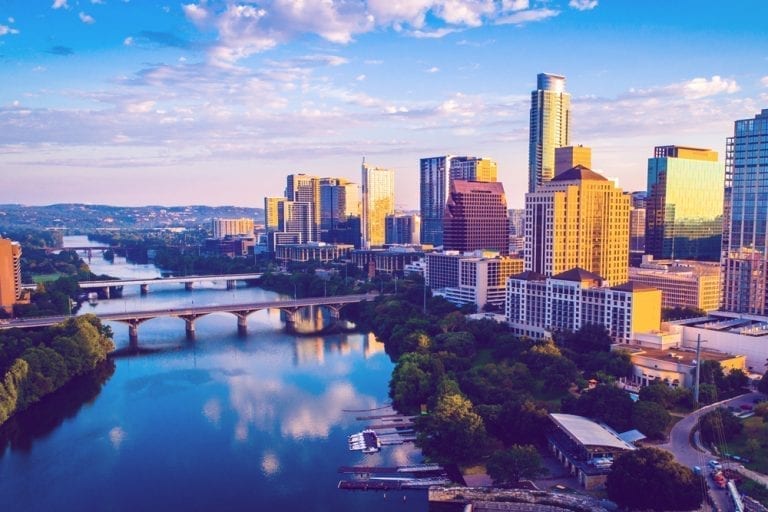
Empower Data Science Initiatives with Geo Spatial Data Enrichment
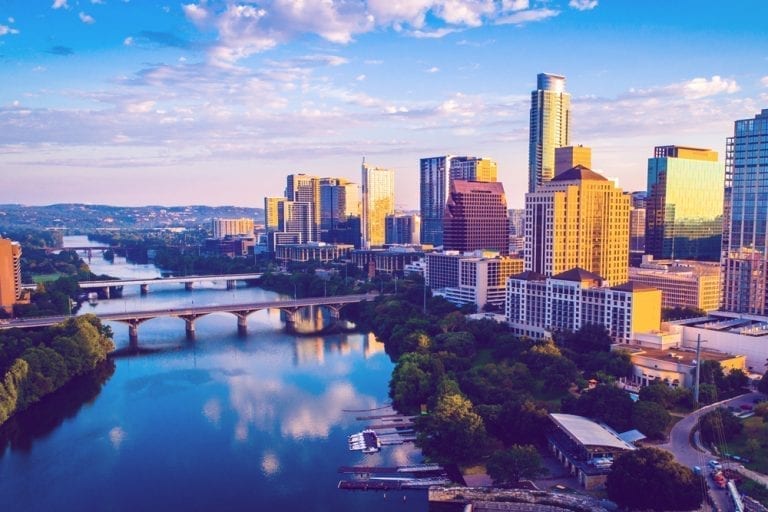
In the past few years, data science has benefited from tremendous new capabilities driven by cloud analytics platforms, artificial intelligence and machine learning, improvements in integration technology, and an explosion of new data sources driven by mobile devices, IoT sensors, vehicle telematics, and more. Location intelligence is significantly enhancing that with geo spatial data enrichment, improving data quality and adding depth and context to existing corporate data.
The two faces of location intelligence
Broadly speaking, location intelligence (LI) falls into two functional categories. The first one is “analytical LI.” This is what people usually think of when they envision interactive dashboards or mapping applications with which a user can explore data across multiple geographies, drawing information and insights about location attributes, characteristics of a bounded area, or travel routes. With analytical LI, there is always a person at the helm, a business user who seeks to better understand a specific domain within a geographical context.
The second category is operational LI. This is where geo-enrichment of existing corporate data takes place. It is almost always automated, so it typically happens in the background. Operational LI doesn’t necessarily involve maps; in fact, in most cases, it will not even require human interaction at all. Rather, operational LI is about inserting data into automated workflows. The resulting data enrichment adds context to existing data and supports improved decision making.
Keeping in mind these two categories of location intelligence applications, let’s explore some examples of how spatial data enrichment can empower data science initiatives.
Watch our Webcast
Empowering Data Scientists to Utilize Geospatial Data at Scale – Why “Where” is a Must-Have for Data Science
Explore what your organization needs to know to tap the potential of geo-enrichment and geospatial data.
Spatial data enrichment use cases
Let’s begin with a simple example that will be familiar to most Internet users: address type-ahead and address standardization. Most of us have had the experience of typing our address into an online form and seeing a list of possible addresses appear in a drop-down box. Likewise, when making a purchase, we’ve all been presented with a “corrected” version of the address we provided, perhaps with a four-digit postal code extension and other changes that standardize the information so that it will be clearly understood by shipping companies, credit card processing, and tax calculation engines.
From a data science perspective, this kind of standardization has obvious benefits. Consider, for example, the case of a street or road that has multiple name designations, or which contains abbreviations or accented characters. Address standardization ensures that such data is consistent across the entire enterprise, and that analytics can accurately render meaningful information and insights about it. Precisely Spectrum Geocoding provides these kinds of capabilities for resolving and standardizing addresses, and it can be configured to meet the unique needs of your company.
Another common use case revolves around sales tax compliance. Enforcement has ramped up in recent years, and rules for online purchases have changed significantly, especially since the Wayfair decision in 2018. With location intelligence, organizations can accurately determine the rates and jurisdictions for online purchases based on the customer’s location.
In the mortgage industry, location intelligence is helping companies to predict property values more accurately, with little or no direct human intervention. LI can take into account the characteristics of the surrounding community such as crime rates, available services and amenities, the quality of local schools, and proximity to public transportation. LI can provide information about comparable properties to arrive at an estimated value. This improves lending decisions and streamlines the decision-making process.
Insurance underwriters, likewise, can gain a better understanding of property characteristics by using location intelligence tools. This can include the application of risk models based on proximity to water, location relative to emergency services such as fire departments and fire hydrants, distance from combustible material, crime rates, and more.
Precisely offers over 9,000 data points that can be associated with a given location. Considering the fact that virtually every data point can be associated in some way or another with location, this opens up a vast world of opportunity for data scientists.
A four-step process for location intelligence insights
As data scientists look to expand the business value they provide, LI affords a natural opportunity to add context. To achieve this, they must undertake a four-step process:
Location determination: The process begins with the determination of a location, that is, establishing where a particular address, area, or point of interest exists geographically. This often begins with geocoding, resolving an address to a specific latitude and longitude. At Precisely, we provide an important additional value, which we call the PreciselyID. The PreciselyID is a unique identifier that designates a specific address and links that location to over 9,000 location-based attributes that are available from Precisely.
Feature selection: This step answers the question “What is nearby?” In other words, which points, lines, or areas of interest do we care about? Insurance underwriters, for example, would be interested in proximity to sources of risk such as bodies of water (flooding), combustible materials (wildfire), or high-crime areas. People shopping for a new home may be interested in the proximity of coffee shops, fitness facilities, or public transportation.
Data extraction: Next comes the question of what can be learned from the spatial data. This involves the selection of attributes, calculations, distances, and comparisons. How far is a home from the river? How close are combustible materials, and what are the wind patterns in the area? What are the drivetimes to local hospitals during both peak and off-peak hours?
Business insights: Finally, there is a decision or conclusion that arises from all this information. What is the risk level? How profitable might a store be in this location? Where do our customers spend their time on weekends? Which potential location for a new fire station would best serve the community’s needs?
The opportunities to leverage location intelligence in the field of data science are virtually boundless. As the adoption of artificial intelligence and machine learning continues to accelerate, LI will undoubtedly see broader adoption across a wide range of industries and use cases.
If your organization is seeking to improve results using a proven, data-driven approach, check out our recent webcast, Empowering Data Scientists to Utilize Geospatial Data at Scale – Why “Where” is a Must-Have for Data Science