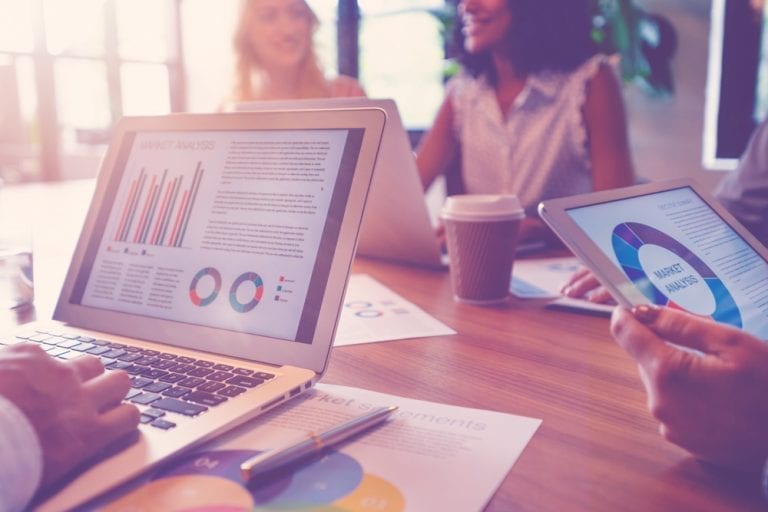
Cleanse, Match, and Standardize Cloud Data for Better Business Insights
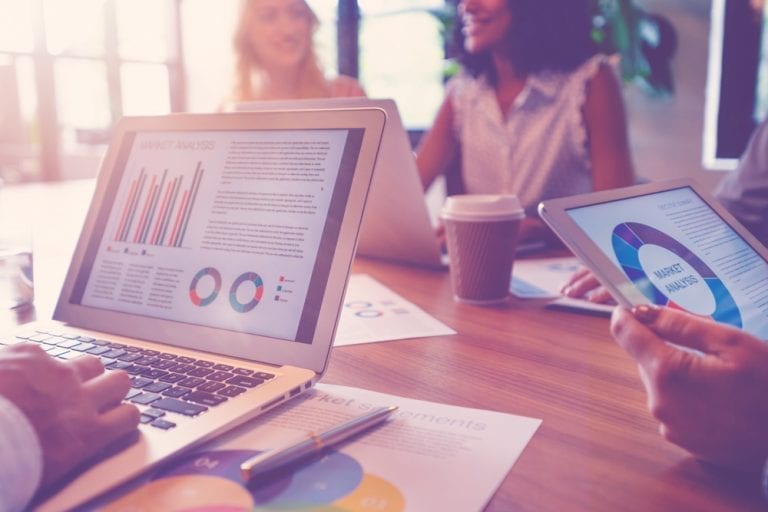
IT environments have grown vastly more complex in the past few decades, as enterprises have added a multitude of new data sources, all of which may necessitate data cleansing, matching, and standardization. Mainframes now sit alongside distributed systems that incorporate ERP, CRM, specialized logistics software, custom applications, and more. Mobile technologies, clickstream analysis, social media, digital marketing, and IoT devices are driving an explosion in the availability of fine-grained data for modern organizations. In addition, more and more business leaders have seen the potential of data enrichment, location intelligence, and other additive technologies that can dramatically increase the value of corporate data.
Without a clear and comprehensive plan to bring order to this chaos, business leaders stand little chance of achieving the clarity of vision and powerful insights available from today’s modern analytics applications.
Challenges of ingesting and standardizing data
Achieving the necessary level of quality (and then maintaining it) starts with a three-step process:
1. Discovering and profiling your data. The first challenge, and sometimes the most significant one, is merely understanding the universe of data assets available to you. Given the array of software systems and information sources within a typical organization, the process of discovering and cataloging data can be a daunting task. Those data sources may include everything from mainframe systems that house critical business data to static spreadsheets stored on a local server and shared among colleagues within a department. In today’s world, an increasing volume of business data is stored in the cloud, within specialized cloud data platforms or SaaS applications such as cloud CRM or ERP systems.
To effectively and efficiently catalog all of that information, organizations need tools that can automate the process of discovery of data sources, profiling schemas, generating statistics, and analyzing content. Those tools must also be capable of establishing value and pattern frequencies, detecting key dependencies, and identifying keys and joins.
The end result of that process is a clear answer to the questions: “What do we have?”, “Where is it located?”, and “What relationships and dependencies exist between our various data entities?”
Read our eBook
Governing Volume: Ensuring Trust and Quality in Big Data
Learn how data quality can increase trust in business intelligence and support a larger vision for data governance.
2. Analyzing current data quality. The second major challenge is to develop a clear understanding of the quality of your data. Where is your data lacking in accuracy, completeness, timeliness, and/or accessibility? Are addresses incorrect, or missing key information? Does your database of customers or prospects include large numbers of duplicate records? Have obsolete records been flagged as such or deleted? Is the timely availability of data limited by bottlenecks resulting from excessively slow or unreliable integration processes?
The answers to these questions usually require a substantial amount of input from stakeholders throughout the organization. That includes line-of-business employees who don’t necessarily have IT skills. They understand that data and can provide the critical business context that is required to determine where data quality is lacking, but they don’t have the database expertise to manipulate large data sets with the same tools that a data scientist or database manager might use. To effectively engage these stakeholders in the process, data quality initiatives must provide easy-to-use data analysis tools with which the average user can feel at home.
3. Implementing data quality rules and monitoring improvement. Automated application of data quality rules provides a highly scalable process for cleansing data on an ongoing basis, delivering trustworthy data that provides better results for both transactional processing and analytics. De-duplication of customer records, for example, prevents wasteful spending on redundant efforts to reach the same customers multiple times. By correcting and standardizing addresses, an organization can reduce the number of delayed or returned shipments, which saves money and increases customer satisfaction. Even greater benefits can be realized from analytics.
Reaping the business benefits of cleansing data
Modern cloud data platforms have emerged as a preferred environment for aggregating and analyzing large amounts of information. By bringing together data from multiple sources across the enterprise, cleansing and standardizing it, and then adding location intelligence and data enrichment from outside sources, enterprises can have a vastly more complete view of their customers, suppliers, and competitors than ever before.
This provides the jumping-off point for strategic analytics initiatives that have the potential to drive lasting competitive advantage. Retailers are using such analytics to develop a 360° view of their customers, many of whom have shifted to a mix of online and in-person interactions. Banks are using it to develop more effective models for assessing branch performance. Insurers are using artificial intelligence and machine learning to identify claims with the potential to turn into larger liabilities. Consumer products companies are building predictive models for better forecasting and distribution.
Big data comes with a big caveat. If the source data driving business insights is incomplete or inaccurate, it will produce results that fail to deliver their full potential. In other words, bad data can result in bad business decisions. Clean, quality data provides organizations with the confidence they need to make better data-driven decisions.
The big picture: Data governance
An effective data quality initiative does not exist in a vacuum. A good data quality program must be effectively integrated with an organization’s overall data governance vision. Many of the steps necessary for achieving and maintaining good data quality also lend themselves to good data governance. Likewise, data governance often demands that business rules be applied across the enterprise, and that a mechanism be in place to ensure that those rules are enforced adequately.
If your organization is seeking to rein in the chaos of multiple data sources, integrating and standardizing information from across your enterprise, Precisely can help. To learn more about how data quality can increase trust in business intelligence and support a larger vision for data governance, download our ebook Governing Volume: Ensuring Trust and Quality in Big Data today.