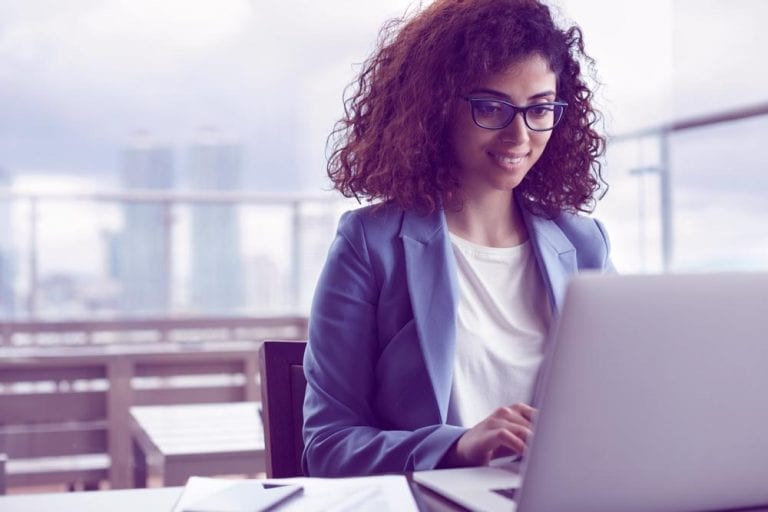
Developing a Data Quality Framework: How to Herd the Cats
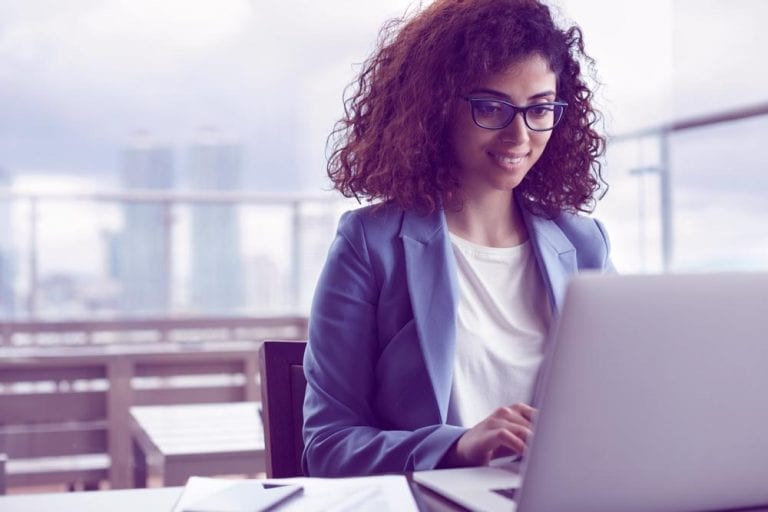
Developing a data quality framework is reminiscent of herding cats. You have to bring together disparate groups to establish a set of standards to maintain data quality, when these groups don’t want to be brought together and don’t agree on the standards.
This task isn’t impossible, though. Read on to learn how to create a data quality framework, how to roll it out according to best practices, and how to answer questions about who owns the framework.
Creating a data quality framework
There are several steps involved in creating a data quality framework. First, you define the goals for improving data quality, who owns the data (more on that later), the stakeholders, what business processes will be impacted, and what the data rules will be.
As the name implies, the goal for improving data quality is what you want to get out of your information. If you have customer data, you want it to be accurate and consistent. The business processes impacted will be whatever processes are used to gather information; again, using the customer data example, those processes might be order entry and invoicing. Your data rules will be the rules that ensure data quality standards are met.
After you’ve developed a definition, you have to assess your existing information against the rules you’ve developed. Next, you must analyze the results of your assessment – is there a gap between your current information and your goals? If yes, the following phase, Improvement, takes a step towards closing that gap by creating an improvement plan. The phase after that, Implementation, puts that plan into action.
The last step, Control, involves periodically verifying that the data quality is consistent with business goals and that the rules are in place. So, how do you successfully roll out a data quality framework?
One of the most important things to remember is that your data quality framework must fit into your existing organizational culture and its workflows. Understanding how those two aspects shape your firm’s data will go a long way towards preventing data quality problems.
Who owns the data?
As we promised earlier, we’re going to delve into who owns the data in the first place. Should IT take responsibility for it, or should the business unit be the owner of the information?
The answer is actually that both units should take joint ownership. While that seems like a recipe for disaster, this ownership plan ensures that IT and the business unit have a stake in preventing data quality issues from causing issues such as bad decision making and regulatory fines.
Developing a data quality framework, as well as determining data ownership, has several benefits: you make better decisions, you avoid compliance issues, and business units will see improved collaboration because they trust corporate information.
Precisely’s data quality solutions can help you build data quality framework.