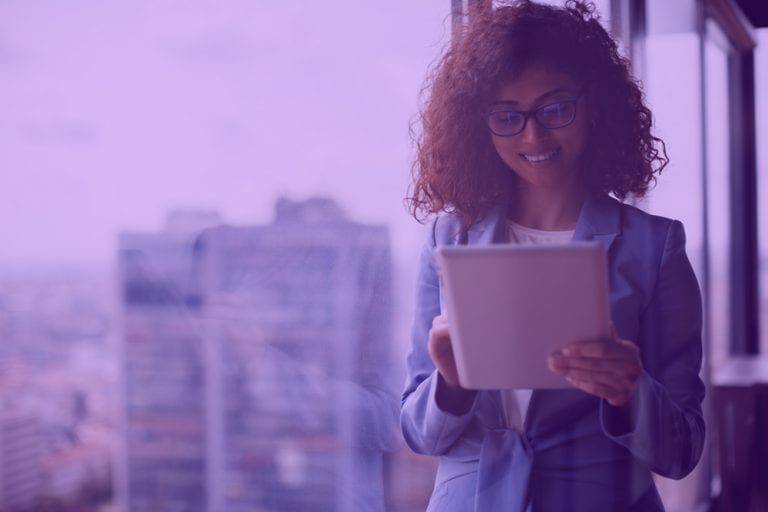
5 Steps to Data Quality That Set a Firm Foundation for Data Integrity
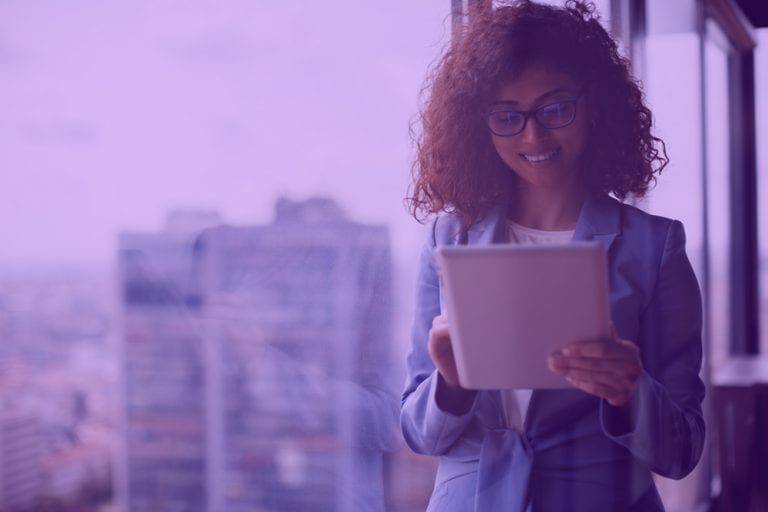
Today’s businesses have a greater opportunity than ever before to leverage the power of information. Forward-looking leaders understand that their approach to data integrity can be a critical differentiator in the competitive landscape. By effectively leveraging reliable, quality data, businesses can foster richer and more rewarding customer experiences, develop new products and revenue streams, increase efficiency, and get more value from their marketing spend.
Companies that fail to approach data effectively, on the other hand, risk missing out on important business opportunities, resulting in lost revenue, wasted money, and low productivity. Even worse, organizations may incur costly penalties if they are found to be out of compliance with data regulations, and they could suffer reputational damage as well.
To take full advantage of data as a strategic asset, business leaders must make data integrity a priority. At Precisely, we help organizations achieve better results by addressing these four pillars of data integrity:
- Enterprise-wide data integration ensures that data is consistently integrated according to a set of clear standards and applied consistently throughout the various systems within an organization.
- Data quality ensures that information is accurate, complete, and consistent.
- Location intelligence adds a geospatial component to bring location context to enterprise data.
- Data enrichment increases and extends the value of corporate data, combining it with external sources of information to enhance the benefits that an organization can draw from it.
As a pillar of data integrity, data quality is a discipline that business leaders must commit to as a priority. Following a five-step process to ensure data quality puts your organization firmly on the path to the accuracy, consistency, and context required for data integrity.
Step 1: Setting data quality objectives
Every worthwhile project begins with a clear statement of objectives. For any initiative aimed at improving the quality of data, it is critically important that project leaders clearly articulate the concrete benefits to stakeholders throughout the business. Although data quality generates value across multiple domains within an organization, it is often best to begin with finite, well-defined use cases that can be associated with tangible, measurable results.
Connecting your initiative with specific savings (or revenue opportunities) will help you build a case with stakeholders across multiple departments, as well as with executive management. In fact, top-level executive sponsorship is virtually always a factor determining the success of data quality initiatives.
Step 2: Data discovery and inventory
In most complex organizations, data may be housed in a number of different places. That can include a multitude of disconnected software systems, individual spreadsheets, and other files stored on users’ hard drives, and even on paper documents stored in filing cabinets. Assembling a complete inventory of all that data can be daunting, but it is an important step early in the process.
A comprehensive catalog of data assets assures that nothing is being left out. As the business moves forward toward achieving consistency, accuracy, and completeness of all that information, it’s critical that the project team operates with a complete picture of the organization’s data assets in mind.
The data discovery and inventory process helps organizations to not only identify where data resides, but also to understand the size and scope of common issues such as duplication, standardization, completeness, and the suitability of data assets to the organization’s objectives.
Step 3: Evaluation
After the data has been inventoried, the project team should undertake a comprehensive evaluation of that information to discover and prioritize any quality issues that may exist. That evaluation may include an assessment of the business processes, software systems, and ancillary tools required to ensure that corporate data serves its intended purpose.
The evaluation process is likely to uncover some fundamental quality issues including validation, standardization, and matching and de-duplication. This step also includes prioritization, which involves aligning the focus of the data quality project to the key business objectives defined in step number one and communicated to stakeholders.
Download the Forbes Insights report
The Data Differentiator - How Improving Data Quality Improves Business
This report from Forbes Insights looks at how improving data quality is a critical differentiator to business success. To learn more about how your organization can achieve data quality that lays the groundwork for true data integrity, download the full report.
Step 4: Establishing parameters for improvement
Step four is all about focus and execution. It begins with defining the criteria for success at a more granular level and creating a framework for a rules-based approach to building data quality.
Data quality is achieved by applying systematic tools and processes to the common problems of invalid, incomplete, or inconsistent information. Once a target level of data quality has been achieved, the application of those tools and processes should continue as a body of best practices that contribute to the ongoing improvement of the organization.
Step 5: Monitor progress
Finally, business leaders should be mindful that this is not a finite process. While it’s good to celebrate initial successes and the business benefits they produce, effective data management practices require ongoing attention and investment resources. That includes data quality and, ultimately, data integrity.
By establishing benchmarks for all four pillars of data integrity, organizations can maintain clear visibility to the results achieved and the value generated for the business as a result of the initiative. When benchmarks fall below the expected parameters, business leaders can take proactive measures to correct the problem.
Above all, it’s important that stakeholders throughout the organization understand the necessity of ongoing data initiatives so that corporate data assets continue to drive tangible value for the business into the future. Data quality is not a “one and done” proposition.
Business leaders have a unique opportunity today to build a competitive advantage through data. For those that wish to seize that opportunity, data integrity is a non-negotiable requirement. By building a meaningful strategy around data integration, data quality, location intelligence, and data enrichment, business leaders can lay a solid foundation for real digital transformation.
To learn more about how your organization can achieve data quality that lays the groundwork for true data integrity, download the Forbes Insights report titled The Data Differentiator: How Improving Data Quality Improves Business.