Analyst Report
Close Enough is Not Good Enough - Why Hyper-Accurate Location Data Matters for Insurance
The Business Case for Better Location Data
Insurers stake their businesses on their ability to accurately price risk when writing policies. For some, faith in their pricing is a point of pride. Take Progressive, for example. The auto insurer is so confident in the accuracy of its pricing that it facilitates comparison shopping for potential customers—making the bet it can afford to lose a policy that another insurer has underpriced, effectively passing off riskier customers to someone else’s business.
There are a number of data points that go into calculating the premium of a typical home or auto insurance policy: the claim history or driving record of the insured; whether there is a security system like a smoke or burglar alarm installed; the make, model and year of the car or construction of the home. Another contributing factor, of course, is location, whether it’s due to the area’s vehicle density or crime statistics or distance of homes from a coastline.
Insurers pay close attention to location for these reasons, but the current industry standard methods for determining a location—whether by zip code or street segment data—often substitutes an estimated location for the actual location. In many cases, the gap between the estimated and actual location is small enough to be insignificant, but where it’s not, there’s room for error—and that error can be costly.
Studies conducted by Perr & Knight for Precisely looked into the gap between the generally used estimated location and a more accurate method for insurers, to find out what impact the difference had on policy premium pricing. The studies found that around 5% of homeowner policies and a portion of auto policies—as many as 10% when looking at zip-code level data (see box, p. 5)—could be priced incorrectly because of imprecise location data. Crucially, the research discovered that the range of incorrect pricing—in both under- and overpriced premiums—could vary significantly. And that opens insurers up to adverse selection, in which they lose less-risky business to better-priced competitors and attract riskier policies with their own underpricing. We’ll discuss the research and its findings in more detail in Part II of this paper.
This Forbes Insights paper discusses the business case for hyper-accurate location data in insurance as well as considerations when improving location data.
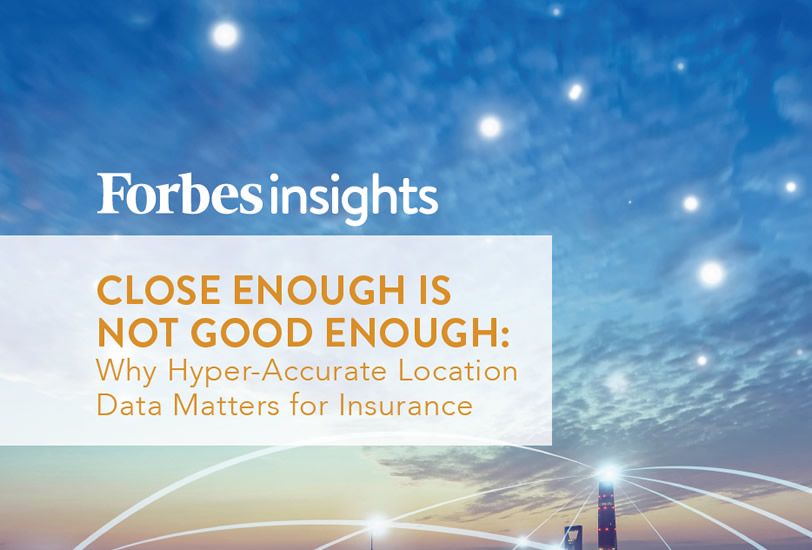