eBook
The Rise of Artificial Intelligence for IT Operations
Read this eBook to learn more about how the inclusion of machine learning processes into an analytic platform takes it beyond correlation on key performance indicators and evaluation of IT operations services and makes it truly self-learning.
Introduction
From the early days of IT operations, organizations have grappled with the challenges of understanding how well their infrastructure is performing in support of the business. They have used a plethora of tools to detect, manage and resolve problems that are causing disruption of services.
Some of tools included have been domain-based performance monitors, network monitors, application monitors, end-user response time measurement facilities, and more. However, with all these tools, comes a fundamental problem facing organizations – how to bring together information from the various platforms in the organization and correlate that information so that business services can be accurately monitored and managed.
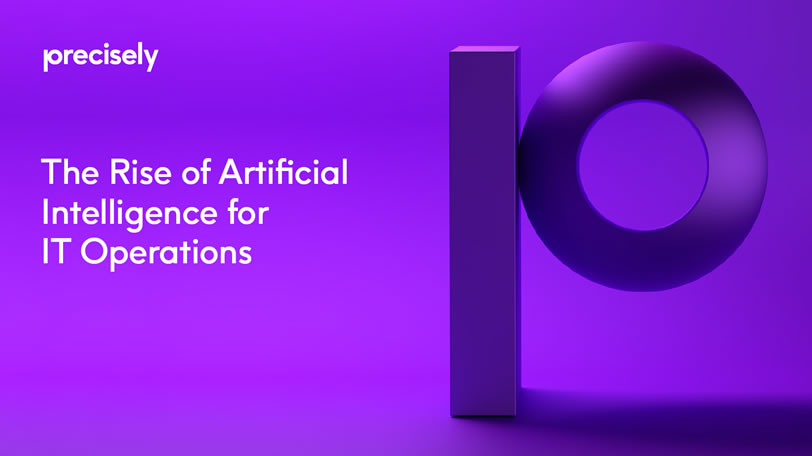
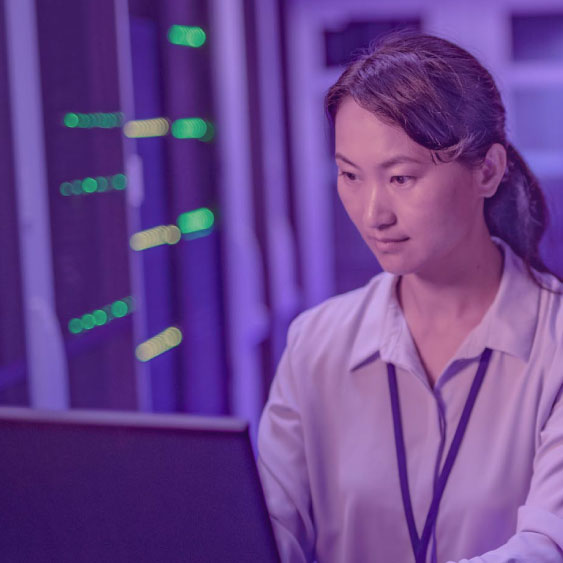
The unfulfilled promise of BSM
For nearly two decades, software vendors and IT organizations were focused on the concept of Business Service Management (BSM). BSM was supposed to map IT components to the business services they supported and utilize dashboards to show how well those business services were running. Yet the promise of Business Service Management was never fulfilled because it was too ambitious for its time, too complex in its make-up, and suffered from deficient underlying technologies. In most vendor provided BSM solutions it was too hard to create and update service definitions, too rigid in how service data was collected and managed, and they relied on too few data sources for actionable business insight. The final nail in the BSM coffin was the fact that they were based on distributed system platforms resulting in one of biggest short-comings – the weak integration between metrics from distributed platforms with Traditional IBM systems within IT infrastructures.
This unfulfilled promise of BSM means that domain-specific monitoring tools still in use today do not present a unified, cross-domain understanding of performance.
The emergence of big data analytics
The good news is that emerging analytics platforms are laying the foundation for innovation and fundamentally changing the approach IT organizations take to managing their infrastructure. Over the past few years analytics platforms like Splunk, Elastic, and others have emerged to address requirements around IT Operations Analytics (ITOA). Their goal being to provide a multi-platform, cross-discipline, integrated view of what is happening across the IT infrastructure and help organizations to eliminate their dependency on those domain specific tools with little or no integration capabilities.
IT Operations Analytics (ITOA):
According to Gartner Group, ITOA is a market for solutions that bring advanced analytical techniques to IT operations management use cases and data. ITOA solutions collect, store, analyze, and visualize IT operations data from other applications and IT operations management (ITOM) tools, enabling IT operations teams to perform faster root cause analysis, triage, and problem resolution.
Say hello to Artificial Intelligence Ops (AIOps)
In just the past couple of years, Artificial Intelligence Operations (AIOps) has emerged from the ITOA discipline by marrying together analytics with machine learning capabilities. As the technology and discipline has become more sophisticated, Gartner has redefined ITOA as “AIOps,” initially calling it Algorithmic IT Ops, and now morphing into “Artificial Intelligence Ops”. This name reflects the increasing use of machine learning, predictive analytics, and artificial intelligence in these solutions.
Machine learning has come to be known as a discipline within computer science that uses statistical techniques to give computer systems the ability to “learn” with data, without being explicitly programmed.
Essentially it is an exercise in learning about and from the data to make data-driven decisions. Machine learning can be used to quickly create, deploy, and continuously monitor a high volume of analytic models to make better use of data and drive better outcomes. It is the “artificial intelligence” that when combined with ITOA provides an AIOps platform.
AIOps uses machine learning and advanced analytics to simplify operations, prioritize problem resolution, and align IT with the business. Using metrics and performance indicators that are aligned with strategic goals and objectives, it goes beyond reactive and ad hoc troubleshooting to proactively organize and correlate relevant metrics and events according to the business services they support.
Artificial Intelligence for IT Operations:
Platforms that combine big data and machine learning functionality to enhance and potentially replace a broad range of IT Operations processes and tasks – including monitoring, event correlation and analysis, service management, and automation.
This allows maturing IT organizations to quickly get a deep understanding of the impact that service degradation has, not only on the components in their service stack, but also on service levels and business capabilities. It provides a business versus a technology perspective. For example, IT can understand “How is the web store performing?” instead of checking the status of the web-server and application.
In the research note “Deliver Cross-Domain Analysis and Visibility With AIOps and Digital Experience Monitoring”, Published 5 July 2018 (ID: G00352799) – Gartner Group predicts that by 2023, 30% of large enterprises will be using artificial intelligence for IT operations (AIOps).
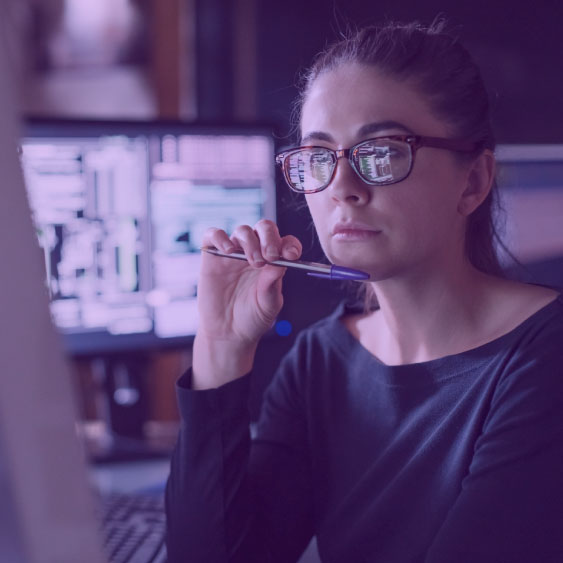
Analytics platform – A critical component of AIOps
Analytics platforms like Splunk, Elastic, and others are laying the foundation for innovation and fundamentally changing the approach IT organizations take to managing their infrastructure. They are in many ways, the essential backbone of an effective AIOps strategy, providing the capability to capture data from a variety of platforms and sources, and normalize the data so it can be effectively correlated and easily visualized. Furthermore, these platforms typically include advanced search capabilities, extensive analysis, and in-depth visualization including basic reporting, event monitoring, and comprehensive dashboards.
Analytics platforms have emerged to address many of the requirements IT organizations have dealt with in the past using different platform tools. Rather than have domain specific tools that operate independently, without integration, analytics platforms are being used to address the following requirements:
- Network performance monitoring
- IT infrastructure monitoring
- Application performance monitoring
- End-user experience monitoring; this is response time monitoring and is sometimes referred to as Digital Experience Monitoring (DEM)
- Service Level Management (SLA) monitoring
Machine learning – Completing the AIOps solution
Using big data analytics, an organization can monitor and correlate all their IT performance indicators, including those in the traditional IBM environments, for a comprehensive view of service delivery. What is still missing however, is being able to detect anomalies and understand patterns in the behavior of the IT components and applications and use that information to predict future behavior. This is why machine learning technologies for pattern discovery, along with predictive capabilities, are being subsumed into the major analytics platforms to provide a complete AIOps solution.
The inclusion of machine learning processes into an analytics platform takes it beyond correlation of key performance indicators and evaluation of IT services, and makes it truly self-learning. With machine learning, predictive analytics can help to effectively set Service Level Agreements (SLAs), identify when potential problems might occur, and help to plan for needed changes in the IT capacity or environment.
3 key properties of AIOps
- Ingest infrastructure and application availability, performance, and event data of different types across all IT platforms and components
- Analyze the data collected using statistics methods and provide visualization through reports and dashboards
- Apply machine learning algorithms to collected data to discover patterns, detect anomalies, understand relationships, and predict future behavior
Addressing the gap with traditional IBM systems
With so much mission-critical data coming from mainframe and IBM i platforms, it is surprising to find that the new analytics platforms have for the most part ignored them or have pushed the responsibility of gathering those data sources onto other vendors to supply.
With the IBM systems serving as the back-end for many mission-critical applications by providing high-volume transaction processing and database services, it must be treated as a first-class citizen in the service environment alongside cloud, mobile, web, and other “newer” technologies. Therefore today’s analytics platforms must ingest data from traditional IBM systems and combine them with data sources from other IT platforms to provide cohesive insights into the activity, status, and performance of cross-enterprise services.
For the most part, this will require special technology from partner vendors that understand the traditional IBM system data sources and can map, normalize, and transform the data into a format which is easily ingested and understood by analytics platforms
Conclusion
Today’s computing environments are a complex arrangement of many hardware components and several software layers, and it is vital for each of these parts to be functioning well. Over the past several decades, this complexity coupled with the variety of platforms has created some interesting challenges for IT organizations attempting to monitor delivery of services to their customers. This resulted in many different and unintegrated domain-specific tools invading the IT infrastructure. Software vendors attempted to address this issue with Business Service Management (BSM) solutions. However, BSM solutions simply could not meet the requirements of IT and most of them failed for a variety of reasons – one of which was their inability to integrate IBM systems data effectively.
Monitoring and analyzing the IT environment remains critical to IT and has resulted in the emergence of the discipline called IT operations analytics (ITOA). For many organizations, ITOA strategies are including big data analytics platforms to help handle data sources from different platforms. These analytics solutions are enabling correlation across platforms and common visualization using state-of-the-art dashboard capabilities. The next step for these technologies is to go beyond correlation and visualization and extend analysis capabilities to include machine learning and predictive analytics – in essence adding artificial intelligence (AI) resulting in the emergence of Artificial Intelligence for IT Operations, or more simply AIOps
In the research note “Deliver Cross-Domain Analysis and Visibility With AIOps and Digital Experience Monitoring”, Published 5 July 2018 (ID: G00352799) – Gartner Group predicts that by 2023, AIOps platforms will become the prime tool for analysis of monitoring data and today’s domain-specific monitoring tools will feed their important data into AIOps for consolidated, higher-level analysis.
With big data analytics, an organization can monitor and correlate all their IT performance indicators, including those in the traditional IBM system environment, for a comprehensive view of service delivery. The inclusion of machine learning processes into an analytics platform takes it beyond correlation of key performance indicators and evaluation of IT services and makes it truly self-learning. This is the promise of AI Ops-analysis, machine learning, and prediction across the entire IT infrastructure.