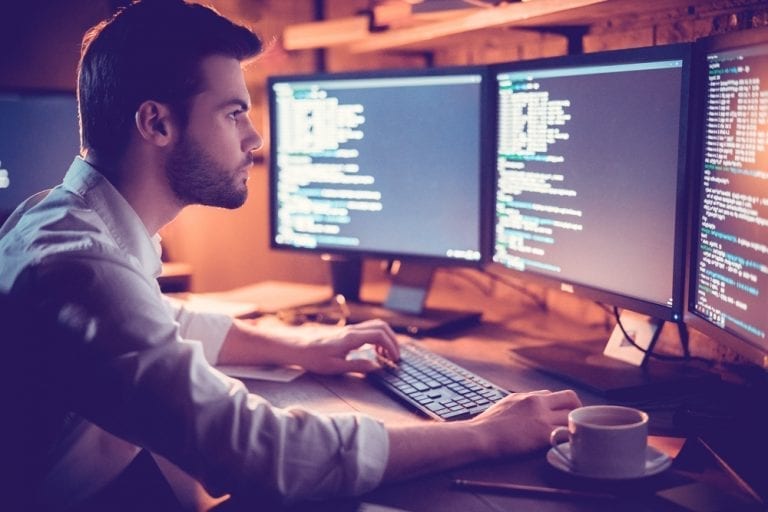
What Role Does Data Quality Play in Data Governance?
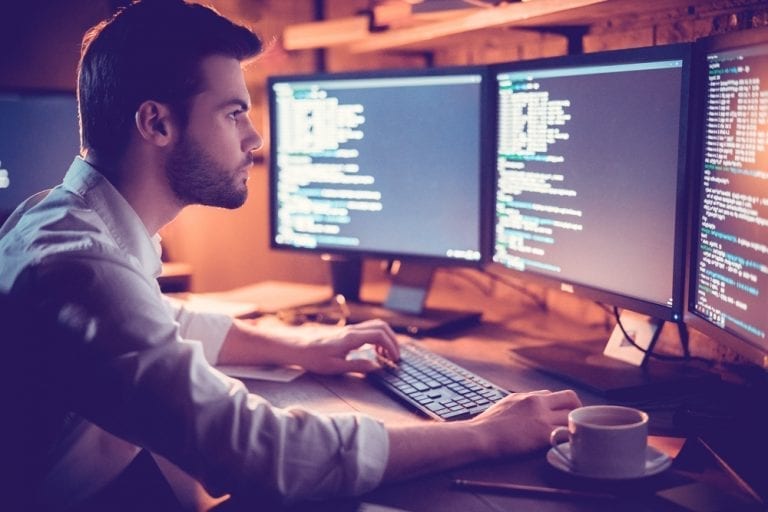
Executives at large enterprises have understood the value of data and data quality for years, but that awareness has been pervading an ever-broader spectrum of organizations. As the volume and velocity of data available has skyrocketed, leaders in business and public sector entities alike have realized that their accumulated data holds great potential as a strategic asset.
Just as physical assets such as machinery, equipment, and real estate require maintenance and oversight, so too do an organization’s data assets. As we engage in discussions about the value of corporate data, we commonly hear the phrases “data governance” and “data quality” used interchangeably. In fact, these are two very distinct disciplines, but they are closely interrelated in a kind of symbiotic relationship where each one feeds off of the other.
Data quality defined
Data quality ensures that critical information is accurate, complete, and consistent across multiple domains within the enterprise. Accuracy suffers for a number of reasons. When information changes, such as when a customer moves to a new address or changes their legal name, existing company data may be rendered inaccurate simply because it is out of date. This is especially common in the case of customer data, which as a whole grows less accurate over time.
Accuracy may also be the result of errors in the data entry process, or the acquisition of poor quality data from an external source.
Poor data quality may also arise from information that is incomplete. Again, this might arise from an oversight during data entry, or it can occur when new data elements are added and no provision has been made for updating existing records. Finally, data may be incomplete if integration processes are poorly designed.
Read our eBook
Fueling Enterprise Data Governance with Data Quality
Learn how data quality is strengthening the overall enterprise data governance framework.
Consistency of information across multiple databases within an organization can also be a source of quality problems. Mismatched addresses in CRM vs. ERP, for example, may result in shipments being sent to the wrong location. Duplicate and obsolete records are extraordinarily common. They cost enterprises millions of dollars every year, for example, wasting money to reach out to the same customers multiple times, or marketing to businesses that no longer exist.
An even bigger problem is the manner in which poor data quality can distort the insights derived from advanced analytics. We worked with one large company at which over 90% of customers were flagged in the CRM system as being in the aerospace industry. Why? Because “industry” was a required field, and “aerospace” was the first item in the drop-down list of possible values. Busy salespeople, eager to minimize the time spent entering customer information in CRM, simply took the path of least resistance.
Imagine how that kind of anomaly might distort your company’s analytics. What if this irregularity had been harder to detect? Poor data quality can lead to a “garbage in, garbage out” scenario in which important business decisions are driven by information that is simply wrong.
Data governance defined
Data governance is closely related to, but in most respects fundamentally different from data quality. Governance provides a framework from which companies can manage data within their organizations, ensure its security, maintain compliance with important government regulations, and make sure it is available to the people who need it, when they need it. Data governance must account for the location and content of data assets, the integration points and business rules that govern data exchange between disparate systems, regulatory constraints, security and privacy vulnerabilities, and more.
Ultimately, data governance is about making sure that the organization is able to maximize the value it gets from its data while controlling security and regulatory risks.
How are data governance and data quality related?
Data governance and data quality exist in a symbiotic relationship. Each one is essential to the other, and organizations that intend to extract meaningful value from their data assets must be mindful of both. Both require ongoing efforts; neither is a “one and done” proposition.
In some respects, data quality almost functions as a component of data governance. After all, it is hard to imagine effective data governance without addressing data quality. The reverse is also true, though. It is difficult to achieve meaningful levels of data quality without an effective governance framework in place. This is especially true at scale. In smaller, more focused scenarios, we can imagine an effort to improve quality in the context of a well-defined domain. Efforts to clean up the CRM database, for example, might be performed in relative isolation. However, in order to really do the job effectively, data governance and data quality must be seen as two sides of the same coin. This is especially true where larger volumes of data and multiple software systems are involved.
Keys to success
If your organization is just getting started on data governance and data quality initiatives, adhering to the following steps will help to make your initiative successful. For organizations trying to improve existing initiatives, these steps apply as well.
- Be willing to start small and grow. As with many other endeavors, there is virtue in starting with a limited-scope project and learning from your early efforts. It is important to prioritize the data sources and integration points that are most important to the organization. By showing success with a smaller project at first, you can demonstrate the business value of data quality and governance initiatives, gain additional support throughout the organization, and prepare for your next success.
- Collaborate. Not everyone in the organization will understand the value of data quality and data governance initiatives right away. That doesn’t mean you should shy away from including them in the process when you can. By incorporating different perspectives and priorities, you will be better positioned to build momentum going forward.
- Measure your results. Data quality and data governance produce tangible business value. Seek out your colleagues in other departments, understand their priorities and pain points, and develop your data initiatives around opportunities to generate business value. Your plan should specify how you will measure your results so that you can build upon your efforts with the next iteration.
Ultimately, data quality and data governance work together to ensure that the information available to stakeholders throughout the organization is trustworthy, secure, and available when it’s needed. By building a meaningful strategy around these two pillars, business leaders can ensure that their organizations are well positioned to optimize the value of their data assets.
To learn more about how data quality is strengthening the overall enterprise data governance framework, read Fueling Enterprise Data Governance with Data Quality.