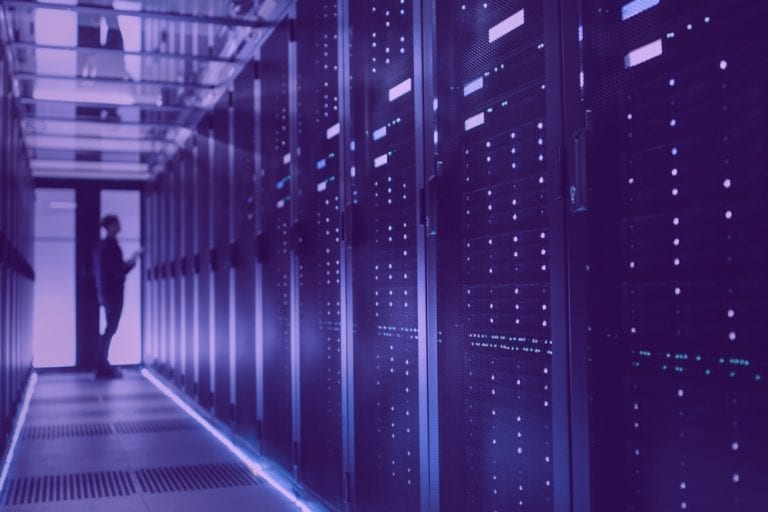
How to Solve the Top 4 Data Integration Challenges of 2021
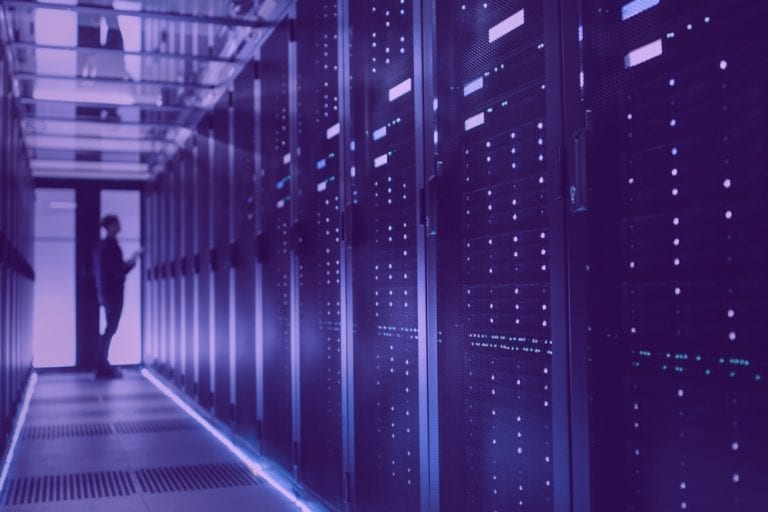
As the volume of data available to large organizations continues to increase, data integration challenges are becoming more difficult. Business leaders clearly understand that their data is a promising source of value, but the volume, velocity, and variety of data available today is daunting. It’s hard to keep up with all of the information flowing from mobile devices, IoT & telematics, clickstream analysis, transactional systems like mainframe, and a flood of unstructured data from social media and other user-generated online content.
If your organization is resolved to gain greater mastery over your data assets in 2021, you’ll need to address some of the core data integration challenges that arise from volume, velocity, and variety. Here are the top four challenges that every business leader should bear in mind.
Challenge #1: The right data is not available in the right place
Organizations are constantly looking at different modes of technology investment whether it is large platforms or best-of-breed solutions. Specialty systems for marketing automation, logistics, inventory planning optimization, and other focused business processes have eclipsed many of the on-premises platforms of the past. Cloud computing, together with the widespread adoption of web services APIs, has made point-to-point integration simpler.
At the same time, the increased number of different systems in use within many organizations has made it more difficult than ever to rein in all of that complexity. Those systems can be highly interdependent. In some cases, transactional integrity rests upon accurate and timely integration of data across those disparate software products. Inventory planning and transportation logistics, for example, must have clear visibility to ERP data, with read/write capability to ensure that customers, suppliers, and internal personnel have access to information that is accurate and up-to-date at all times.
Analytics is playing an increasingly important role, as well. In financial services, timely access to transaction data drives effective fraud detection and prevention programs. Supply chain planners can be more responsive to both external events (such as weather) and internal changes (such as factors affecting production or sourcing) when they have access to that information in real time. In other words, it’s not just about getting the right data available in the right place – it’s also very much about timing.
Solving this data integration challenge is ultimately about having a robust enterprise-grade integration solution – one that is capable of plugging into multiple data sources, including legacy, cloud, and on-premise software systems.
Read our eBook
A Data Integrator’s Guide to Successful Big Data Projects
When it comes to successful big data projects, the reality is your business is relying on you to get it right. To learn how you can get data integration right in 2021, download our free e-book.
Challenge #2: Inconsistent formats and data models
Many of the data integration challenges arise from the differences in data formats and models across various systems. Organizations that run mainframes are acutely aware of this; fixed-length data types, COBOL copybooks, hierarchical databases, and other anachronisms make it especially challenging to pass data back and forth between mainframes and cloud platforms.
Even when integrating data between two or more systems, differences in data models can make integration very problematic. ERP is typically the system of record but must share data with a CRM system that classifies customers very differently and includes lists of leads that range from existing customers to warm leads to window-shoppers with little or no intention to purchase.
Master records are often coded differently in those multiple systems – that is, they have unique identifiers that conform to different alphanumeric formats and must be mapped following a well-defined set of business rules.
Again, a robust enterprise-grade integration solution can address the challenges of mapping and harmonizing data, as well as master data management (MDM). The process should start with a comprehensive data profiling exercise, which will establish a baseline upon which an effective integration strategy can be built.
Challenge #3: Poor data quality
That same data profiling process provides an excellent starting point for gaining control over data quality. Data quality problems typically arise from a variety of different sources, including human error, inconsistencies in the way the information is managed in different systems, previous integration errors, and more. Static data also tends to degrade over time. This is especially true of customer information, which becomes outdated as customers change their names, relocate, merge or go out of business (in the case of commercial customers), or pass away (in the case of individuals).
A comprehensive approach to correcting data quality issues begins with data profiling but also includes putting tools and processes into place that enable line-of-business workers to own and manage data quality effectively and efficiently. In addition to implementing those technical capabilities, it is also important that leaders institute programs that drive awareness throughout the organization as to the quantifiable cost of poor data quality.
Challenge #4: Extracting value from the deluge of data
We referenced the three V’s of data; volume, velocity, and variety earlier. At Precisely, we like to talk about a fourth “V” – value. Where the first three V’s bring challenge and complexity, value is where true competitive advantage is to be found. Our research has shown that more than half of enterprises rely on the effective use of big data for strategic gains, often incorporating location intelligence to enhance specific business processes. Examples include retail site selection, better responsiveness to natural disasters in the insurance industry, or improved performance management for bank branches.
Strategic use of data often includes a 360° view of customers, which drives stronger marketing initiatives, better product development, and higher levels of customer service. Extracting strategic value from your data should include a data enrichment strategy, as well as a location intelligence perspective to add context to your existing data.
Getting data integration right
Precisely is a global leader in data integration, data quality, enrichment, and location intelligence; we offer best-in-class tools and expertise to help companies achieve excellence in enterprise data integration.
Learn how your organization can get data integration right in 2021. Download our free e-book A Data Integrator’s Guide to Successful Big Data Projects.