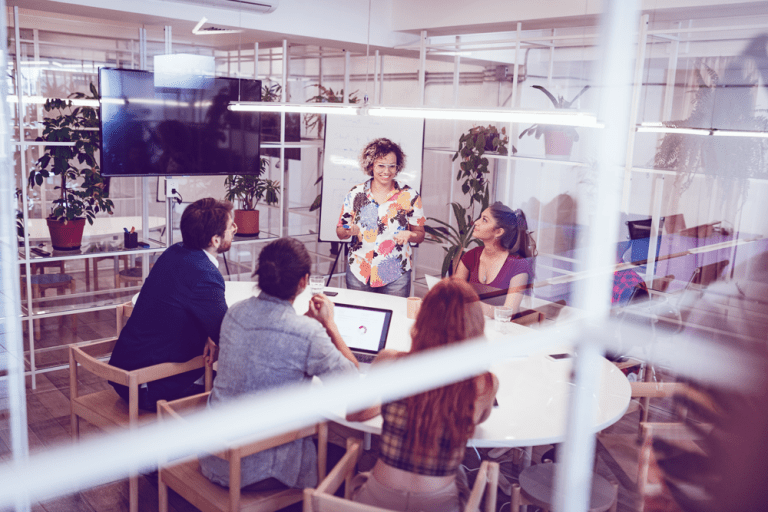
Four Steps to Building a Successful Data Governance Team
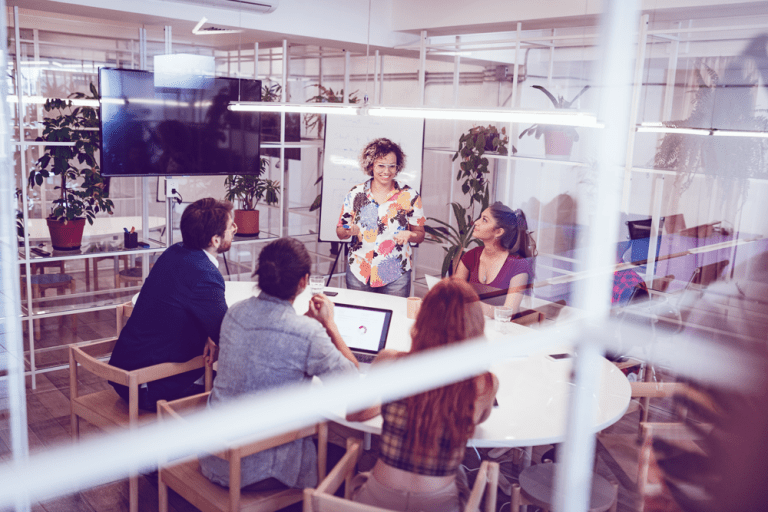
A successful data governance team requires alignment to clearly defined business requirements. Yet far too many data governance programs fail to achieve objectives because they miss this prerequisite. They also fail to address the elephant in the room: why should an organization invest in governance over data—what many consider their most important asset?
A governance team must answer these three questions regarding fundamental business requirements:
- Is governance necessary to achieve regulatory compliance?
- Is governance a critical success factor to achieve analytics insights faster?
- How can governance reduce the time, cost, and risk for business transformation/operational excellence initiatives?
For most organizations, data governance objectives are a combination of these three. But which one is your primary business driver?
If you can answer why you’re investing in governance, then not only will the governance team be behind it, but so will the organization. Governance is much more than the features and functionality of modern-day data governance tools. What’s overlooked is that a governance team is only successful when they fine tune the formula of people, process, and technology. Of course, a data governance tool can enable people and process—but first you must decide how to implement governance to maximize the probability of success, given the business drivers and organizational culture of the organization.
Defining a governance strategy
This leads to the next critical question: what’s your data governance approach? Do you wish to catalog every data element like a public library? This is called the “bottoms-up” approach. Perhaps you need to govern business processes and associated data to drive a business outcome? This is referred to as the “middle-out” strategy. Or it could be that your organization wants governance to monitor and manage data around the goals, objectives, and metrics of the business. This is the “top-down” approach.
No matter what approach you choose, the governance team needs to socialize the approach to gain organizational buy-in that this is the right governance strategy, based on company culture and goals. However, this doesn’t mean that the other approaches will never be addressed. For example, one organization chose a “middle-out” approach to govern a new product introduction process, and then evolved it to key KPI’s and metrics that exemplify the “top-down” approach. The point is to choose one as a logical starting point and evolve as the data governance program achieves organizational acceptance and momentum.
Defining data governance
While there are many definitions of data governance, we define it as the formal orchestration of people, processes, and technology to enable an organization to leverage data as an enterprise asset. It’s important to note that every organization customizes the definition based on its unique culture and the business drivers of their governance program. Nevertheless, what’s equally important is building a strong team to develop and mature the data governance strategy. Selecting the right team can make or break the success of a program.
The data governance team is typically responsible for gaining budget approval, setting governance goals and priorities, architecting the data governance model, selecting technologies to adopt, and evangelizing the program. In many ways, the team is the pipeline to creating a data-driven culture because they build excitement and energy across the entire company, to ensure continuous utilization, collaboration, and communication around data.
Read our eBook
Data Governance 101: Overcoming Data Governance Challenges
Learn more about the challenges associated with data governance and how to operationalize solutions.
Let’s now delve into the four critical steps that businesses must address to establish a prosperous data governance program:
- Establishing a leadership team
- Selecting management-level employees
- Appointing data owners, stewards, and users
- Choosing modern technologies
Establishing data governance leadership
Successful data governance starts at the leadership level. Businesses typically appoint a Chief Data Officer (CDO) or other senior executive to spearhead governance efforts. The CDO is responsible for overseeing the entire team and ensuring that data governance efforts remain on track, on budget, and generate expected results or ROI. The CDO is also responsible for communicating procedures and monitoring success.
Working under the CDO is a team of executive-level leadership from various departments across the business, such as marketing, human resources, and IT.
As a unified group, the CDO and the data governance leadership team develop and establish data governance processes that include data policies and procedures, while overseeing the entire program.
After the leadership team is in place, the next step is to develop the remainder of the team.
Building the data governance team
Various departments use data for different purposes, making it critical to appoint data governance advocates from diverging lines of business. Representatives from different departments are tasked with fostering collaboration across the enterprise to ensure governance processes are followed, compliance standards are met, and data literacy is cultivated.
Together, these data governance team members work to establish common data definitions, implement and monitor data quality scores, enact governance metrics, and assign roles and responsibilities to data owners, users and stewards. These governance initiatives promote data understanding, encourage data utilization, and build trust in data assets, to encourage data consumers to engage with data and generate meaningful business insights.
Beyond this data governance team, the designated data owners, stewards, and users also play critical roles in a successful data governance strategy.
Appointing data owners, stewards and users
Data owners, stewards, and users each have different data governance roles and responsibilities:
- Data owners are responsible for ensuring data quality doesn’t degrade as it flows through a business’s data supply chain, all regulatory requirements are met, and that data is used appropriately according to defined policies and procedures.
- Data stewards supervise the analysis of data sets, produce easily digestible reports for business users, and answer data questions.
- Data users must follow all established guidelines and policies outlined by management and report any data abnormalities they uncover to the appropriate data owner.
It’s also important to choose names for these roles that are meaningful to the organizations. In some instances, people are averse to the term “data owner,” as they believe no one person owns the data. In that case, you might call it a “data custodian” instead.
Once the data governance team is in place and processes are created, the organization must select technologies that will ensure comprehensive, successful data governance. To ensure quality data that produces better business decision-making, organizations need a tool that features integrated data management capabilities on an enterprise scale.
Choosing Modern Technologies
To facilitate an effective data governance program, the governance team must select an enterprise data intelligence platform that delivers a broad range of integrated capabilities for data governance, data quality and analytics.
Data governance capabilities should deliver clear visibility into an organization’s data landscape, including available data, assigned owners/stewards, data lineage and usage, as well as associated definitions, synonyms, and business attributes. Take, for example, the “top-down” governance approach. In this scenario, a tool must incorporate an easy-to-visualize governance dashboard to show key KPIs and metrics to gauge progress towards achieving key business objectives.
In addition, the platform should encourage collaboration through visual interfaces and workflows, to foster a data community that brings people and data together. Workflows are of particular importance for two reasons: first, workflows for processes such as data access requests, reporting a data quality issue, or suggesting a new governance term should be easy to build and not require a coding handbook. Secondly, workflows don’t just need to be triggered by a human. Workflows should be automatically triggered for events like a data quality metric dipping below an acceptable threshold. This automatic workflow event promptly alerts data owners and stewards that an issue requires their attention. No one wants to find out a data quality issue has existed for months and is only found by accident.
The platform should also empower all users to easily define, track, and manage all aspects of their data assets.
Data quality is an integral part of any data governance strategy, and the data management solution must ensure the accuracy and reliability of data throughout the data supply chain with high-level and detailed quality metrics. For example, data quality checks for data profiling, consistency, conformity, completeness, timeliness, and reconciliation are often the “devil’s in the details” metrics that analytics users want to inspect before they trust that the data set is fit for purpose.
By taking the appropriate steps to build an inclusive data governance team and electing state-of-the-art technologies, businesses can increase the value of enterprise data assets across the entire organization.
Learn more about the challenges associated with data governance and how to operationalize solutions. Read our eBook Data Governance 101: Moving Past Challenges to Operationalization.