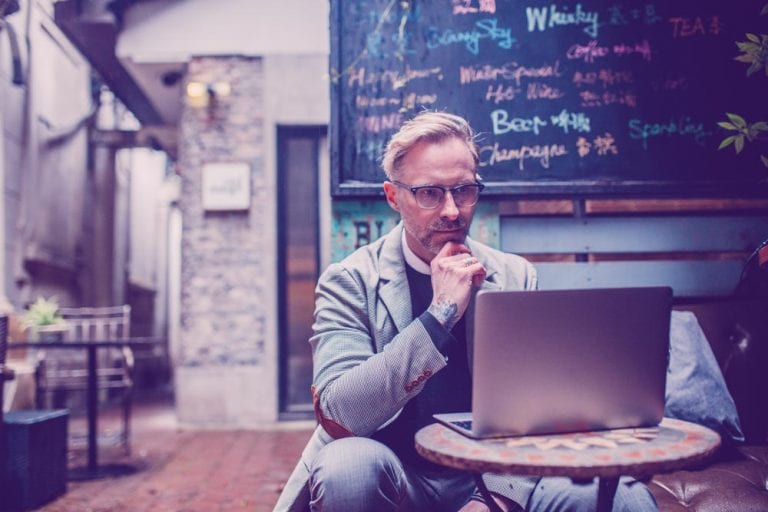
Data Quality Dimensions: How Do You Measure Up? (+ Downloadable Scorecard)
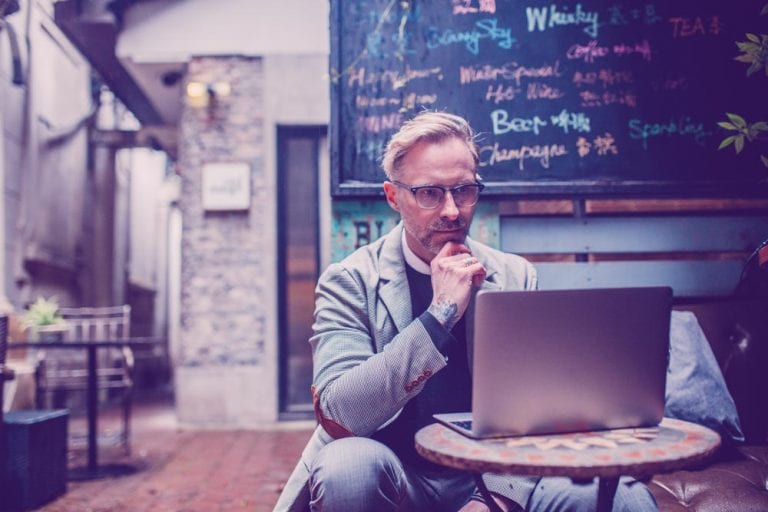
Virtually every business leader understands just how valuable data can be for driving innovation, increasing revenue, improving customer satisfaction, optimizing processes, and achieving compliance. A recent study from 451 Research found that almost 80% of business leaders say that data is becoming more important for effective strategic decision-making. That’s why they’re implementing tools and processes that support data-driven decisions in their organizations, improving outcomes and accelerating the pace of business.
Naturally, there’s an important caveat. Data can only deliver business value if it has high levels of data integrity. That starts with good data quality, contextual richness, integration, and sound data governance tools and processes. This article focuses primarily on data quality.
Data quality is often the starting point for organizations seeking to improve overall data integrity. In a recent study by Drexel University’s LeBow College of Business, 70% of respondents who struggle to trust their data say that data quality is their number-one problem.
How can you assess your data quality? Data quality is measured along six dimensions:
- Accuracy
- Completeness
- Consistency
- Timeliness
- Validity
- Uniqueness
Six Data Quality Dimensions at a Glance
Dimension | How it’s measured |
---|---|
Accuracy | How well does a piece of information reflect reality? |
Completeness |
Does it fulfill users’ expectations as to how fully it represents the truth? |
Consistency |
Does information stored in one place match relevant data stored elsewhere? |
Timeliness |
Is your information available when users need it? |
Validity | Is information in a specific format, does it follow business rules, or is it in an unusable format? |
Uniqueness | Is this the only instance in which this information appears in the database? |
Accuracy
The term “accuracy” refers to the degree to which information correctly reflects an event, location, person, or other entity. For example, if a customer’s street address is correct, but the postal code does not match, then the data lacks accuracy. That can cause a multitude of problems.
What steps can you take to improve your accuracy? Ask yourself whether the information represents the reality of the situation. Is there incorrect data (that needs to be corrected)?
Completeness
Data is considered “complete” when it fulfills expectations of comprehensiveness. Let’s say that you ask the customer to supply his or her name. You might make a customer’s middle name optional, but as long as you have the first and last name, the data is considered to be complete. If, on the other hand, you have a database of prospective customers who registered on your website using a fake phone number such as (111) 111-1111, then you’re missing some important information that could be useful.
There are actions you can take to improve this data quality dimension. You’ll want to assess whether all of the requisite information is available, and whether there are any missing elements.
Consistency
At many companies, the same information may be stored in more than one place. If that information matches, it’s considered to be “consistent.” For example, if your human resources information systems say an employee doesn’t work for your company anymore, yet your payroll says he’s still receiving a check, that’s inconsistent. Customer information, likewise, is often inconsistent across multiple systems such as CRM and ERP.
To resolve issues with inconsistency, review your data sets to see if they’re the same in every instance. Are there any instances in which the information conflicts with itself? A sound data integration strategy will reduce inconsistency across multiple systems.
Timeliness
Is your information available right when it’s needed? That data quality dimension is called “timeliness.” Let’s say that you need financial information every quarter; if the data is ready when it’s supposed to be, it’s timely. There are other cases when timeliness can be even more important. If you’re using data analytics for fraud detection, for example, you’ll want access to real-time data (or at least very close to real-time).
The data quality dimension of timeliness is a user expectation. If your information isn’t ready exactly when you need it, it doesn’t fulfill that dimension.
Validity
Validity is a data quality dimension that refers to information that doesn’t conform to a specific format or doesn’t follow business rules. A popular example is birthdays – many systems ask you to enter your birthday in a specific format, and if you don’t, it’s invalid. Address information, likewise, must conform to a set of rules or it will be invalid. US zip codes are at least a 5-digit numeric string but sometimes may include a four-digit appendix. Each country has its own rules governing the validity of postal codes.
To meet this data quality dimension, you must confirm all of your information follows a specific format or business rules.
Uniqueness
“Unique” information means that there’s only one instance of it appearing in a database. Data duplication is a frequent occurrence. “Daniel A. Robertson” and “Dan A. Robertson” may well be the same person.
Meeting this data quality dimension involves reviewing your information to ensure that none of it is duplicated. Customer databases often contain duplicate entries. Enterprise-grade data matching and entity resolution solutions automatically discover additional records and apply a rules-based approach to de-duplicate records improve data quality.
How does your data measure up?
Data with high integrity has contextual richness, is well-governed, and is integrated across multiple systems so that your organization has a single view of the truth. Data with high integrity must also have high data quality, of course.
Are you fulfilling all possible data quality dimensions? Download a free scorecard to assess your data quality initiatives. Data quality solutions can help improve your score and ensure your data is accurate, consistent, and complete for confident business decisions.
To learn more, read our eBook: 4 Ways to Measure Data Quality