone. At the 2023 Gartner IT Symposium Research Super Focus Group, only 4% say their data is AI-ready.
What’s the Difference between Data Integrity and Data Quality?
Although many people confuse data integrity with data quality, often using the two terms interchangeably, there are some important differences between data integrity and data quality.
Data quality refers to the reliability of data. It is an essential subset of data integrity. When data has high quality, it is complete (not missing essential elements), unique (free of redundant or extraneous entries), valid (conforming to the syntax and structure defined by the business requirements), timely ( sufficiently up-to-date for its intended use), and consistent (represented in a standard way throughout the dataset).
Data integrity goes beyond data quality, requiring that data be complete, accurate, consistent, and in context. Data integrity is what makes the data actually useful to its owner. Data integrity is based on six main pillars:
-
-
- Data integration: Regardless of its original source, on legacy systems, relational databases, or cloud data warehouses, data must be seamlessly integrated to gain visibility into all your data in a timely fashion.
- Data observability: Prevent business disruption and costly downstream data and analytics issues using intelligent technology that proactively alerts you to data anomalies and outliers.
- Data quality: Data must be complete, unique, valid, timely, and consistent to be useful for decision-making.
- Data governance: Manage data policy and processes with greater insight into your data’s meaning, lineage, and impact.
- Location intelligence: Make data more actionable by adding a layer of richness and complexity to it with location insight and analytics.
- Data enrichment: Add context, nuance, and meaning to internal data by enriching it with data from external sources. Adding business, consumer, or location information gives you a more complete and contextualized view of your data for more powerful analysis.
-
2025 Outlook: Essential Data Integrity Insights
What’s trending in trusted data and AI readiness for 2025? The results are in!
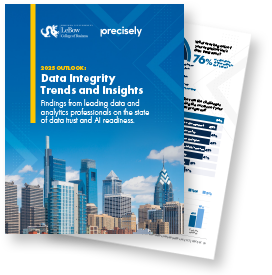
The Journey to Data Integrity Begins with Business Value
Data integrity is not a binary all-or-nothing proposition, and it’s a journey that will look very different from one organization to another. There is no one-size-fits-all approach. The data integrity journey often begins with initiatives around specific projects, where the impact of data integrity efforts is readily visible, and its results deliver business value across many different teams across the organization.
For example, improving the customer experience might begin with breaking down the silos between mainframe systems, digital marketing automation, and CRM, and ensuring that all systems using data are kept current with changes to transactional systems. That effort may reveal data quality issues that must be addressed, correcting system discrepancies, identifying anomalies, and proactively managing quality going forward. Finally, the project team may identify a need for external data sets to enrich the company’s internal customer data with demographic, lifestyle, and geospatial information — all of which provide essential context.
A data integrity initiative may begin with the need to establish policies for safeguarding customer information, controlling unauthorized access to data, and documenting compliance with all relevant privacy and data sovereignty regulations. The solution, data governance, will also enable you to answer essential questions about your data usage, impact, and lineage. A data governance initiative may lead you to identify and address data quality issues — and so the data integrity journey continues, increasing confidence in data across your organization and producing more accurate, informed decisions and reporting.
Starting Your Data Integrity Journey with the Right Tools
The Precisely Data Integrity Suite delivers value at every step along the data integrity journey, regardless of how your company may choose to approach it. The Precisely Data Integrity Suite enables your business to build trust in its data through market-leading data integration, data observability, data governance, data quality, geo addressing, spatial analytics, and data enrichment capabilities. These core capabilities deliver value at each step on your journey to data integrity – data that is accurate, consistent, and filled with context.
In a recent IDC survey of 310 business and data analysts, nearly half of the respondents indicated a general lack of trust in data quality, and 56% indicated a lack of trust in the results of data analysis. This lack of trust is not the source of the problem – it is a symptom of the data. Data integrity provides a firm foundation for data analytics and confident actions. Accuracy and consistency in data, enhanced with context through location and enrichment, can help companies achieve data integrity
Precisely partnered with Drexel University’s LeBow College of Business to survey more than 450 data and analytics professionals worldwide about the state of their data programs. Now, we’re sharing the ground-breaking results in the 2023 Data Integrity Trends and Insights Report. Get your copy today.
Frequently Asked Questions:
What are the main factors that cause organizations to lack data integrity?
Most organizations struggle with data quality. According to the 2023 Data Integrity Trends and Insights Report, 70% of companies that struggle to trust their data cite data quality as their biggest issue. Yet most organizations are grappling with broader issues, including the lack of contextual richness in their data. Location intelligence and data enrichment, for example, provide the raw materials for far richer and more valuable data-driven insights.
How does data integrity impact business outcomes?
Data with integrity is trusted because it provides maximum accuracy, consistency, and context. It is available whenever and wherever it’s needed, empowering organizations to make fast, confident decisions; add, grow, and retain customers; move quickly; reduce costs; and manage risk and compliance.
What’s the best approach for businesses looking to improve their data integrity?
Every company’s path to data integrity is unique, but most begin by focusing on scalable, repeatable processes for developing and maintaining quality data. That requires enterprise-grade tools that apply business rules to define and enforce data quality, route potential problems to the individuals best suited to address them, and monitor KPIs for the organization.
What tools does Precisely offer to help organizations achieve and maintain data integrity?
The Precisely Data Integrity Suite is an innovative, modular, interoperable, and scalable solution. It enables each organization to address its own unique requirements with a holistic set of tools that work seamlessly together. The Suite comprises seven interoperable cloud services: Data Integration, Data Observability, Data Governance, Data Quality, Geo Addressing, Spatial Analytics, and Data Enrichment. Together, these seven services form one powerful data integrity foundation that accelerates your data integrity journey and provides the confidence you need for fast decision-making.