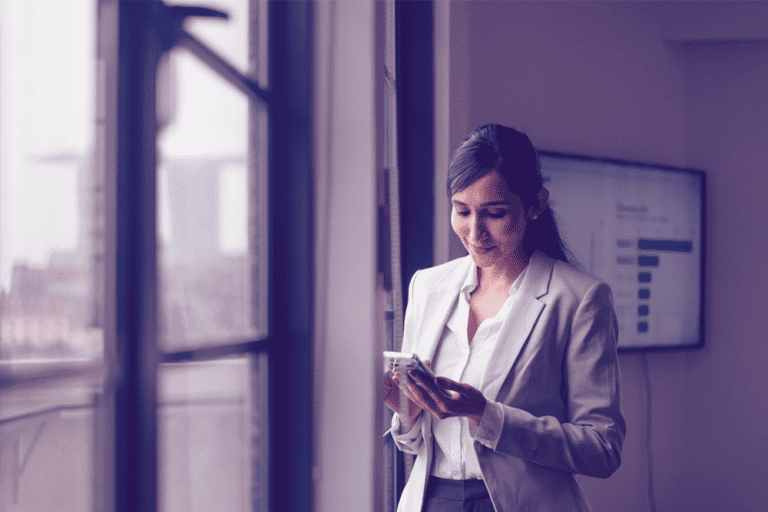
The Intersection of Data Analytics and Data Governance
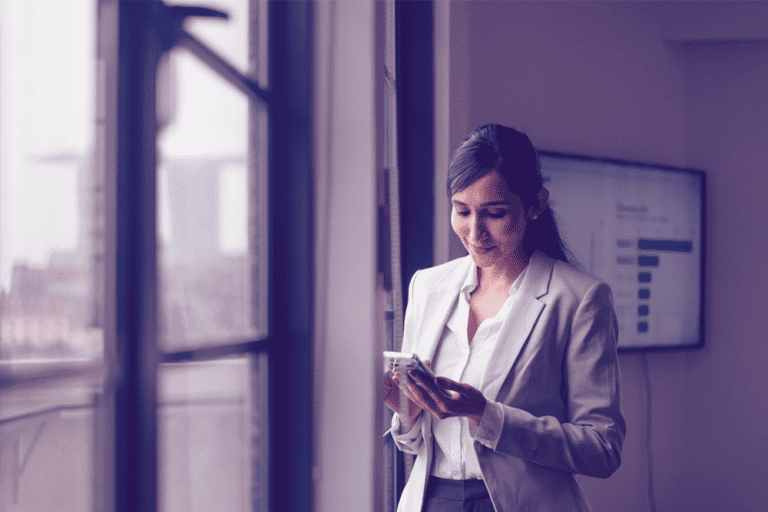
Are you suffering from data exhaustion? The symptoms can vary widely, but it generally stems from the unrelenting influx of data and your organization’s continued struggle to define a full-scale strategy to organize, understand and leverage that data for maximum advantage. There’s certainly no shortage of treatment options being marketed; whatever analytics and data management obstacles you’re facing, there’s literally scores of solutions available to overcome them and ease your pain.
You’re not alone in this affliction, and it is entirely understandable. The fact is, the era of big data arrived in fits and starts for most companies, with many organizations forced to take a reactionary approach to data management. As the value and volume of data grew, projects arose and fires erupted, and tools to address a specific need would be purchased or built as the constraints of both budget and resources allowed. Today, this has left some organizations with fatigue and frustration from the patchwork of tools that loosely comprise their data management strategy.
Such an approach was borne out of necessity, but unfortunately it isn’t strategic, it isn’t scalable, and it won’t serve as a long-term solution for managing data and maximizing data value for increased profitability and competitive advantage. It’s time for organizations to consolidate their data management strategies, and find ways to increase efficiencies and synergies among tools and solutions. Identifying symbiotic relationships is a good first step towards developing an integrated data management plan to serve both immediate needs and future goals.
Finding data management symbiosis
Symbiotic relationships generally occur in nature, and interactions such as mutualism represent species who work together for reciprocal benefit. Yet this concept can also be applied to an organization’s data ecosystem—where seemingly disconnected or disparate data tools can serve a shared purpose. A good example of this is the connection between data analytics and data governance. Data governance is increasingly recognized as a foundational component of any strong data management plan, and analytics can improve the performance and efficiency of an organization’s governance efforts. But organizations don’t accrue data for data’s sake; the end goal of raw data is the insights it can reveal and the improved decision-making and outcomes that can result. Analytics are the critical enabler of this process, and data governance also plays an important role in helping organizations maximize the value of their analytics and data assets.
Analytics-enabled data governance
Data governance is the formal orchestration of people, processes, and technology to enable an organization to leverage their data as an enterprise asset. It serves a critical function in business to support regulatory compliance, but it is also crucial to ensuring a common understanding of organizational data assets across an enterprise. Key components include business glossary, data dictionaries, data lineage, and metadata management. These inform users about the source, use, relationships, and definitions related to data—including business terms, attributes, and dependencies. It also assigns data owners and stewards to data assets to increase accountability and enable access to data resources, encouraging data usage. And it can have a direct impact on data quality by providing monitoring, quality dimensions, and scores.
With analytics-enabled data governance, machine learning algorithms can monitor and improve data quality across an enterprise, self-learning as issues are resolved. Improved data quality increases user trust in data reliability, and therefore increases data utilization for analysis. Machine learning can also play a vital role in compliance efforts, with automatic monitoring for potential non-compliance. Without analytics, governance programs simply confirm compliance with regulatory requirements. With analytics and active monitoring, organizations can proactively identify areas where they may have violations—a capability that will only grow more important as regulations like the General Data Protection Regulation (GDPR) go into effect.
Read our eBook
Maximizing Analytics ROI
For more information on the integration of analytics and data management to build a comprehensive data governance solution, read our eBook.
Enhanced analytics through data governance
Analytics can clearly improve organizational data governance efforts, but equally apparent is the impact that data governance can have on an organization’s analytics efforts. Data governance is all about increasing data understanding across a business enterprise, and encouraging collaboration to get the most from your data assets. It shouldn’t reside in IT as a technical understanding it needs to be business focused to drive data utilization. Engaged users who trust and understand data are far more likely to then use analytics to look for intelligence and insight. Strong data governance creates educated users who can rely on data assets and will frequently turn to analytics to help solve business issues and uncover unseen issues and opportunities.
Elements of governance also make analytics possible. Metadata management is oversight of “data about data,” and was previously mentioned as an important aspect of a comprehensive data governance approach. But it is also the key to true predictive analytics for deriving valuable business insights, and predictive analytics are in turn the key to gaining competitive advantage and insight into future outcomes and events.
Self-service is essential
The mutually beneficial relationship of data governance and analytics should be clear, but it must be said that if an organization delegates all responsibility for analytics to IT or an elite group of data scientists, it is largely a moot point. Data management and data governance today should be focused on empowering business users with a self-service approach to accessing and leveraging data assets as they’re the power users looking for to use the data. IT resources are scarce and overburdened, and their time can be better spent than juggling a constant stream of competing requests from myriad business users. Likewise, business users can’t afford to wait weeks or even months for a data request to be processed; by then, the issue may be irrelevant, or the data may be out-of-date.
As organizations re-examine their data strategies, they need to give users access to self-service analytics that will allow them to routinely rely on analytics, increase their speed to insights, and put them in the driver’s seat as they look for innovative ways to gain competitive advantage and improve the bottom line.
For more information on the integration of analytics and data management to build a comprehensive data governance solution, read our eBook Maximizing Analytics ROI.