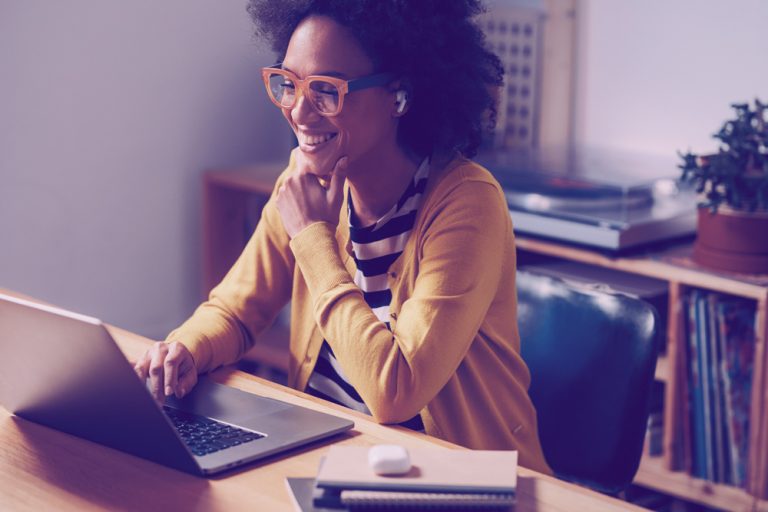
How Data Quality Builds Data Integrity
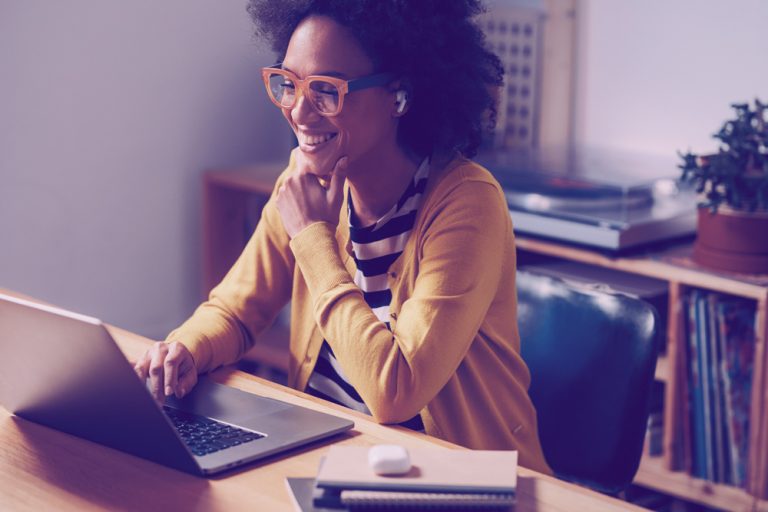
Data integrity is defined as data with accuracy, consistency, and context for confident decision-making. Achieving true data integrity calls for technical capabilities such as data integration, data quality management, data enrichment, and location intelligence. Together, these capabilities ensure that data provides a complete and accurate picture of reality–that is, “the truth, the whole truth, and nothing but the truth.”
As a quick refresher, data integration breaks down silos and ensures that data from across the enterprise can interoperate effectively and add maximum value along the way. Data quality ensures that information is complete, consistent, accurate, available, and timely, and that it fully conforms to business rules. Data enrichment provides for a complete picture, eliminating blind spots in your corporate data. Location intelligence adds a whole new world of geospatial context, further enriching data.
As data comes into greater focus as a foundational element of competitive advantage, enterprises need to pay close attention to all the technical capabilities that support data integrity. This article drills down into data quality specifically, exploring it in further detail and explaining how it contributes to the bigger picture of data integrity.
Let’s begin by exploring each of the six dimensions of data quality:
Completeness
Completeness of data indicates that all required information is present. It does not necessarily imply that each record contains a value for every possible field, but that all required information is present. For example, in a typical customer record pertaining to an individual, first name and last name will likely be required fields, whereas middle initial, prefix, and suffix may be optional.
To illustrate why completeness is vital, consider shipping and delivery. If an address is missing a postal code, then shipments or marketing literature may never reach the intended addressee. Shipping companies frequently charge penalties when this kind of information is incomplete. When those penalties are multiplied by a large number of incomplete addresses, it can add up to a significant expense.
Completeness can be an even greater necessity for things such as inventory items and their associated costs and selling prices. In these instances, missing information may result in incorrectly valued inventory or may prevent staff from transacting business for certain items.
2023 Data Integrity Trends & Insights
Results from a Survey of Data and Analytics Professionals
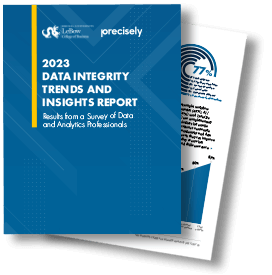
Consistency
Consistency refers to the degree to which data that exists across multiple systems is in sync. What happens when a business retains customer information in multiple systems? Imagine, for example, a hospital system that outsources billing to a third party, stores patient information within its electronic medical records systems, and sends out informational materials to all patients on a regular basis.
If a patient notifies the hospital that his or her address has changed, then that information needs to be replicated across all three of those systems (and potentially more), so that each functional area can continue to communicate effectively with the patient. Should inconsistencies emerge in the data, it is important to have systems in place to detect those discrepancies and fix them, either by using automated business rules or prompting human intervention to remedy any errors.
Validity
Data must also conform to pre-defined rules that dictate its validity. In the United States and Canada, for example, phone numbers consist of a 10-digit numeric string. Outside North America, that might not always be the case. In China, numbers can be 10 or 11 digits. In Kenya, all phone numbers are nine digits long.
Assuming that a database stores the country code associated with each customer record (e.g. “+1” for the US and Canada, or “+86” for China), you should be able to predict the format of possible values for the phone number field. If a customer is located in Toronto, Canada, and has a country code of +1, then their phone number should invariably consist of 10 digits. Anything else indicates a record that does not conform to business rules.
Accuracy
Accuracy is one of the simplest concepts in this list to understand. Simply put, it refers to whether or not a particular piece of information represents the truth. If the data indicate that the company sold 20,000 widgets last quarter, when in fact the company sold 22,000 widgets, then there is a problem with accuracy.
Very often, you can detect accuracy issues by establishing parameters around expected values. If a database of medical records lists a patient’s height as 60 feet tall, then you can be quite sure that there is a problem with the accuracy of that record. In all likelihood, the patient’s height is 6 feet and was simply keyed in incorrectly. By establishing business rules to detect these kinds of anomalies, businesses can increase the accuracy of their data.
Timeliness
In today’s rapidly changing business environment, the timeliness of information is more important than ever before. Timeliness is closely related to integration within the larger picture of data integrity (as are several of the other items within this list). When an enterprise relies on batch mode integration, scheduled to take place daily or weekly, information may become available too late.
Business leaders depend on accurate, up-to-date information. Increasingly, data is the source of competitive advantage for innovative enterprises because it drives insights that form the basis for effective and timely business decisions. In some cases, data that arrives too late may not be useful at all.
Uniqueness
One of the problems that plague customer relationship management systems throughout the world (and many other systems, for that matter) is the existence of duplicate records. When “Shawn Smith”, “Sean Smith” and “Sean A. Smith” are all listed as individual customers sharing the exact same home address, there is a very good chance they are, in fact, the same person.
Record duplication can emerge with business names as well. Abbreviated company names or variations of a name can result in duplicate records. This often happens with holding companies or “doing business as” (DBA) names as well. For a sales manager looking at a quarterly sales pipeline report, duplicate records can present a very real problem, because projected sales may easily be overstated without anyone being aware of it until it’s too late. For marketing departments, likewise, duplicate records are an issue. They often lead to wasted money spent mailing multiple pieces of collateral to the same person.
These six dimensions of data quality play a critically important role from the broader perspective of data integrity. Data quality is inextricably tied to the other three capabilities on which data integrity is built–data integration, data enrichment, and location intelligence.
Precisely partnered with Drexel University’s LeBow College of Business to survey more than 450 data and analytics professionals worldwide about the state of their data programs. Now, we’re sharing the ground-breaking results in the 2023 Data Integrity Trends and Insights Report.