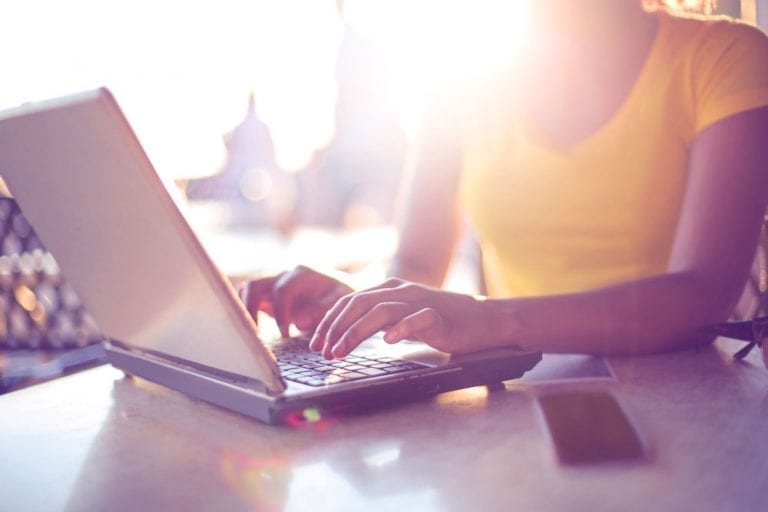
Solving 5 Big Data Governance Challenges in the Enterprise
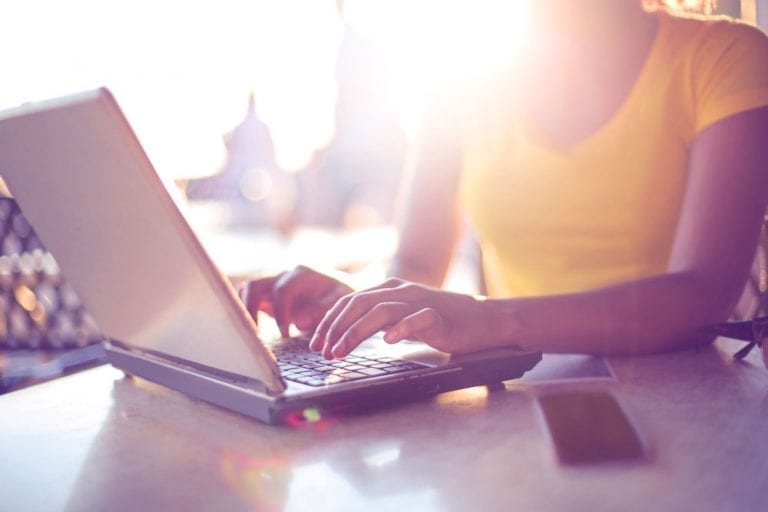
According to a recent report from Drexel University’s LeBow Center for Business Analytics, 77% of data and analytics professionals say that data-driven decision-making is an important goal of data programs. However, fewer than half of survey respondents rate their trust in data as “high” or “very high.” Poor data quality impedes the success of data programs, hampers data integration efforts, limits data integrity causing big data governance challenges.
That said, the same report shows that organizations with a data governance program are seeing improvements in the quality of data analytics and insights (57%) and the quality of data itself (60%).
The results are clear. To truly succeed in an increasingly data-driven world, organizations need data governance.
Data governance is the formal orchestration of people, processes, and technology to enable an organization to leverage data as an enterprise asset. Data governance provides a structured framework for decision-making and authority for data and data-related subjects.
Data governance challenges: traditional frameworks can’t scale
Formal governance provides significant benefits to the enterprise, and it’s increasingly important for compliance. The California Consumer Privacy Act, for example, requires that organizations understand what data they have, where it is stored, and how it is being used. Similar laws in other jurisdictions are raising the stakes for enterprises, compelling them to govern their data more effectively than they have in the past. Traditional frameworks for data governance often work well for smaller volumes of data, and for highly structured data. As the landscape of data changes, however, the old way of doing things falls short. The need for scalable data governance frameworks is growing.
Enterprises can face many challenges trying to govern their modern ecosystems. Here are five to consider.
1. More Data Sources Than Ever Before
The world has moved away from big monolithic systems that house most of their mission-critical data. Today, organizations augment large-scale ERP systems with CRM software and digital marketing automation, ecommerce systems, customer service tools, and more. Smaller, ad hoc data sets are common as well, often taking the form of Excel workbooks or small homegrown databases.
A data catalog provides a single, go-to source of truth for users throughout an organization. Simply put, data catalogs offer an inventory of data assets, a common definition of what the data means, and a shared understanding of how the data can be used. Developing a data catalog is a time-consuming process, made simpler and more manageable with the right technology tools.
2. The Rise of Unstructured Data
Governing structured data is relatively easy. It’s a fairly simple proposition to define the attributes of the data and detect records that fail to meet expectations.
The same does not hold true for unstructured data. Social media, audio, video, and online reviews represent a huge volume of unstructured data facing companies. Such unstructured data is messy, and it tends to move fast.
Unstructured data contains many quality dimensions. Governance should account for every point of potential failure in data assets. This includes risks like sensor signal loss, noise, and incorrect data defaults.
3. Higher Volumes of Data
The variety, volume, and velocity of data are increasing. Consumer and B2B interactions have gone digital. IoT devices deliver detailed data about time, temperature, position, and numerous other attributes. Video and audio data play a more important role than ever.
Gartner found that professionals spend 50% of their time searching for information and that, on average, they take 18 minutes to locate each document. As data volumes grow, the problem is getting worse, highlighting the urgent need for automation and rules-based workflows to streamline data quality and data governance tasks.
4. Context is Critical
You can’t fully tap into the value of your organization’s data assets unless you understand how it fits within the larger context of the world that matters to you. Data governance helps companies develop a unified and holistic view of their own internal data, but it also lays a foundation for enriching data with geospatial context, demographic detail, and other factors that add value to the insights and decisions that emerge from the data.
Compliance, security, and business value are critical. Each of these can impact data integrity, storage, and access requirements, like masking personally identifiable information (PII). Data governance frameworks help organizations address these must-have requirements while maximizing the contextual richness of their data, thereby increasing business value.
5. Siloed Data Governance Efforts are Bound to Struggle
Governance efforts have little impact if they are not designed with people, processes, and technology in mind. Data governance frameworks must be holistic, based on cross-functional collaboration, shared terminology, and a common set of standards and metrics. Even organization-wide transparency may not be enough. Governance can’t exist separately from data quality monitoring technologies.
You Can’t Govern Data without Quality and Profiling
Data governance has a mutually beneficial relationship with data quality. Understanding is the first step to creating policies for data collection, storage, access, and quality. Business logic, policy, and metrics can only work if they’re measured and monitored. Using the Precisely Data Integrity Suite is a best practice to unify data quality and governance.
Governance translates business strategy and regulations into policies and metrics. The Precisely Data Integrity Suite creates an interactive catalog of data sources and business definitions to spark cross-functional collaboration between data stewards.
The Precisely Data Integrity Suite can profile big data environments at scale, providing a steady stream of insights to continuously measure metrics, policy, and risk.
An effective big data governance framework requires real-time profiling, quality, and business context. A symbiotic relationship between governance and quality technologies is the key to success.
To learn more about big data governance challenges, read our eBook: Fueling Enterprise Data Governance with Data Quality.