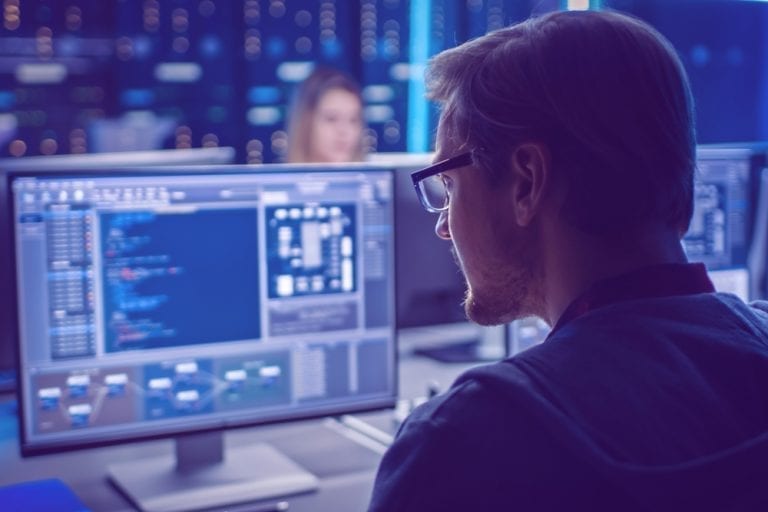
5 Reasons Data Quality Initiatives Fail
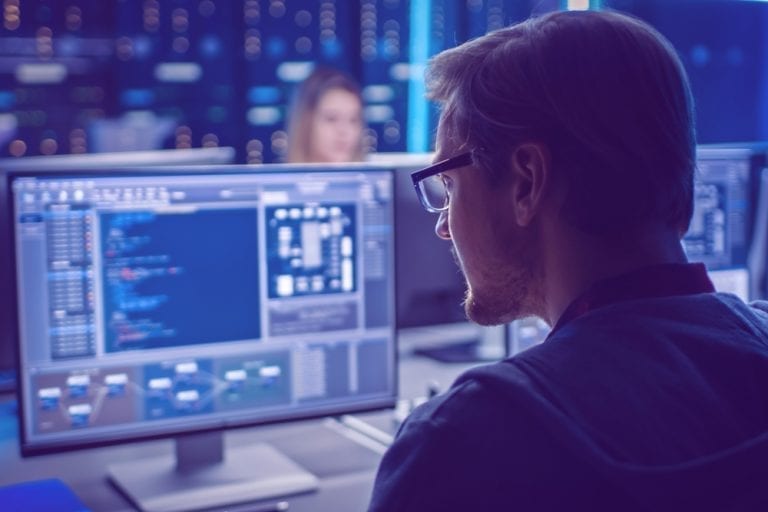
As more and more enterprises recognize the value of data analytics and data-driven decisions, the challenges associated with poor data quality have been brought to the forefront. As business leaders seek to leverage data for strategic advantage, the cost of getting it wrong is higher than ever before.
Poor data quality has a direct negative impact on the bottom line. For frontline employees, it can result in time wasted tracking down and resolving errors. The business may waste money on shipments sent to the wrong addresses or on marketing their products and services to the wrong audience. Poor data quality can lead to a bad consumer experience, resulting in customer defections and lost revenue.
Even bigger problems arise, however, when poor quality data is used to inform business decisions. As the power of big data analytics has increased and as the volume of available information has skyrocketed, the potential for data-driven approaches in management has risen tremendously. Poor data quality can lead to bad business decisions.
Unfortunately, many data quality initiatives fail to achieve their intended results. For these programs to succeed, they require a clear vision that aligns with business objectives, adequate buy-in from stakeholders throughout the organization, and a realistic roadmap designed to achieve specific measurable outcomes. Let’s explore some of the potential pitfalls.
1. Lack of clear objectives
The first key to successful data quality initiatives is to clearly define the desired outcome. It is not enough to just say “we need to improve the quality of our data.” There must be a well-defined problem (or problems), including an understanding of its current consequences. Project leaders should establish objective measures of existing data quality and tie those measures to costs (or lost revenues) that negatively impact the bottom line. They must also lay out a vision of the desired end-state and define the criteria against which success will be measured. Most experts recommend starting small, with a limited-scope objective that can be achieved in the short term. Pick your top priority, and then clearly outline the current state of data quality, the economic benefits of improving it, and the criteria for measuring success.
Read our eBook
Fueling Enterprise Data Governance with Data Quality
To learn more about how data quality is strengthening the overall enterprise data governance framework, download our ebook.
2. Lack of alignment
Not everyone in your organization will immediately understand the benefits of a data quality initiative. It’s important to clearly articulate the problem, the cost of doing nothing, and your proposed solution. Listen to the concerns expressed by department heads and frontline staff. Probe into potential pain points arising from poor data quality. Ask about some of the common challenges workers face when information is inconsistent, incomplete, or inaccurate. Consider how those problems contribute to lower productivity, negative customer experiences, or wasted expenditures.
By opening up those paths of communication, you can build ground-level support that will pay dividends as you roll out your data quality program. At the same time, though, it is critically important to establish buy-in from upper management. Successful data quality initiatives require an investment of time and resources, ultimately leading to better business results. Senior-level executives need to understand the business case and be supportive of data quality efforts.
3. Resistance to change
Regardless of how well you have communicated the need for improved data quality, in many organizations, there will still be some resistance to new ways of doing business. That may arise from a defensive stance among department heads eager to protect their turf from outside meddling.
Just as often, though, managers may fail to understand the tremendous value of data-driven decision-making in today’s world. This is frequently rooted in concerns that data-driven decisions will somehow supplant human judgment and experience. In reality, the situation is very much the opposite; the most innovative companies in the world are deploying advanced analytics and even artificial intelligence to supplement and extend the value of human knowledge, giving experts a powerful set of tools with which to apply their expertise.
The first step toward addressing this problem is to simply recognize that it exists and be prepared to deal with it. Work with managers throughout the company to understand their concerns and address them head-on. For those people within the organization who are still resistant to change, a top-down mandate from management serves as an important fallback to ensure that you gain the cooperation that is necessary to move forward with your data quality initiative.
4. Lack of focus
For companies with multiple disparate systems, undertaking data quality initiatives can be daunting. When a program aims to achieve too much too quickly, the likelihood of delays or even project failure tends to increase dramatically. To make your data quality initiative successful, start small and focus on a concrete, well-defined objective that can be achieved relatively quickly.
By starting with a small, low-risk project that delivers measurable value, you will strengthen your case for further management commitment to data quality. Success begets success; start with the proverbial “low hanging fruit.”
5. Using the wrong tools
Data analytics have come a long way in the past decade. So, too, have the tools that support data integrity and data management. Modern analytics platforms are increasingly deployed in the cloud. The number and diversity of source systems have grown considerably in most organizations. Compliance issues have introduced additional complexities around issues such as data sovereignty.
To make matters even more complex, modern enterprises remain in a constant state of flux, as new systems are added, existing systems are modernized and migrated to the cloud, and organizational changes impact the IT landscape. This makes it difficult (if not impossible) to predict what your IT landscape might look like in the future. The right data quality and data management tools can grow and adapt as your organization changes.
For most modern organizations, serious data quality initiatives call for enterprise-grade tools. As a global leader in data quality, data enrichment, and location intelligence, Precisely helps organizations to achieve strategic advantage in an increasingly data-driven world.
To learn more about how data quality is strengthening the overall enterprise data governance framework, download our ebook Fueling Enterprise Data Governance with Data Quality.