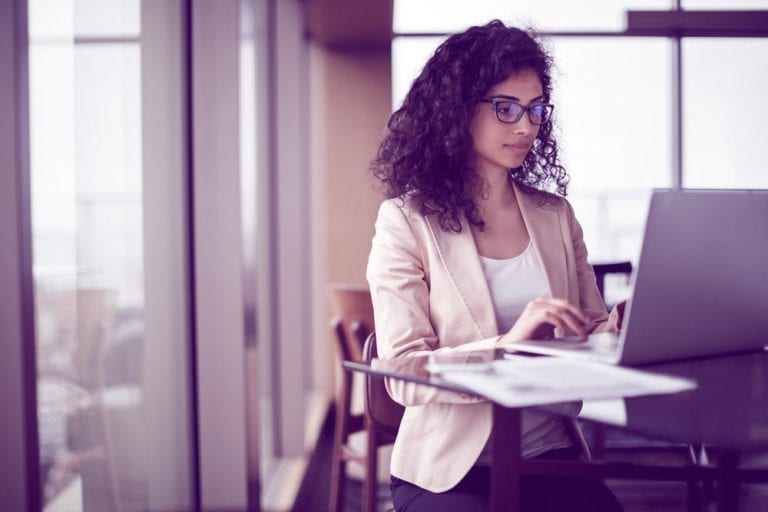
Data Cleansing: How to Get Rid of Bad Data
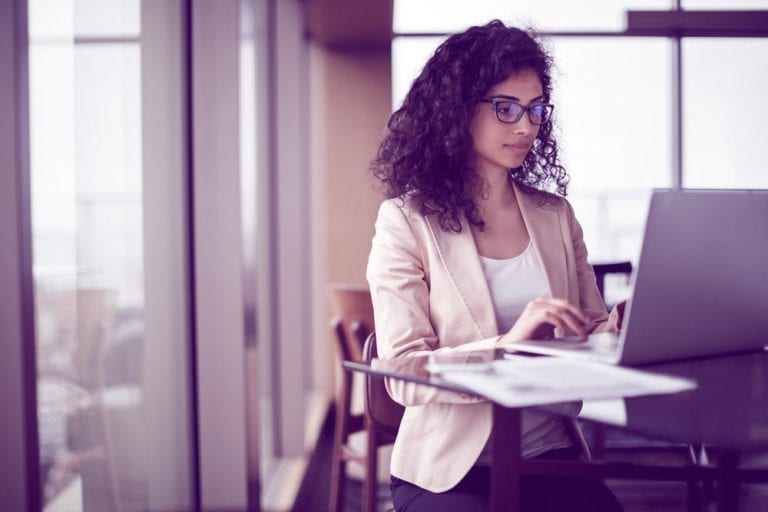
Bad data costs time and money. The majority of companies know that bad data is an issue, yet have no solid plan in place for eliminating bad data and its effects. In fact, one study concluded that 85 percent of companies know that they are using data that is incorrect or out of date, and as much as 40 percent of the data used to prospect new business is not accurate. Since data is the primary driving factor for facilitating new business, bad data is the one thing standing in the way of success. What is your data cleansing plan?
What is bad data?
Bad data includes data that is outdated or simply incorrect. This means addresses and job titles that are no longer valid, misspelled names, old phone numbers, or corrupted data that was never correct to begin with. Using bad data in your marketing efforts means you usually never get in touch with the people you are trying to market to, or worse, you reach them but alienate them by misspelling their name or getting their job title wrong.
Can your company afford to reach out to 40 percent of prospective customers who will never see your message or will become angry over your inaccurate information on them? Many customers assume that if you spell their name wrong or fail to recognize that they got a significant job promotion that you simply don’t care. This is far worse for your marketing efforts than if you’d never been able to reach them at all.
How to identify bad data
Most companies simply instruct their marketing workers to “fix it when you find it.” But this doesn’t solve the problem at all.
Bad data can be identified and removed, helping the entire marketing campaign to be more successful. The first thing you can do is search data fields for data that obviously doesn’t belong, such as names with numeric characters. Data files can also be checked against more current or accurate records to find and remove discrepancies.
How to cleanse bad data
Data should be cleansed at least quarterly. Create an invalid data file and a valid data file. Check for invalid characters within your data fields, such as letters in phone numbers or strange characters in addresses. Move valid data to the valid data file and invalid data to the invalid data file.
Since this process is quite time consuming, many businesses choose to depend on an outside vendor to help identify and remove bad data sets, especially before rolling out a new marketing campaign.
White paper
Data Quality Gets Smart
In today’s competitive landscape, data quality matters more than ever. That’s why data users across every industry need to take a more active role in data quality.
Cleansing bad data allows you to utilize good data
Not only does removing bad data help you reach prospective customers, it also opens the door to effective analysis on the good data that is left after a data cleansing. Various tools are available to allow you to see new patterns and relationships among your data, so that you can identify ways to improve your marketing efforts and boost customer service ratings.
For example, if you analyze the data and begin to see a pattern of customers dropping out of your services after three months, you can then identify what happens after about three months that causes customers to leave. You can then correct the issue to improve customer retention rates.
Big data is what will drive the future of business. But only good data is useful in generating business and retaining that business. Are you heading into the future with a plan to assure your data is legit?
Precisely offers data matching and entity resolution solutions to help you cleanse your data. For more information, be sure to check out our white paper: Data Quality Gets Smart