eBook
4 Ways to Measure Data Quality
Assessing Data Quality
There are lots of good strategies that you can use to improve the quality of your data and build data best practices into your company’s DNA. Although the technical dimensions of data quality control are usually addressed by engineers, there should be a plan for enforcing best practices related to data quality measurement throughout the organization.
After all, virtually every employee comes into contact with data in one form or another these days. Data quality is everyone’s responsibility.
Assessing data quality on an ongoing basis is necessary to know how well the organization is doing at maximizing data quality. Otherwise, you’ll be investing time and money in a data quality strategy that may or may not be paying off.
To measure data quality – and track the effectiveness of data quality improvement efforts – you need, well, data. What does data quality assessment look like in practice? There are a variety of data and metrics that organizations can use to for data quality measurement. We’ll review of few of them here.
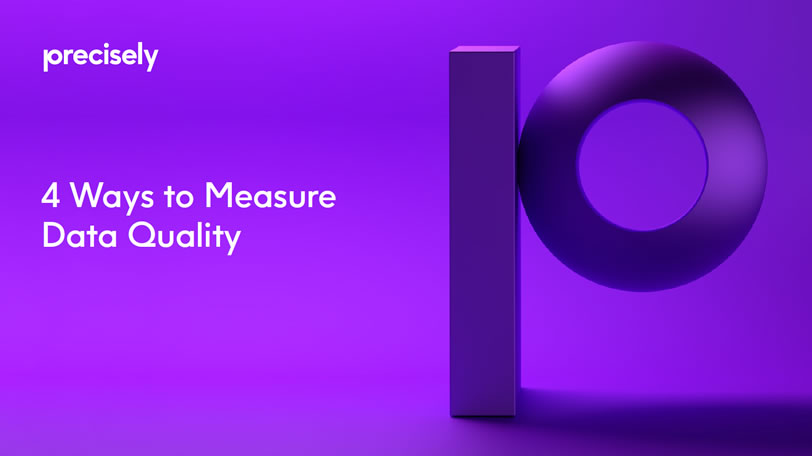
Database entry problems
In cases where you are working with structured datasets, you can track the number of database entry problems that exist within the datasets. The fewer data quality problems you have to start with, the faster you can turn your data into value. A few of these measurements include the ratio of data to errors and the number of empty values.
The ratio of data to errors
This is the most obvious type of data quality metric. It allows you to track how the number of known errors – such as missing, incomplete or redundant entries – within a data set corresponds to the size of the data set. If you find fewer errors while the size of your data stays the same or grows, you know that your data quality is improving.
Number of empty values
Empty values in fields that should have values indicate that information was missing or recorded in the wrong field. You can quantify how many empty fields you have within a data set, then monitor how the number changes over time.
Data analytics failure rates
The most obvious and direct measure of data quality is the rate at which your data analytics processes are successful. Success can be measured both in terms of technical errors during analytics operations, as well as in the more general sense of failure to achieve meaningful insight from a dataset even if there were no technical hiccups during analysis. The main purpose of a data quality plan is to enable effective data analytics, so fewer analytics failures mean you are doing a good job on the data quality front.
How long is your data time-to-value
Calculating how long it takes your team to derive results from a given data set is another way to measure data quality. While a number of factors (such as how automated your data transformation tools are) affect data time-to- value, data quality problems are one common problem that slows efforts to derive valuable information from data.
How much data you are processing
Your ability to process ever-larger volumes of data is one reflection of your ability to maintain data quality. If your data cleansing processes perform poorly, you are unlikely to be able to sustain a high volume of data processing and analytics.
Data transformation error rates
Problems with data transformation – that is, the process of taking data that is stored in one format and converting it to a different format – are often a sign of data quality problems. Your data transformation tools will struggle to work effectively with data that they encounter in unexpected formats, or that they cannot interpret because it lacks a consistent structure. By measuring the number of data transformation operations that fail (or take unacceptably long to complete) you can gain insight into the overall quality of your data.
“Your ability to process ever-larger volumes of data is one reflection of your ability to maintain data quality.”
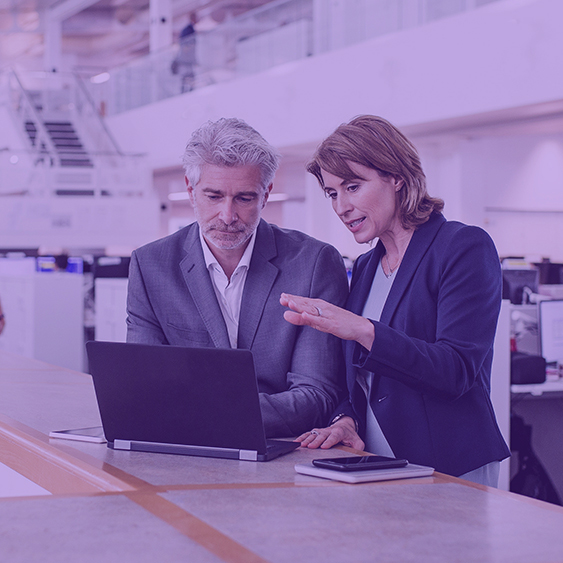
How much you pay for data storage
Are your data storage costs rising while the amount of data that you actually use stays the same? This is another possible sign of data quality issues. If you are storing data without using it, it could be because the data has quality problems. If, conversely, your storage costs decline while your data operations stay the same or grow, you’re likely improving the data quality front.
The metrics that make the most sense for you to measure will depend upon the specific needs of your organization, of course. The most important thing is to have some kind of data quality assessment plan in place, whatever its details may be.
The importance of data quality, and the amount of data you have to process will only increase with time at most organizations. Continually improving your ability to maintain data quality will help keep you prepared for the data analytics requirements of the future.