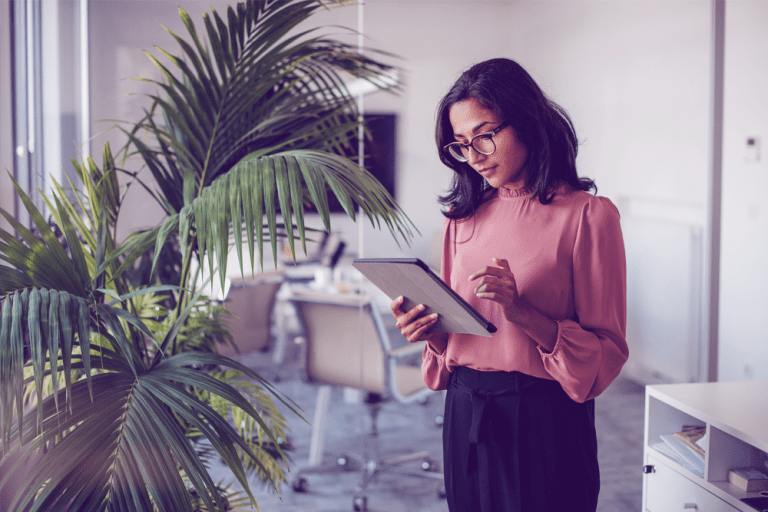
Why a Data Supply Chain is Required in the Age of Big Data
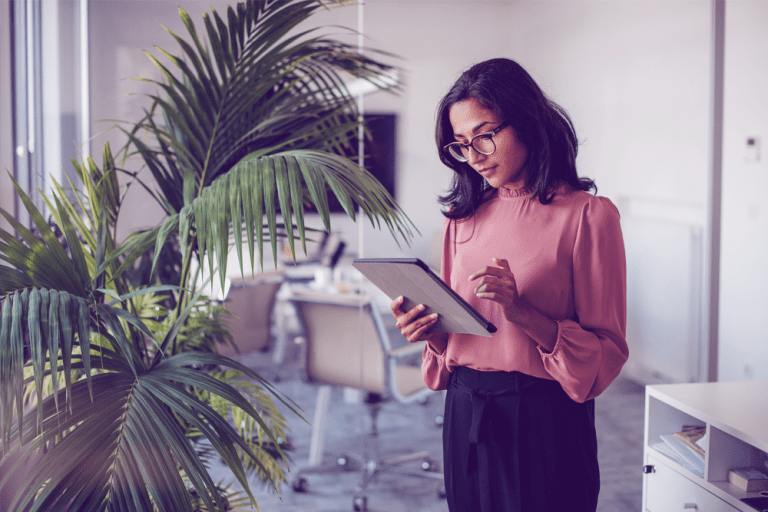
The promise of big data rests on the laurels of customer impact and value creation at fascinating speeds. However, the more data an organization collects directly correlates to how difficult it is to manage, analyze and achieve these values. It’s not for lack of interest, value, or investment. Many organizations realize that winning requires harnessing the power of big data to become an industry juggernaut and using data-driven decision making to tailor specific information to understand customers’ needs before they do. Yet many organizations are coming up short when it comes to their big data initiatives. So, what’s an organization to do that’s looking to harness the power of big data, yet doesn’t want to become a statistic as noted above? To make better business decisions, organizations are repurposing the supply chain management discipline in a new way – the data supply chain.
What is a data supply chain?
To understand a data supply chain, start by picturing a traditional supply chain – the sequence of processes that transform raw materials to finished goods – and then to the distribution of the finished goods. Supply chain management tracks how goods and services flow through the chain effectively and efficiently. The challenge is that most organizations have a data supply chain, but they have zero visibility into how it works. This can quickly become the Achilles heel that will undermine your big data legacy.
Now correlate this approach to organizational data. Data is the raw material that enters an organization. That data is then stored, processed, and distributed for analysis – akin to the transition of the raw material to the distribution of a finished product. Or in our case, from raw data into insights. The last leg of a data supply chain involves an easily searchable data portal that allows the business user to discover and order the data to their particular environment.
To break it down, the data supply chain consists of three parts. First, on the supply side, data is created, captured, and collected. Next during the middle stage, management and exchange, the data is enriched, curated, controlled, and improved. Then on the demand side, data is utilized, consumed, and leveraged. Those that master the process will become leaders in their industry that the laggards will try to emulate.
Read our Solution Sheet
Precisely Data360
To learn more, read our solution sheet and explore how a platform approach to validating, governing, and analyzing your data will connect the dots of your data supply chain.
Data storage challenges
Organizations feel overwhelmed by the volume, variety, and velocity of data entering their system. If their infrastructure is dated, it’s an even bigger challenge because there is nowhere to store the data. As a result, IT ends up tossing out the data or only storing it for a short period of time before it gets deleted and the business knows that data has a shelf life before it’s no longer of significant value to analyze. Any gaps in the supply chain prevent organizations from running predictive analytics because the historical data is missing.
Such scenarios have led organizations to adopt data lakes and other big data storage strategies which, while flexible and cost-effective, have brought other difficulties to the forefront. One of those challenges includes data discovery or an organized way of finding data once it is stored. Organizations just load data into a repository with no attempts to clearly define what the data is or put it into a relational or query structure. This clouds up the data lake and makes it nearly impossible to find or deliver the data to the business user.
The benefits of a data supply chain
Every business that deals with data has a data supply chain, but most businesses are focused on taking in data and/or analyzing the data that they have. What they are missing are the processes that enable meaningful analytics and ultimately, insight. The benefits of an optimized data supply chain are like the benefits of an organized kitchen. The best chef in the world will struggle to make a good meal in a messy kitchen with unlabeled, or worse, mislabeled ingredients on the shelves. If you think of data as the ingredients in the messy kitchen, you will be describing the data situation at many firms. Even if the chef has an assistant, they won’t know what ingredients need to be bought from the grocery store because they don’t know what is in the cupboard. The determined chef will persevere – they will sort through the cupboards to find out what’s there, but it could take all day to make a simple lunch, and there is always the danger that they put paprika in the soup instead of salt. Furthermore, you also don’t want to pay a world-class chef to clean the pots and pans, yet, this is basically what some businesses are doing when they hire the high-priced data scientists that spend their hours sifting through or ‘cleansing’ unreliable data.
Creating a data supply chain
To create a successful data supply chain, organizations need to know where their data is and how to find it. If they don’t, the process is undoubtedly strenuous and time consuming. Requesting and receiving data using a single, easily searchable portal can improve search-ability, promote higher productivity, enable compliance, and streamline data supply chain management. Luckily, new solutions automate the process. But for meaningful analysis of data to be successful, three types of initiatives need to be in place:
- Track conceptual metadata: Conceptual metadata is the meaning and purpose of a data set from a business standpoint. For example, a salesperson needs an address to send material to a current customer. The problem is that a customer might have several addresses – there is the address of the factory, the billing address, the general customer service address, and the address of the executive suite. To know which address is best for sending sales material, a seemingly simply data field like ‘address’ must be appropriately labeled and appended with meaningful metadata.
- Track data lineage: Data lineage helps organizations track where their data came from, what systems and processes it went through, how it was formatted, and how it was transferred. With all that information, organizations know exactly what they are dealing with when it comes to their data.
- Ensure data quality: Knowing organizational data is complete, accurate and consistent is paramount because business managers don’t know if their data is trustworthy or not. If they select low quality data, and are unaware of the quality issue, it can lead to flawed business decisions, and ultimately an organizational disaster.
When organizations track conceptual metadata, track data lineage, and ensure data quality, search-ability becomes possible, leading to end-to-end data supply chain success.
To learn more, read our solution sheet and explore how a platform approach to validating, governing, and analyzing your data will connect the dots of your data supply chain.