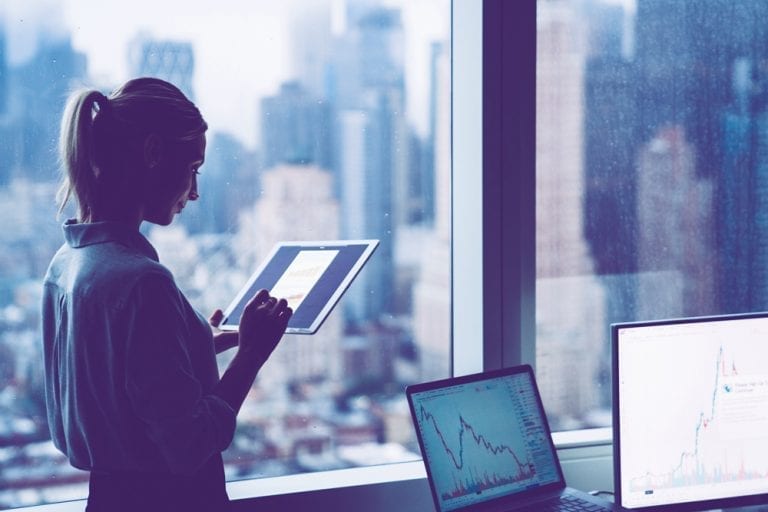
Data Quality: 4 Metrics to Monitor
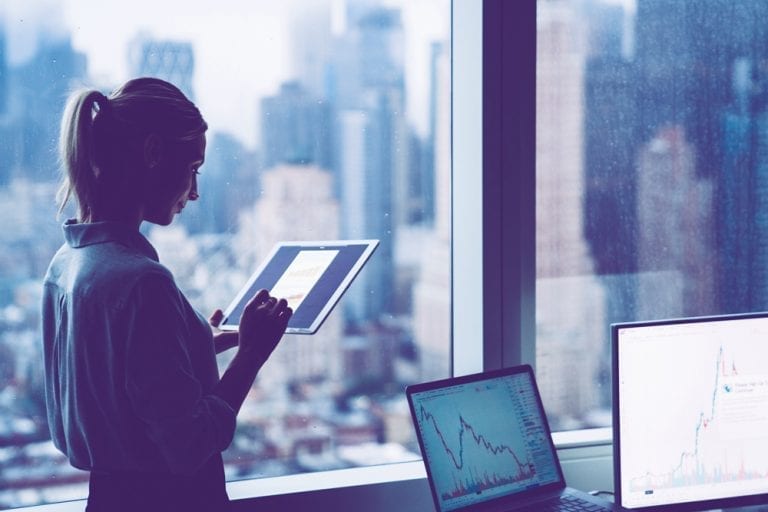
Artificial intelligence and advanced analytics will be significant drivers of competitive advantage in the coming decade. The volume of available data has skyrocketed in recent years, made possible by the proliferation of mobile devices, IoT sensors, clickstream analytics, and other technology. Leading organizations will align their strategies around a clear vision for data integrity. Data integrity consists of a reliable and adaptable platform for integration, a focus for data quality metrics, attention to location intelligence, and a sound data enrichment strategy.
While these four elements of data integrity are closely interrelated, we will focus here on the question of data quality specifically. Here at Precisely, we define data quality as having four key attributes. Data must be:
- Accurate. That is, it must be factually correct.
- Complete. Data must not have gaps; no information should be missing.
- Timely. Data must be current in order to be relevant and valuable.
- Accessible. It should be available to people in your organization who need it when they need it.
In data quality, as with many other things, perfection is an ideal that most organizations are unlikely to ever attain. You will never achieve perfect accuracy or completeness, particularly if your organization manages multiple software systems and databases. For many people, timeliness and accessibility seem easier to achieve, but even those are standards of perfection that can be challenging in many real-world situations.
So how do you assess how your business is doing with respect to data quality? If you are responsible for any aspect of data science or analytics in your organization, you already know the answer. You need to measure, monitor, and report. In other words, you need data quality metrics. Here are some suggested data quality metrics that you may want to consider as you are getting started with this process.
Error ratio
One important measure for accuracy is the error ratio. How much of your data includes errors, relative to the total volume of data? This is typically expressed as a percentage. Some organizations express this in positive terms, by stating that data is 96.2% accurate, for example. Others might express it as the percentage of errors (e.g. 3.8%). As long as the information is reported consistently, either option will work.
An error ratio may be determined in various ways, depending on the nature of the data and the potential for automation. A list of email addresses, for example, is generally easy to validate because bounced emails can be flagged automatically. When email opens are tracked, likewise, the validity of an address can be established programmatically.
Read our eBook
Fueling Enterprise Data Governance with Data Quality.
Data quality is strengthening the overall enterprise data governance framework. To learn more about how your business can benefit from improved data quality, read our ebook.
Duplicate record rate
A common problem in customer relationship management (CRM) databases is that of duplicate customer or contact records. This can happen for a number of reasons. In the case of business customers, it is common for salespeople or others in your organization to enter a new record using some variant of the company name. Once upon a time, IBM might have been entered as both “International Business Machines” and “IBM, Inc.” Abbreviated words such as “Association” (Assn.) or “Manufacturing” (Mfg.) can also be the source of such problems. Holding companies, DBAs (“doing business as” prefixes), corporate name changes, and brand nomenclature can lead to even further variation.
Similar issues often occur with individual contacts or consumers within a CRM database. When people move to a new address, have a name change, or undergo some other life change, they frequently end up being added to the CRM database a second time. That can result in a good deal of marketing money being wasted on duplicate mailings. For the sales team, it may result in an inaccurate pipeline and missed forecasts.
Fortunately, duplicate detection is a task that you can effectively automate when you have the right data quality tools, with attributes sophisticated enough to establish data matching rules at a granular level and employ algorithms that match soundalike names and similar addresses. By standardizing data formats, you can avoid some duplication, but effective data quality software will go a long way toward solving the problem and preventing it from reemerging.
Address validity percentage
What percentage of addresses are valid, complete, and consistent across multiple data sources? For many basic business functions, it’s critically important to have an accurate address. Customer shipments may be sent to the wrong location, for example, resulting in delays and/or shipping penalties. Sales tax calculations in many jurisdictions are driven by fine-grained location information which, if incorrect, could lead to discrepancies in tax liability.
The value of accurate addresses goes much further than that, though. Data scientists have become acutely aware of just how powerful location can be. When the precise geospatial position of an entity can be established accurately, it opens up a whole new world of information. For example, if you know where one of your customers lives, you can gain a much deeper understanding of their socioeconomic status, the type of dwelling they live in, where their children likely go to school, and so on.
Data time-to-value
When data takes too long to get from one system to another (that is, to be synchronized across multiple databases), the down-stream value of that information can be diminished. Historically, many companies have relied on business intelligence tools built on data warehouses that were updated once or twice a day. As technology has advanced, many organizations have moved to real-time or near real-time analytics built around streaming data to tools such as Hadoop, Snowflake, or Databricks.
In many cases, the value of analytics is particularly time-sensitive. Credit card fraud detection algorithms, for example, help financial services companies identify anomalies and prevent fraud quickly. If that data is not accessible to analytics platforms in real time, then its value diminishes rapidly. Data time-to-value will vary considerably from one data set to another, depending on its specific purpose.
These four examples of data quality metrics will be relevant to the vast majority of organizations. Other potential metrics include the percent of values that match across different databases (consistency), percentage of null values in a dataset (completeness), or data transformation error rates (accessibility and timeliness).
The important takeaway here is that every organization should have some means of measuring and monitoring data quality. The data quality metrics you choose will depend on your organization’s particular priorities.
To learn more about how your business can benefit from improved data quality, read our ebook Fueling Enterprise Data Governance with Data Quality.