eBook
A Data Integrator’s Guide to Successful Big Data Projects
This eBook will guide through the ins and outs of building successful big data projects on a solid foundation of data integration. Read more.
Reality Is…the Business Relies on You to Get Big Data Right
Traditionally, three “V’s” have defined big data – volume, velocity, and variety. Volume is the amount of low-density, unstructured data that need to be processed. Depending on the organization, that volume could range from tens of terabytes of data to hundreds of petabytes. Velocity is the rate at which you receive and act on data. Depending on the data type, that could mean ensuring real-time responses to the information. Variety refers to all the different types that make up big data, from structured to semi-structured and unstructured. All these data types need to come together for downstream business use.
However, adding to the complexity of big data is a fourth V – value. Businesses are increasingly relying on big data success to enable strategic gains. And as a result, the capital and revenue benefits that come from well-managed big data efforts become the more critical piece of defining big data. Precisely research has shown that more than half of organizations rely on the effective use of big data for strategic gains.
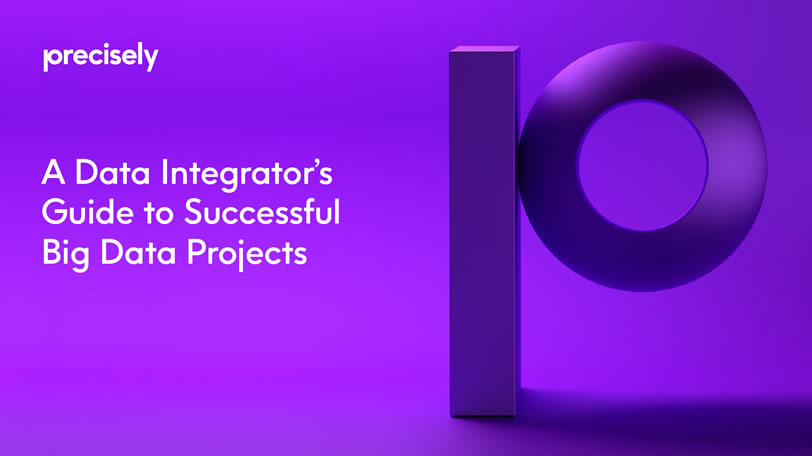
What are the top 3 business initiatives you organization will support in the next 12 months?
Increased operational efficiency
Improve customer experience
Improved access to
data for decision-making
N = 230 IT professionals
Sources: Data Trends for 2019: Extracting Value from Data, Precisely 2019
You simply need to successfully bring together massive amounts of data in a variety of forms and integrate it all in a cohesive way that enables business users to make real-time decisions. Easy right? The reality is that successfully tackling big data is one of the hardest parts of IT’s job. Yet the business relies on you to get this done right, even when it can seem impossible to know where to begin. That is why this eBook is here. Its goal is to help guide you through the ins and outs of building a successful big data projects on a solid foundation of data integration.
Ingredients of a Winning Big Data Strategy
You can’t bake a cake without crucial ingredients like flour, and big data success is much the same. To build a data integration framework that can support big data, you need to begin with a set of ingredients. However, unlike a complicated cake recipe, there are only four ingredients required for big data success.
1. Have a clear business case
Having a clear business case for implementing big data frameworks gives you a “why.” That “why” helps you to understand not just how the data will inform a business strategy, but also helps you give you a focused working goal. It’s essential to work across all stakeholders in an organization when determining your business case.
2. Know what data you need
Understanding the end goal helps to inform the types of data you will need to integrate. Often this requires extracting data from different silos and sources across the organization and bringing it together into a unified data flow using a data integration framework that is repeatable. This enables you to reuse your process for all different kinds of data projects.
3. Understand the data
After establishing points of integration, it’s critical to ensure the data delivered is of quality. As data moves to downstream applications, you must have a strategy for how data will be viewed and understood. The best way to approach this is to take a hard look at the data profiling processes that you have within your organization.
4. Address data lineage and governance
When you are working with data, it is not enough to understand and integrate it. Any big data initiative must also have practices to satisfy regulatory, compliance, and data governance requirements for all data used.
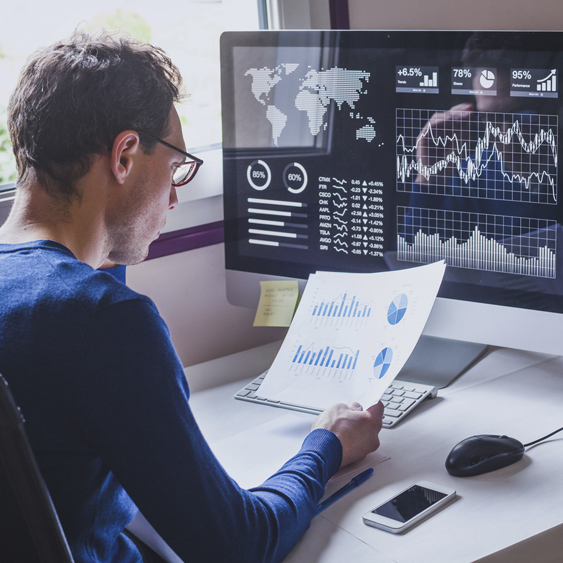
Building a Repeatable Data Integration Approach
A repeatable data integration framework can reduce the time it takes to accomplish the goal of a big data project. Creating a repeatable process is all about what works best for your team and organization. At Precisely, we have found that a flywheel approach is best to build repeatable processes. Flywheels provide a comprehensive way of looking at a process, helping your team to understand how one step affects the other.
A flywheel process for data integration is helpful when you are developing your business case. It helps you understand initial requirements but, most importantly, iterates on the business case. Key components of the business case include knowing the end goals for all stakeholders and defining a data integration model that is flexible enough to respond to any goal changes. Additionally, the more successful the delivery and integration of data, the more demand it drives!
The framework in detail
The framework in detail
Don’t Forget Data Quality!
A repeatable data integration framework is just one piece of a successful big data initiative. Real success with big data projects means you also need to account for data quality. Peeling back the onion on data quality reveals that it is data profiling that plays a critical role in delivering the right data downstream.
Data profiling can come in two different flavors. The first is a broad-brush approach where you point a tool at the data to generate information about the actual content. As a result, you receive data insights in the form of summaries of the data and details of value and pattern frequencies.
The second, tighter approach uses business rules to ensure that data is “fit for use” in its intended operational and decision-making contexts. Checking data against business rules helps to address the accuracy, completeness, consistency, relevance, timeliness, and validity of data.
Regardless of your approach, data profiling helps you use your high-quality data for:
- Decision making – Helping to ensure that there is trust in the data that drives your business.
- Customer centricity – Getting a single, complete, and accurate view of your customer for better sales, marketing, and customer service.
- Compliance and governance – Knowing your data and guaranteeing its accuracy to meet industry and government regulations.
- Accuracy – Making sure that high-quality analytics, machine learning, or AI models are training on high-quality data.
Not sure where to begin with data profiling? Ask yourself these five questions!
Q. How you want to analyze the data?
Q. What should you review?
Q. What should you look for?
Q. When should you build rules?
Q. What needs to be communicated?
Tracking Data Lineage and Governance
Your data integration strategy for big data is only as good as the governance practices that surround it. Implementing data governance helps you achieve a successful business result and meet regulatory compliance requirements. Governance requires a multi-faced approach that includes data quality, security, and lineage. In the context of data integration, data lineage is crucial.
Data lineage is important to governance for several reasons.
Data lineage helps you:
- See linkages to external data sources and targets
- Gain insight into the flow of data across the enterprise
- Trace usage and assess the impact of changes across the data lifecycle
- Diagnose data problems faster
Any solutions that you decide to use for data lineage should supply an end-to-end approach regardless of the data source.
Remember, data lineage has two parts!
Business lineage + technical lineage =
Secure foundation for big data
Business lineage is the who, what, where, why, and how of the business data. Reports on business lineage highlight the transformation and aggregation of data needed by a business user.
Technical lineage shows the flow of physical data through underlying applications, services, and data stores. Technical lineage helps maintain your data architecture.
The Next 90 Days
Now that you have an idea of the foundational elements for building data integration workflows that help you tackle big data, what should you do to get started over the next 90 days?
- Define your business case
- Ensure you understand the right data to integrate
- Look at how you can build a repeatable data integration framework
- Make sure to incorporate data quality and profiling into your plans
- Set up a process for end-to-end data lineage and governance
- Understand how your team can drive data integration today and tomorrow
How Precisely Connect Can Help
The Precisely solution, Connect, helps you with all your data integration needs for big data projects. Connect offers a simple, one solution approach for ETL and data replication. Features of Connect are:
- A visual, design-once-deploy-anywhere approach for building repeatable data integration workflows
- Reliable transfer of data from legacy systems to business applications, even if connectivity fails on either side
- End-to-end data lineage regardless of the data source
- Real-time data integration regardless of physical, virtual or cloud platform, operating system, and type of data storage
- Unrivaled performance for scaling and future-proofing data integration workflows