White Paper
Getting Started Guide: Common applications for Place Intelligence Metrics
Intro to Place Intelligence Metrics
Place Intelligence Metrics, known as PIM, is an aggregated dataset that provides various metrics about visitors to a location, on a weekly basis. Since it is keyed on locations (rather than device_keys like some of our other datasets), it is a much smaller dataset to work with than our Firehose offerings. You can leverage dimension tables (place, chain, category) combined with metrics to analyze visitation patterns, trends, seasonal shifts, and visitor attributes for those locations. Although the dataset is available at the location level, we often recommend filtering for a specific set of locations to enhance visitor scale and ensure more stable insights.
The visitors included in the PIM dataset are already pre-filtered for high-quality visits, eliminating the need to process raw device-level data to uncover insights. However, this dataset does not offer the flexibility to adjust parameters such as score thresholds, visit duration, or movement sources. If needed, we can provide further details on the filtering criteria applied to this dataset.
What sort of places may work better with Place Intelligence Metrics?
Visitor Scale:
- The metrics in PIM are built on a set of visitors to a location, weekly. If this is a small location, with few visitors, metrics will be unstable compared to locations that garner more visitors.
- When analyzing locations with low visitor scale, we recommend aggregating similar locations (by geography, chain, category) together across multiple weeks, and recalculating the metrics for the set of locations overall.
- A quick diagnostic for visitor scale is the “weekly_unique_devices” sub_category in this dataset – when working with spatial_ids that garner fewer than 50 unique devices per week, we recommend combining with nearby locations to understand visit behaviors.
Single Use Locations:
- The metrics in this dataset will reflect visitors to the spatial feature you filter for, not necessarily only the chain you’re filtering for. If there is a spatial feature than spans multiple places in a building/parcel (think a strip mall, shopping center, busy urban block), the visit metrics will not be specific to a single POI connected to that spatial feature.
- We generally recommend using locations that have the is_high_confidence = ‘true’ & is_multiuse = ‘false’ flag in this dataset – see “How Should I Filter Places For My Use Case” section.
- If you’re explicitly looking to understand visit behaviors to places like malls, shopping centers, or other locations that have multiple uses, be cognizant of the various POIs associated with that spatial feature.
Single Story Buildings:
- PlaceIQ scores movement data based on latitude & longitude of the locates and does not account for altitude of the observations. If there a store on the first floor of a skyscraper, there is no differentiation between the devices at the store and the ones which are in the upper floors.
- Analyzing single story buildings means you won’t be analyzing visitors that are there for a mix of reasons, even if the spatial feature is reflective of the store’s footprint.
- This concept also translates for dense urban areas. It will be more difficult to understand visitation habits in dense cities due to foot traffic on the adjacent sidewalks of a store, attached neighboring buildings, and lower movement data accuracy in places with tall buildings.
Read the full white paper to learn more.
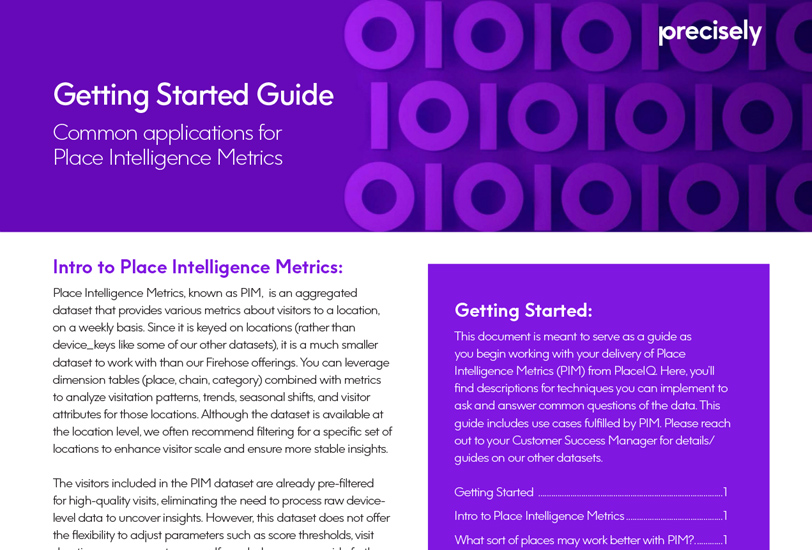