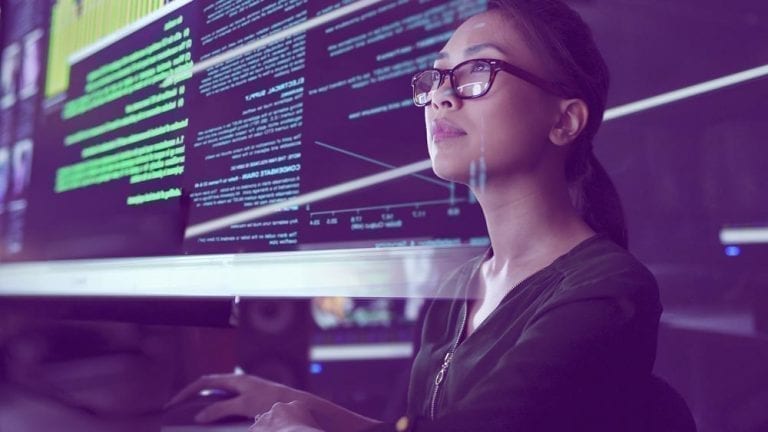
Data Quality Scorecard: How Does Your Company Measure Up?
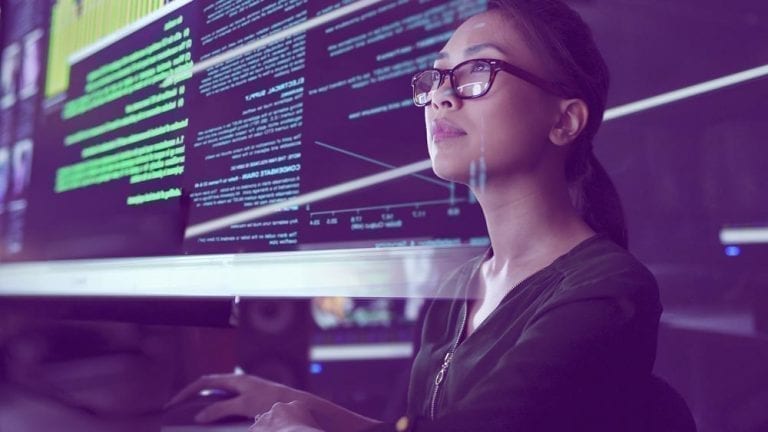
You know a data quality strategy is important. But do you know how to assess how good of a job your company is doing at achieving data quality? Keep reading for tips on developing a data quality scorecard for your organization.
Data quality refers to the ability of a given set of data to serve a specific purpose. Achieving data quality is important because, without it, you will struggle to put your data to work for you. In fact, a lack of data quality could mean that your data causes you more headaches than it’s worth.
Implementing data quality strategy
In order to maximize data quality, your company should have an overall data quality strategy in place. Although the technical dimensions of data quality control can usually be addressed only by engineers, there should be a plan for enforcing best practices related to data quality throughout the organization.
After all, virtually every employee comes into contact with data in one form or another these days. That’s why data quality is everyone’s responsibility.
Assessing data quality
When you make data quality the responsibility of the entire organization, it’s important to assess on an ongoing basis, how well the organization is doing at maximizing data quality. Otherwise, you have no way of knowing how much benefit you are reaping from your data quality strategy, or determining how to make it better.
Data quality problems result from issues like inconsistent data formatting, redundant or missing entries within databases and a lack of data structure.
Read our eBook
4 Ways to Measure Data Quality
See what data quality assessment looks like in practice. Review four key metrics organizations can use to measure data quality
You can evaluate the effectiveness of your data quality operations by tracking the following metrics:
- Data analytics failure rates – The most obvious and direct measure of data quality is the rate at which your data analytics processes are successful. Success can be measured both in terms of technical errors during analytics operations, as well as in the more general sense of failure to achieve meaningful insight from a dataset even if there were no technical hiccups during analysis. The main purpose of a data quality plan is to enable effective data analytics, so fewer analytics failures mean you are doing a good job on the data quality front.
- Database entry problems – In cases where you are working with structured datasets, you can track the number of database entry problems that exist within the datasets. For example, you might use data quality tools to assess the number of missing or redundant database entries. A decrease in the number of such errors within raw datasets means you are doing a good job of achieving high data quality at the time of data collection — which is great because the fewer data quality problems you have to start with, the faster you can turn your data into value.
- How long data quality tools take to analyze data – Another obvious way to assess your data quality strategy’s effectiveness is to track how long automated data quality tools take to complete their operations. Although the tools’ execution time can be affected by a number of factors that are unrelated to data quality, in general, higher-quality data can be processed more quickly.
- How long it takes to migrate data – Data migration times can be a proxy for measuring data quality. The reason why is that low-quality data is harder to transform when you migrate it; your data transformation tools will struggle to work effectively with data that they encounter in unexpected formats, or that they cannot interpret because it lacks a consistent structure. Other factors can affect migration time, of course, such as disk I/O. But if you can control for those variables in your assessments, data migration time is a good way of measuring overall data quality.
- How much data you are processing – Your ability to process ever-larger volumes of data is one reflection of your ability to maintain data quality. If you perform poorly on the data quality front, you are unlikely to be able to sustain a high volume of data processing and analytics.
- How much your employees know about data quality – In addition to tracking the technical data points listed above, you might consider quizzing your employees periodically to ask how much they know about data quality and your data quality strategy. Can they define data quality and identify common data quality mistakes? Assessing this knowledge will help you measure how well your organization understands and adheres to your data quality strategy.
The frequency at which you analyze the metrics listed above will vary depending on your organization’s needs, of course. As a rule of thumb, it might make sense to perform an analysis every six to twelve months, although analyzing your data quality effectiveness on a more frequent basis could be helpful when you have just implemented a data quality plan for the first time.
Regardless of how often you assess your data quality strategy, your end goal should be to strive for continuous improvement. The importance of data quality, and the amount of data you have to process will only increase with time at most organizations. Continually improving your ability to maintain data quality will help keep you prepared for the data analytics requirements of the future.
See how you measure up with our data quality scorecard
Are you fulfilling all possible data quality dimensions? Download a free scorecard to assess your own data quality initiatives. Precisely’s data quality products can help improve your score and ensure your data is accurate, consistent and complete for confident business decisions.
To learn more, read our eBook: 4 Ways to Measure Data Quality