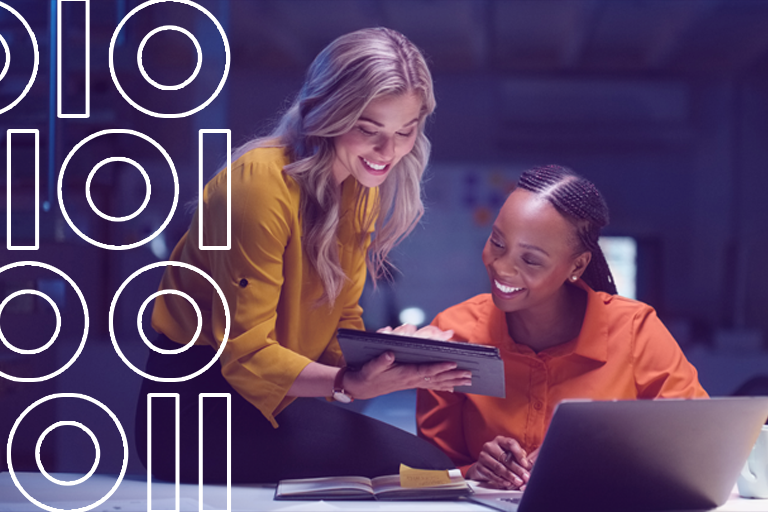
Data Quality Trends for 2024
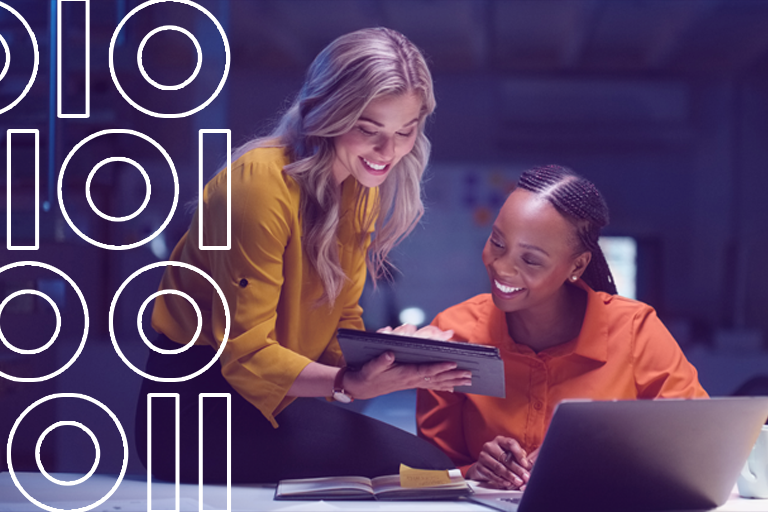
The demand for trusted data is at an all-time high.
This was made resoundingly clear in the 2023 Data Integrity Trends and Insights Report, published in partnership between Precisely and Drexel University’s LeBow College of Business, which surveyed over 450 data and analytics professionals globally.
77% of respondents cited data-driven decision-making as the leading goal of their data programs – but only 46% have “high” or “very high” trust in the data used for decision-making.
70% who struggle to trust their data say data quality is the biggest issue.
Data quality remains top-of-mind for organizations across industries, with the use cases constantly growing.
Many companies are investing heavily in artificial intelligence (AI) and advanced analytics, seeking a strategic advantage over their competition. Data democratization is receiving more attention than ever, and data analytics is becoming a central element in compliance. Data governance is going mainstream as well, prompting companies to focus more attention on managing data quality at scale.
Here are some of the most important trends in data quality for 2024.
1. A Cloud-First Strategy Calls for a Scalable Approach to Data Quality
Cloud-based analytics platforms allow for large-scale computational processing at a level that would have been prohibitively expensive for most enterprises just a few years ago. At the same time, the digitization of business processes, the proliferation of mobile devices, and the advent of practical and affordable IoT sensors generate mountains of new data for analysis.
As the world grows more and more interconnected, the nature of data quality initiatives must adapt to scale. Two decades ago, most organizations might have struggled with a limited number of internal datasets. Duplicate records and decaying data quality – especially in customer databases – were the primary concerns for many.
Today, the volume and variety of data quality challenges have exploded – so much so that it’s simply become too much to manage manually. Data quality must now be approached through a lens of scalability.
The best solutions are augmented data quality tools that provide robust mechanisms for discovering, profiling, and cataloging data; and then building sophisticated business rules to define what good data quality looks like. Using that as a foundation, automation can bring potential data quality issues to the attention of line-of-business data owners to address problems as soon as they emerge. As the amount of data and the uses for it continue to climb, automation is crucial.
2. Data Democratization Hinges on High Data Quality
Data democratization has the potential to help stakeholders throughout an organization make better business decisions, faster.
That’s why there’s such an increased demand for data marketplaces that enable quick self-service access to the trusted data needed for those decisions.
But marketplaces and other democratization efforts come with some risks. Without high data quality, poor decisions could be made with potentially devastating consequences.
According to IDC’s Data Trust Survey, trust in data quality tends to be lowest among front-line employees working in operations, human resources, and sales/marketing. Top executives, in contrast, are typically far more confident in the data they use to make important decisions.
This has important implications for executives aiming to increase data democratization within their organizations. When trust is low, utilization will likewise be diminished. Enterprises simply will not be taking full advantage of the data assets at their disposal, which amounts to a huge missed opportunity.
Proactive, scalable data quality programs offer two very important benefits. First, they increase overall confidence in data integrity throughout the organization. Second, they yield more accurate insights, which lead to better business decisions.
2023 Data Integrity Trends & Insights
Results from a Survey of Data and Analytics Professionals
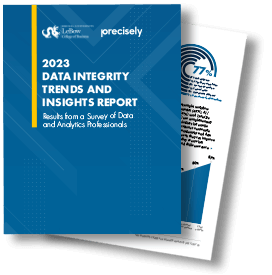
3. Without Data Quality, AI Remains a Huge Liability
Everyone’s talking about AI. In software, AI technology provides a unique ability to automate or accelerate user tasks, which results in greater efficiency and productivity, as well as a reduced dependence on manual labor.
When it comes to generative AI, there’s been an unexpectedly astronomical rise in the usage among everyday consumers over the past year, sparked by the launch of ChatGPT in November 2022.
This transformational technology has the world buzzing, and AI’s potential continues to grow. But what does that mean as it relates to data quality and the bigger picture of data integrity?
AI-powered software capabilities can address a broad set of data integrity needs, providing the agility and decision-making power that organizations need to thrive and compete. That includes providing insights into data quality and how to improve it. Intelligent solutions can provide automatic data quality scores that users can adjust – cutting down the need for data experts to meticulously, manually analyze data.
But AI without high data quality is a huge liability. That’s because data quality is directly linked to data trust. And without trusted data, you risk compromising your AI initiatives (or any advanced analytics efforts) due to unreliable insights and biases that don’t fuel business value.
But with data integrity, and data quality in particular, you reduce risk and liability while gaining more trustworthy and dependable AI results. That means you’re set up for confident data-driven decisions that help you grow the business, move quickly, reduce costs, and manage risk and compliance.
4. Compliance Depends on High-Quality Data
Enterprises are spending increasing amounts of time, energy, and resources to comply with new reporting standards, contractual obligations, and government regulations.
Government regulators, likewise, continue to ratchet up requirements for timely, accurate reporting to meet a host of new mandates. And they’re more frequently asking for data governance and quality measures specifically. At the same time, large customers are demanding that their vendors need increasingly stringent standards, supported by routine reporting of key metrics.
Improved data quality continues to be essential for successful privacy, security, and industry-specific compliance, along with minimized reputational and financial risk.
5. Better Data Quality Relies on a Strong Data Governance Program
Data governance has officially shifted from a “nice to have” feature to a mainstream necessity for any organization wanting to use its data to generate strategic and tactical business value.
A solid data governance program is the foundation of enterprise-scale data quality, providing a framework that aligns people, processes, and technology. Without governance, the quality of data is often misunderstood and mistrusted. The good news is that organizations with a data governance program are seeing improvements in the quality of data analytics and insights (57%) as well as the data itself (60%).
A governance framework is becoming even more critical as business data grows more complex. The digitization of many business processes and digital consumer interactions as well as a wealth of geospatial information and demographic data add up to big opportunities. At the same time, increasing regulatory complexity brings new challenges, requiring that businesses of all sizes understand and control the information entrusted to them.
When data quality is improved within the framework of a solid data governance program, it not only assures data’s accuracy, completeness and relevance, but also tackles data credibility. Data quality tools may include parsing and standardization, cleansing, profiling, and monitoring to improve data quality, but they’re more successful when supported by a solid data governance framework.
These five trends are driving more and more enterprises to find better ways of managing data quality proactively and routinely for high volumes of data. For more on the state of 2024 data quality trends and data integrity at large, read the 2023 Data Integrity Trends and Insights Report.