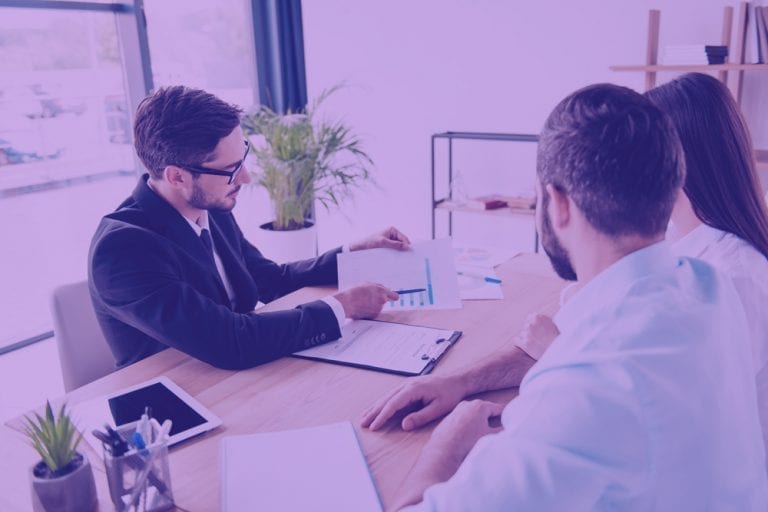
Exploring the Role of Data in the Mortgage Industry
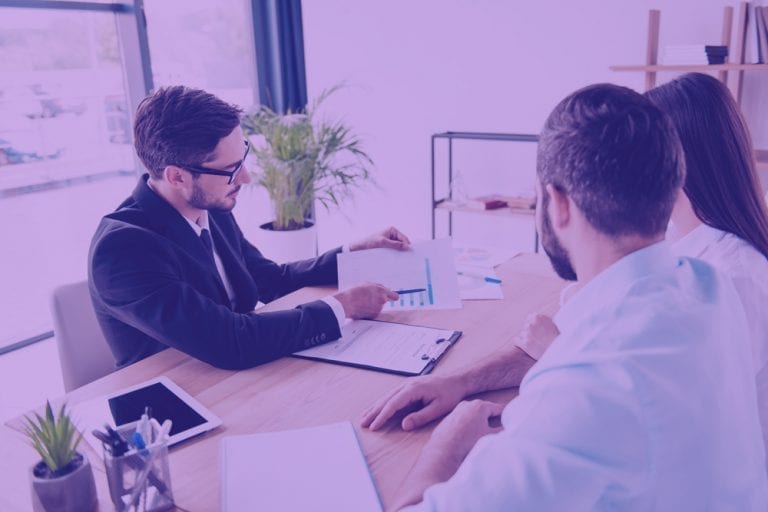
Big data and its corollaries – artificial intelligence (AI) and machine learning – are having a transformative effect on virtually every industry. As the volume of data collected by businesses, governments, and NGOs increases at a faster and faster rate, the accuracy of predictive insights produced by that data increases.
A decade or two ago, many companies were focused on gaining a better understanding of the data residing within their own information systems. Organizations with an especially large volume of data might consider running large batch ETL (extract, transform, and load) jobs to aggregate and normalize information in a data warehouse, where it could be processed and analyzed more easily.
As ambitious as many of those projects were, they seem antiquated by today’s standards. Big data repositories have evolved to handle higher volumes of data, and as processing speeds and interconnectivity have continued to grow exponentially, there has been a natural shift toward greater aggregation and data enrichment. External sources for rich, high-quality data are enabling organizations to unlock vast new potential.
The mortgage industry is no exception. Consider a few use cases.
Predicting creditworthiness with data enrichment
There are plenty of opportunities to leverage big data within the mortgage industry. Many industry insiders have noted, for example, that assessing creditworthiness is more complicated than it once was. Millennials are less likely to have a well-established credit history because they are less likely to have credit cards or car loans. In the new “gig economy”, many are self-employed as well.
Recent increases in non-qualified mortgages (“non-QM” mortgages) attest to this fact. According to Moody’s, residential mortgage-backed security issuances for non-QM loans have increased from $570 million in 2016 to around $23 billion in 2019. Lacking a traditional credit history for many non-QM borrowers, mortgage companies are looking to alternative sources to assess creditworthiness. Big data and predictive analytics help to fill that gap.
Using big data, mortgage companies can also model loan classifications according to an ever richer array of data points. This can help lenders to better understand which loan applications require a closer review.
Unlocking the power of location intelligence
Many underwriters have turned to automated valuation models (AVMs) to gain a better understanding of property values and risk. Some of the traditional measures of property value, such as previous sale prices for property, are readily available. Comparable nearby properties are also a key element of traditional approaches to valuation, but sophisticated lenders are turning to data enrichment and leveraging third-party data to provide an enriched view of the many factors that affect a property’s value.
A simplistic view of comparables might suggest that a similar-sized house within a half-mile of a particular property should give a reasonable indication of its value. Distance alone is not always a good indicator, though. By understanding the boundaries surrounding a property and by understanding the boundaries surrounding potential comparable properties, underwriters can get a better view of whether they are really comparing apples to apples.
Let’s say, for example, that our target property is in a highly desirable subdivision, with access to a golf course and swimming pool. The proposed comparable property is nearby but sits outside of the subdivision boundaries. This gives us a much clearer (and therefore more accurate) picture of the situation, but unless we understand the boundary lines (in this case, the subdivision boundaries) that encompass that property, we will have a limited view from which to assess that property’s value.
eBook
Location Intelligence for the Cloud
Location-based data and software are helping companies improve performance exponentially. Download this eBook to learn how to expand your opportunities with location intelligence.
Now let’s take the same situation, but instead of looking at subdivision boundaries, we decide to look at school district boundaries. If those two houses are in different school districts, and if one of those school districts is significantly better than the other, that will affect property values as well.
In addition to local boundary data, lenders are also looking to data enrichment by joining additional datasets with a property record, such as demographics, points of interest, or type of dwelling. By integrating this geo-enriched data into machine learning processes, the most important attributes that help predict property values become more evident and lenders are able to identify which properties can be prequalified for a loan and which properties would not be a good business investment.
The more data we have, including traditional price information, various boundary definitions (such as the two examples given), crime rates, and proximity to services, the better we are able to determine an accurate value of a property. Location intelligence unlocks a wealth of new information that has historically not been part of the valuation process. Precisely’s extensive data portfolio provides the information fueling this location intelligence, related to points of interest, demographics, boundaries, streets, and addresses. These datasets reveal deeper insights for more accurate valuation.
Increasing customer retention with data enrichment
Data analytics gives lenders a better understanding of their customers as well. Many lenders are using AI and machine learning to assess the likelihood that certain customers may be about to refinance, for example. It’s almost always easier to sell to an existing customer than it is to acquire a new one. If a customer is considering refinancing, and their current mortgage lender can reach out to them preemptively with an offer, it can result in a win for both parties.
By incorporating external data sources with internal customer information, lenders can better identify services that may be of interest to a customer. Predictive analytics can provide a better understanding of which customers represent a default risk and which ones may be in the market for additional mortgage services.
By understanding purchasing behavior, social media profiles, employment history, and other details about their customers, mortgage lenders can increase customer retention and reduce losses. By enriching existing customer data with external sources, lenders are able to see a more complete view of the customer, without making any additional demands on the customer’s time.
Fraud detection
Lenders are using AI and machine learning to improve fraud detection, compliance reporting, and risk management. Analytics can be used to flag outlying data points for further investigation and follow-up. This kind of targeted management-by-exception approach saves time by enabling skilled staff to focus on cases that are most likely to present an actual risk, rather than wasting time on randomized checks.
AI and machine learning are especially well-suited to pattern recognition. Over time, as a greater volume of historical data becomes available, those capabilities will grow even greater.
These are just a few of the long list of use cases for big data and predictive analytics in the mortgage industry. As the volume and variety of information continues to increase and as the trend toward aggregating and data enrichment accelerates, the mortgage industry is likely to realize even greater benefits from effectively organizing, managing, and leveraging location data.
Download our eBook Location Intelligence for the Cloud to learn more.