eBook
Trusted Data, Powerful AI: Driving Better AI Outcomes through Data Quality and Governance
Get trusted AI outcomes by ensuring optimal data quality, robust governance, and data observability.
With its capacity to analyze massive datasets and streamline complex processes, Artificial Intelligence (AI) can transform businesses across industries. However, AI’s effectiveness is directly tied to the quality of the data it processes. It’s the ultimate “Garbage in/Garbage out” technology: When AI training models are given quality data, they can make useful decisions. However, if the data is poor quality, if it is inaccurate, outdated, incomplete, inconsistent, irrelevant, biased, or redundant, AI results will be wrong, leading to bad experiences for customers and business partners, delayed action, diminished revenue, higher risk, and higher costs.
Historically, poor data quality has been addressed with a reactive approach; when there’s a problem, someone does a root cause analysis to determine the cause, fixes it, and puts processes or rules in place to stop it from happening again. With AI, the damage is done as soon as the model uses bad data. In other words, putting GenAI on top of poor data will simply give you the wrong answer faster, and the damage will be harder to undo.
When AI models are given quality data, businesses enjoy increased efficiencies, cost savings, improved regulatory compliance, customer engagement and satisfaction, and reduced output bias.
Though AI can significantly improve every aspect of business, only 4% of organizations say their data is AI-ready. Let’s explore the data quality fundamentals you need to verify your data is ready to support your AI initiatives.
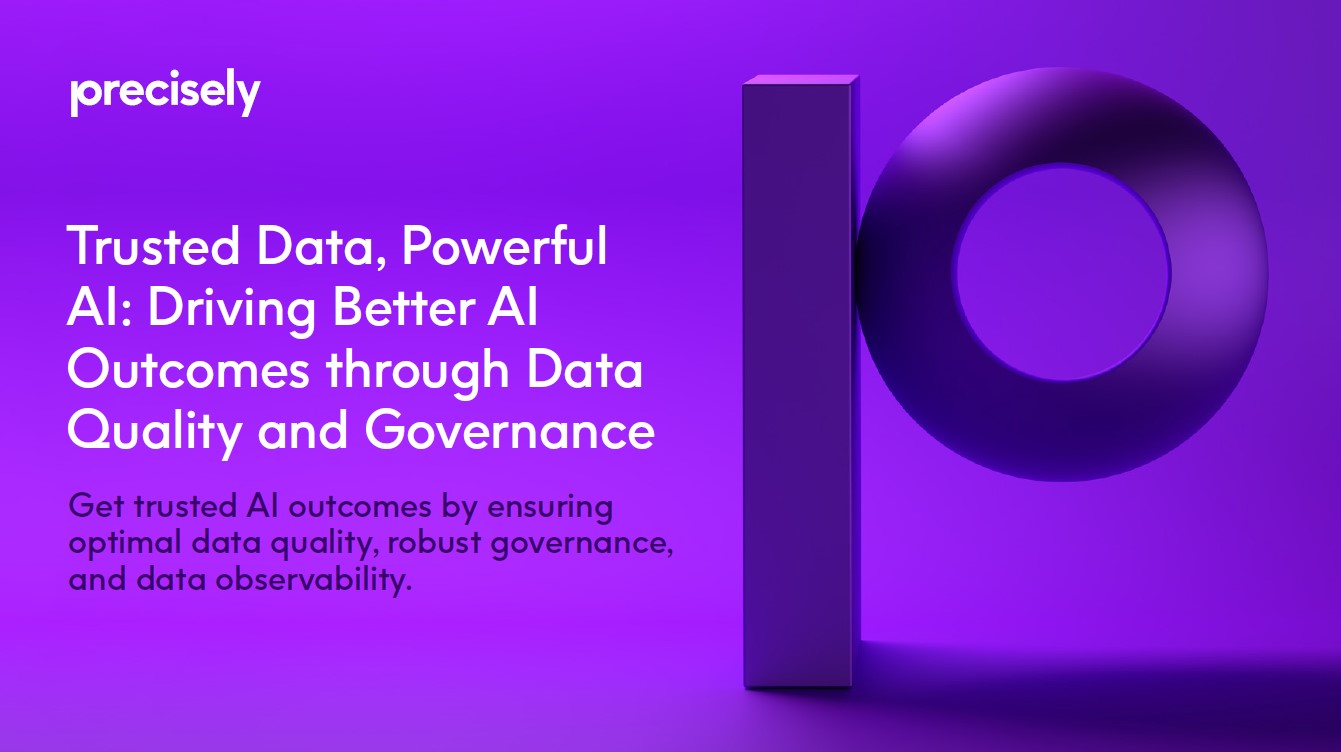
Business Challenge: Untrustworthy AI Results
As businesses look to leverage the power of AI, the importance of using quality data cannot be overstated; without reliable data, advanced AI models are of little use. Inaccurate predictions and recommendations from AI lead to a lack of trust and can potentially prevent further adoption. The stakes are high, and overcoming data quality roadblocks is a priority.
AI initiatives require an innovative approach to data quality to ensure they’re using data that is accurate, consistent, and fit for purpose. This will require proactive core data quality and business rules, automated validation and cleansing, and AI-powered data observations, all with the oversight and benefit of data governance processes.
Data governance for AI
Your company has been collecting and saving valuable data that will contribute to effective and comprehensive AI analytics, reporting, and more. However, you can’t fully realize its value if you don’t know what data you have, where it’s stored, who owns it, or what’s being done with it. And, with expanding data privacy regulations, you may be exposed to noncompliance fines if you can’t identify what policies apply, where your data is, and how it’s being used.
A strong data governance framework is the foundation of a comprehensive data quality solution to ensure trustworthy AI.
Data governance plays a critical role in maintaining the privacy and security of data used in AI. Data must be monitored to ensure with privacy and security regulations related to handling personally identifiable information (PII) data. Data access and usage should also be tracked through data governance to ensure data is used for the intended purposes.
Data governance also ensures your model has all the data it needs and allows you to see where the data is coming from, who is responsible for it, how to access it, and any policies about how you’re allowed to use it.
Data governance answers questions that ensure source data is correct for the intended model. “What does this data set mean?” “Who is responsible for, or owns this data?” and “Is the data I’m using quality data?” A robust data governance framework ensures you can easily find, understand, trust, and leverage critical data across your organization – leading to more accurate and informed AI insights, decisions, and reporting.
Data should also be governed by policies that provide insights into its meaning, lineage, and impact and a clear understanding of how the data you use in AI applications is:
- Collected: what are the data types?
- Stored: where is it located?
- Used: who has access to it?
Data quality for AI
To achieve trustworthy and reliable information from AI systems, it’s vital to have trust in the data used to train your models. As businesses have grown over time, a data quality strategy has usually been an afterthought, if it’s been a thought at all. In the world of AI, harvesting value from data requires a sound data strategy to ensure quality data.
For example, if your business relies on AI to provide customers with personalized experiences and recommendations, such as those that offer movie streaming services, the recommendations will only be as good as the data. If anything is inaccurate, such as age, geography, or language, it could introduce bias and inaccuracies to recommendations.
There are four characteristics which define data quality:
- Accuracy: Is the information correct?
- Completeness: How comprehensive is the information?
- Reliability: Does the information contradict other trusted resources?
- Timeliness: How up to date is the information?
While every business will have unique data quality issues, building sustainable, effective data quality management practices will help you identify the most common sources of poor data quality.
For example, data can be clean, accurate and complete and not be useful. Using data outside specified ranges or markedly different from normal values can lead to false AI conclusions. With a data quality strategy in place, automated controls can validate new and updated data against real-world criteria before being accepted.
Also, data can enter your systems from multiple sources, making it impossible to filter out duplicates. Implementing automated rules and tools for deduplication and entity resolution ensures that critical data sets are accurate, valid, and complete. Duplicates can negatively affect your AI models by creating bias, wasting computational resources, and lead to overall poor model performance.
Finally, when new data is added, data sets can get out of sync, especially when it comes from third parties or streamed from resources such as CRM, credit validation, or trade systems. To ensure AI systems are stable and perform consistently over time, it’s vital to ensure new data conforms to standards and is reconciled with existing data before it’s used.
Data observability for AI
Data observability is a critical aspect of data quality for AI because traditional methods of managing data are no longer sufficient; there’s just too much data to manage manually. Anomalies and inaccuracies can easily happen without anyone noticing, and the longer a data issue goes undetected, the longer AI conclusions will be inaccurate. Data observability eliminates risky and unsustainable manual processes and allows you to proactively ensure AI systems are using quality data to reach conclusions.
Proactive data observability tools can also monitor data pipelines and use advanced AI/ML techniques to quickly identify anomalies and outliers and correct them, so AI models only consume quality data.
For example, if a streaming service is used to getting 10,000 new customer signups a day but suddenly drops to 1,000 or increases to 100,000, there’s an issue that needs to be addressed. Changes to data distribution can happen abruptly or slowly over time, a phenomenon known as “data drift.” Data drift can be much harder to identify but can significantly impact the accuracy of your models.
When handling high volumes of data, maintaining data quality standards is imperative. Data must comply with format rules and be consistent across the data pipeline, and granular data must be continuously checked.
Change within data sets is constant, so capturing data changes quickly and having automated checks is an essential component of data observability. Similarly, unexpected data changes can cause AI to come to false conclusions, especially if they go unnoticed. Data observability automatically detects outliers and unexpected changes and notifies the appropriate users.
As data moves between systems, data that was valid can become inaccurate. Data observability and controls ensure data transformations are performed correctly, business rules are working as they should, and no data is lost or corrupted while data is in motion.
Use Cases
While the potential for AI is expanding every day, let’s explore some use cases with high value returns:
AI Recommendations
The benefits – Faster, more personalized recommendations
How it’s done – An AI recommender system is a sophisticated technology that leverages AI and vast amounts of user data – such as past preferences, behaviors, and interactions – to suggest tailored products, content, or services.
Why data quality is essential
Recommendations will only be useful and relevant if the data is:
- Governed by policies and procedures
- Accurate, complete, and in context
- Consistent and actively observed for changes over time
AI-powered workflow
The benefits – increased productivity, higher efficiency, and lower costs
How it’s done – Workflows can be automated with AI models that process data in real-time. The applications range from automating sales and marketing campaigns and project management workflows to coding assistants where the benefits are pronounced.
Why data quality is essential
Integrated systems often use multiple data sources, so you must be able to combine critical data from all relevant sources, including complex transaction systems. Implementing data quality, data governance, and data observability practices increases the efficiency and reliability of your AI-powered workflows to expedite and track the next best actions.
Machine learning applications
The benefits – Accelerated business processes with greater accuracy
How it’s done – Machine Learning (ML) applications enable computers to learn from data and make predictions or decisions autonomously by generating fast pricing quotes and delivering greater customer satisfaction – but only when data engineers train models with quality data that is governed and observable. Equally as important is flagging highly sensitive data or proprietary information to ensure data is protected.
Why data quality is essential
To dramatically increase accuracy, data observability is critical because it allows you to proactively uncover data anomalies before they explode into your AI models with inaccurate or data that is not fit for purpose.
Foundation Model Training
The benefits – Natural language processing abilities enable Foundation Models (FMs) to generate content and code, summarize text, analyze sentiment, answer questions, and more.
How it’s done – A Foundation Model (FM) is an ML model pre-trained on large datasets and designed to capture general patterns and features.
However, a significant challenge with FMs is the potential for learned bias. For example, FMs used by global banking organizations to process loan applications for minority-owned or home-based businesses are at risk if the data contains inherent biases that reflect societal prejudices, stereotypes, and disparities.
When FMs generate text or provide responses, they may inadvertently replicate and amplify existing social, gender, or racial biases, leading to discriminatory outputs that exacerbate inequalities in various domains.
Why data quality is essential
To prevent bias, you must train GenAI models on data monitored for current and potential adverse data events governed by data quality processes and rules and ensure data is accurate, complete, and in context.
Chatbots
The benefits – Efficient and personalized assistance that increases user engagement
How it’s done – Chatbots built on large language models (LLMs) can deliver natural, contextually rich responses to user prompts. An LLM is a type of FM trained on vast amounts of textual data to understand and generate human-like language.
A chatbot’s ability to dynamically generate responses based on the ongoing conversation sets them apart, enhancing user engagement across multiple industries and use cases like customer support.
GenAI’s impact on customer service is already being felt. The National Bureau of Economic Research surveyed 5,179 customer support agents and found an average productivity increase of 14% when exposed to AI tools. This number goes up to 34% for novice workers.
Why data quality is essential
High-quality chatbot responses require LLMs trained on high-quality, complete data. Data quality practices are essential to ensure data used by GenAI models is accurate, complete, fit-for-purpose, governed by data rules and policies – especially where personally identifiable information (PII) is concerned, and unchanging over time.
Summary
Data governance, data quality, and data observability play a pivotal role in the success of AI initiatives.
However, AI can only be as effective as the quality of data used in its training models. Poor-quality data can lead to flawed or false AI conclusions and increase data privacy and security risks, while high-quality data can transform every aspect of the business. Providing AI systems with complete, trusted, and well-understood data requires a comprehensive strategy that includes data governance, data quality, and data observability.
- Data governance establishes confidence in your data by ensuring that AI models have access to all necessary information and that the data is used responsibly in compliance with privacy, security, and other relevant policies.
- Data quality ensures your data is accurate, complete, reliable, and up to date. AI conclusions based on quality data enable data-driven decisions that reduce costs and increase revenue and compliance.
- Data observability eliminates risky and labor-intensive manual processes by alerting you to identify changes in data and anomalies so you can correct them before they’re introduced into an AI system.
Trusted AI starts with quality data. The Precisely Data Integrity Suite can help you ensure the success of your AI initiatives, including making better business decisions based on trusted data. This modular, interoperable suite of services contains everything you need to deliver accurate, consistent, contextual data – wherever and whenever it’s needed. Data with integrity empowers fast, confident decisions that help you add, grow, and retain customers, move quickly, reduce costs, and manage risk and compliance.