eBook
Data Governance: How to Get Started
Do you speak data governance?
With varying definitions of data governance, it’s critical that your entire organization is on the same page
Have you ever met someone, perhaps at work, but didn’t catch their name or weren’t formally introduced during your first encounter? Since then, you exchanged pleasantries on numerous occasions, perhaps engaged in entire conversations—but still didn’t know their name?
By that point, enough time had passed and so, too, the opportunity to ask their name without it being awkward at best, and insulting at worst.
That’s actually how many people feel about data governance. It seems that everyone is talking about it, and its importance, but there are many among us who feel like we missed some vital introduction. We think we should know exactly what data governance is, but we missed our opportunity to ask, and now it seems almost embarrassing to inquire at this late date.
But the truth is, formalized data governance is really in its infancy, and you’ll find that the people who don’t quite understand it comprise the majority. Even among those who say they understand exactly what data governance is, definitions will vary widely. Industries, lines of business, job function—all of these inform and influence how we define data governance, and what it means to us. But the important thing is not to establish a universal, formal definition, but rather to understand the fundamentals of what data governance entails, what it is intended to accomplish and how it can serve increasingly important functions in an era of big data. So let’s dig into this topic and try to understand what everyone seems to be talking about.
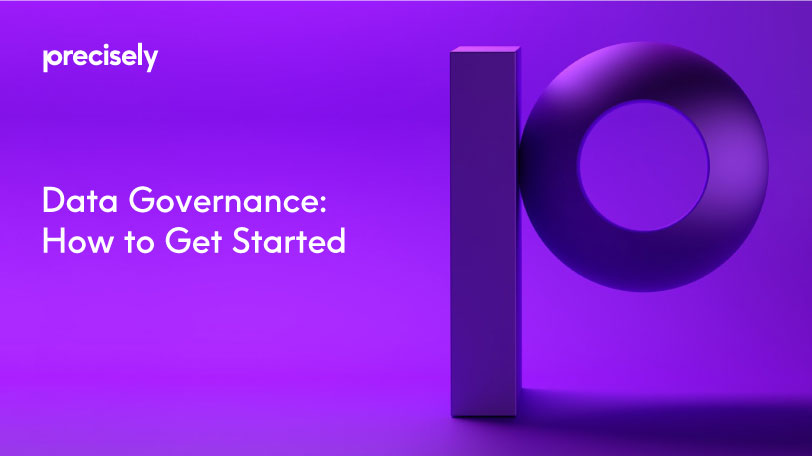
The Evolution of Data
We’re certainly not going to dig into a comprehensive history of data. But a basic understanding of data governance today requires historic context of how data has evolved over time. In the early days, data was largely a transactional concern. The use or production of data was process-centric, applied or generated from business processing activities and limited to a select few.
But over time, the realization gradually dawned that data had real potential beyond the realm of IT and data processing. Organizations began to consider ways that data could be elevated from byproduct to business asset through data analysis for decision-making, an evolutionary step that marked the birth of what is commonly called Business Intelligence (BI). Since that time, the use cases for BI have grown exponentially, and technological advancements have enabled increasingly sophisticated mining of data for business insights. For a number of years, though, it seemed that only the largest companies with the deepest pockets were in a position to reap the full benefits of BI and data analytics, but those days are over. Big data no longer pertains only to big business, as diverse organizations of varying size can collect data at a dizzying pace—yet the value of data lies not in volume, but in an organization’s ability to quickly leverage that data for business advantage. And in an increasingly complex regulatory landscape, the compliance risks can be steep if data and processes aren’t properly managed.
The Emergence of Data Governance
Data today represents a critical asset, and the need to extract value from those assets has moved from a business advantage to a competitive imperative. Its broad array of use cases now require business professionals to find and manipulate data to quickly perform analytics to solve business problems. But to realize data’s full potential, it must be managed like any other asset before it turns into a liability. You need to know where it came from, how old it is, what’s the quality of it, where to find it and how to use it appropriately. Take for example a 3rd party or licensed data set. How do you know if you are authorized to use it in your data analysis? How do you know you can trust it? You don’t unless governance policies and data owners clearly state the scope of its use and metrics to understand its data quality.
The answers to all of these questions comprise the foundation of data governance in business. It requires a repository of these answers, as well as the people and systems that govern data across an enterprise. Simply defined, it is the formal orchestration of people, processes and technology that enables an organization to leverage data as an enterprise asset. Sounds easy, but organizing data governance on a spreadsheet or in SharePoint will only get you so far.
The Building Blocks of Data Governance
Depending on the organizational role, one’s viewpoint of data governance can be quite narrow. For instance, a compliance professional will understandably view data governance through the lens of potential regulatory violations. For example, in the banking industry, BCBS 239 informs many strategies when it comes to data management, but beyond that, banking data also offers a wealth of analytics opportunities for improved customer experience and competitive differentiation beyond the compliance arena. For this reason, data must be properly catalogued, scored and defined so that users across an organizational enterprise can view available assets, understand what they are and how to use them and have a reliable barometer to gauge the caliber of that data to produce quality business decisions.
A data governance program needs to begin with the basics of data governance, such as data lineage (defined as the lifecycle of data from origin over time and through systems and processes); a data dictionary (a description of each data object within a database, its type and its relationship to other data); and a business glossary (the definitions of business terms and how they may vary across business functions). Take data lineage for example. It is of utmost importance to IT professionals, but its information overload for business professionals that require it to be translated into a business lineage which is a key capability of data governance. Beyond these key components, data governance must also define policies, ownership and data quality across an enterprise. But arguably most important to data governance, or at least the key to enabling accurate, meaningful predictive analytics that turn raw data assets into real business value, is another oft-misunderstood buzzword: metadata management.
Where Does Metadata Management Fit In?
If data governance maps the ecosystem of how data flows and functions across an organization’s data supply chain, metadata (often referred to as “data about data”) management provides the underpinnings for understanding that data at a granular level and therefore effectively using it across an enterprise. We are dependent on metadata everyday and don’t even know it. Jump in your car, turn on the radio or plug in your smartphone and metadata automatically shows you the name, artist and duration of the song you’re playing.
To transform data governance you need the ability to connect the metadata to the data governance business glossary (aka data catalog), in order to create a rich understanding of the data beyond its data definition and data quality to include pertinent metadata information. For example, without this fusion of metadata with the data catalog, you won’t be able to connect the dots to do things like translate technical data lineage into easy-to-understand business lineage.
Data, as mentioned previously, can be a tremendous asset, but if business users don’t understand what it is, where it is or how to use it through clearly defined policies and processes, it may as well not exist. But managing metadata in real time as part of a comprehensive data governance framework enables users to easily understand and utilize that data to run analytics and uncover actionable insights. Misunderstanding, on the other hand, breeds mistrust and misuse, leading to questionable results or underutilization.
Implementing a Data Governance Strategy
Clearly there are many moving parts to constructing a successful data governance framework, but building a solution step-by-step maximizes the value of data assets and creates a successful synergy of people, process, and technology. The best data governance program not only maximizes the value of analytic insights, but also ensures the ongoing quality of your data through machine learning, enhanced efficiency and asset utilization through understanding and transparency and increased collaboration across your enterprise through clearly defined responsibilities and workflows. Data governance is dependent on a supporting framework of systems and processes, to be sure, but it is equally reliant on data owners, stewards and the business users who turn that data into value.
So start asking yourself some simple questions like, “Can a broad set of users provide the same answers to what is the definition of the data on this report?” Or, “Who is the data owner and what is the quality of the data?” More often than not, these answers differ depending on who you ask, which is a symptom that one’s data governance isn’t functioning properly.
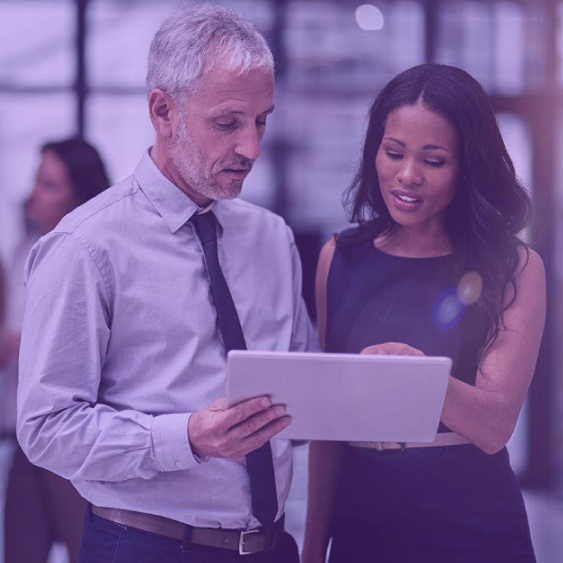
Data Governance: When Is The Right Time To Start?
5 key tips to help identify a starting point for data governance
Data is widely accepted as one of the most valuable assets in an organization. Understanding how data is used, preserving data integrity and maintaining consistency in usage are crucial to the business. Data governance serves as a vital function within an organization by defining guidelines for metadata management, driving processes for data issue resolution and actively measuring data quality improvement over time. An effective data governance program enables business users to make decisions based on transparent and trustworthy data.
Starting a data governance program in a single line of business isn’t easy, let alone across an enterprise. Organizations often struggle to initiate a program due to lack of time, lack of sponsorship or competing budget priorities. One particularly difficult challenge can be the creation of a business case with quantifiable, “hard dollar” benefits. Unless faced with regulatory compliance requirements, the major benefits are often confidence in the data because of common definitions, the ability to track data usage and the ability to trust the quality.
If you find yourself struggling to initiate a program, or build a business case, or determine if a data governance program is right for you, consider the scenarios below:
-
- Centralize and Cleanse Data: If your organization is trying to centralize all their data by building an enterprise data warehouse, a data lake, data hub, enterprise service bus, data transformation layer or a data mart, then you should start a data governance program in parallel. During an enterprise data warehouse initiative or the like, the organization spends an exorbitant amount of time defining what the data means, where it comes from and what kind of transformation it needs to go through before mapping it to the warehouse. Typically, the initiative concludes with a rich array of metadata and governance requirements that quickly grow stale because they are not tracked and governed simultaneously. As a result, a query from a business user about the source of a field in the enterprise data warehouse requires IT to manually trace the element through layers of logic. This same information, the metadata, can be captured and curated while the enterprise data warehouse is under construction.
-
- Address Regulatory Mandates: Many organizations are just beginning to come to grips with personal data capture and use. New legislation, such as the General Data Protection Regulation(GDPR), requires a sophisticated level of monitoring and policing for data. With GDPR effective May 2018, organizations need to identify Personally Identifiable Information (PII) and track where it resides, who has access, where it is sent, and so much more. Similarly, the deadline for the Markets in Financial Instruments Directive (MIFID) II’s transaction reporting obligations is now in effect. Organizations need to understand what they must report, who owns the responsibility for the reports and where they can find the information. Satisfying regulatory mandates is an opportune time to leverage data governance and the business case is clear.
-
- Inefficient Approach to Fixing Data Quality: If your organization’s data management team spends more time fixing data issues than extracting analytics to improve data quality over time, a data governance program is needed. The goal of an effective data management team should be to identify trends in data issues and implement permanent solutions. If constantly researching questions like “Where does this field come from?”, “Why is my data wrong?”, “Who should I contact for this issue?”, or “Does this field exist?” then the data management team has little time to achieve their goal. A data governance program can create the policies, common definitions, data lineage and a shared glossary to provide answers for the users while reducing the number of data issues getting introduced into the environment. The data governance program is how the data management team gets ahead of the data issue volume and systematically injects organization, consistency and accountability into the organization.
-
- Explaining Metadata – One Person at a Time: When valuable time and resources are wasted because your IT team must constantly explain what they mean to the business team, or vice versa, it’s time to initiate a data governance program. Building a business glossary allows an organization to be more productive during cross-team discussions. By defining common terms across the organization, varying teams can communicate easier and analysis from differing organizations is based on the same understanding of the data.
- People as a Single Point of Failure: When your project or business processes slow down significantly because certain members of the team are out of the office, or worse, quit, then it’s a good time to explore how data governance can respond to this challenge. If you panic at the thought of losing a team member because of the institutional knowledge that s/he possesses then that person is the single point of failure. Getting that critical knowledge into a data governance tool can help ensure that others have access to information about where data originates, where it resides, and who has access. Data governance can prevent valuable intellectual property from walking out the door.
So, when is the right time to initiate a data governance program? It’s always a good time to get control of the data, get the enterprise using common definitions, and get data quality measurement underway.
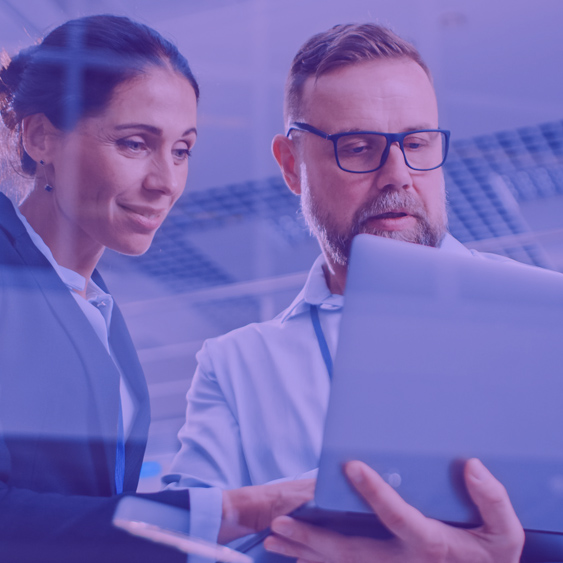
Data Governance: Why You Can’t Afford To Hesitate
Learn How Implementing a Big Data/Data Lake Can Help your Organization Draw Good Data and Make Better Decisions.
We live in a data-driven world. Every business, regardless of industry, relies on data for day-to-day operations. Data creates the levers that organizations pull to optimize the customer experience, to cement customer loyalty, to enable digital initiatives, to make informed business decisions and ultimately to increase profits. For these data levers to have impact, it’s critical to understand the sources of your data, how the data has been transcribed or transformed, who has access to that data and much, much more. Data-driven strategies and decisions require trust in your data quality, accuracy, completeness and relevance. This trust stems from properly governed data.
In the previous chapter, we discussed when organizations should initiate a data governance program. In this chapter we’ll touch on why organizations cannot afford to postpone implementing a data governance program. Given the criticality of properly governed data, it may seem hard to understand why any organization would postpone in the first place.
Why Organizations Hesitate to Achieve Properly Governed Data
As with any major initiative, initial questions often focus on cost and time to value. It might seem like an enormous task to work with stakeholders across an entire organization to get control of the organization’s data. However, hesitation only defers the value of properly governed data and lets the challenges grow, ultimately costing the organization more time and money.
Budget: The biggest reason many organizations are hesitant to implement a data governance program is one simple acronym – ROI (Return on Investment). It comes as no surprise when executive management asks “How much will this cost?”, “How long will this take?”, and “How many people do you need?” What is surprising is how many data governance business cases don’t include ROI. ROI can be difficult to measure, but the lack of this critical piece of information can side track budget approval as other projects with more discrete benefits take priority.
Resources: As mentioned above, implementing a data governance program requires collaboration by multiple teams across the entire organization. Having “governed data” means agreement on common definitions of business terms, development of a data glossary, establishment of data lineage and much more. Subject matter experts from a variety of business domains must work together, often in addition to their primary duties. Getting time from these experts can be a daunting task, let alone getting agreement on business term definitions! Fear not. If necessary, data governance can take a grass-roots approach and start small. The vision can be grand, but the implementation of the program can be done in small, prioritized steps.
Securing budget and resources can be challenging in any organization. Lack of either can cause a data governance initiative to slide down the priority list. Don’t shy away from the grand vision. Start small and prove out the value, but most importantly, start!
Why Organizations Can’t Afford to Defer the Data Governance Program
When organizations start collecting data, it may be on a small scale that is manageable. As decisions are made based on data analysis, organizations begin to see the real value in data collection and, as you guessed it, start collecting even more data. As the quantity of data grows, organizations often lose control of how the data is defined, stored, transformed and consumed. Deferring implementation of a data governance program allows the challenges to grow even larger and make an implementation more difficult.
-
- Loss of Competitive Edge: Trustworthy data is derived from an overarching data governance program. Without properly governed data, organizations unknowingly make business decisions based on stale, inaccurate, or flat out wrong data. To stay competitive, organizations need to be data proactive, not data reactive. Data proactivity is about anticipating data needs and staying ahead of those needs. Data reactivity is taking action in response to an event. Without governance, organizations spend time and energy reacting to emergency data issues rather than focusing resources on proactive measures to do more with trusted data.
- Loss of Competitive Edge: Trustworthy data is derived from an overarching data governance program. Without properly governed data, organizations unknowingly make business decisions based on stale, inaccurate, or flat out wrong data. To stay competitive, organizations need to be data proactive, not data reactive. Data proactivity is about anticipating data needs and staying ahead of those needs. Data reactivity is taking action in response to an event. Without governance, organizations spend time and energy reacting to emergency data issues rather than focusing resources on proactive measures to do more with trusted data.
-
- Overwhelmed by Data: It’s an undisputed fact that data grows tremendously every day. Opportunities to leverage new data sources and requirements to capture/report/forget data surface on a regular basis. The complexity of this data landscape continues to increase. Without proper data governance, without proper prioritization and control of data, this complexity becomes a convoluted mess. Data consumers don’t know what data means, where it resides, who owns it or how to properly consume that data. The longer organizations delay, the more time and effort will be spent cleaning up the chaos. Put simply, the longer an organization defers, the harder it is to start.
- Overwhelmed by Data: It’s an undisputed fact that data grows tremendously every day. Opportunities to leverage new data sources and requirements to capture/report/forget data surface on a regular basis. The complexity of this data landscape continues to increase. Without proper data governance, without proper prioritization and control of data, this complexity becomes a convoluted mess. Data consumers don’t know what data means, where it resides, who owns it or how to properly consume that data. The longer organizations delay, the more time and effort will be spent cleaning up the chaos. Put simply, the longer an organization defers, the harder it is to start.
- Increased Risk for Data Errors: Data quality is a critical component of data governance that helps build trust in an organization’s data landscape and decisions. Data governance helps verify the accuracy and completeness of data. When users modify data sets for their own needs or create their own “single source of truth,” data becomes inconsistent across the enterprise. Proper governance can dictate the authorized sources for data sets and help guide users to pull information appropriately. In industries where data errors can lead to regulatory fines or customer dissatisfaction, those mistakes can cripple an organization.
Eventually the need for data transparency and control will emerge within an organization. Data governance can help deliver that transparency and control that is crucial to achieve properly governed data. The data free-for-all, while expedient at the time, has long-term detrimental impacts on an organization’s ability to make informed decisions and provide trustworthy information to interested parties. The earlier an organization commits to a data governance program, the sooner they realize the benefits. Once data is governed, everyone sings from the same songbook and the harmonious outcome can be enjoyed for years to come.
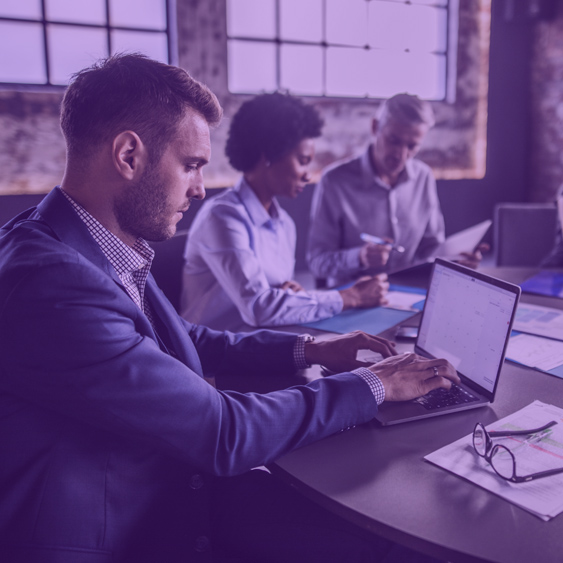
Data Governance: How To Get Started
What you need to know before you begin a data governance program
British mathematician Clive Humby was the first to coin the phrase, “Data is the new oil.” This quote is oft repeated for good reason – data can be an organization’s most valued resource, but it only gains that value after it is refined for use. A key technique for that data refinement is data governance, yet companies often delay data governance initiatives due to competing priorities, lack of budget or challenges in obtaining executive buy-in. However, there is a growing recognition that data governance is a critical piece of an enterprise data strategy. The question organizations are now asking is not why they need data governance, but how to get started.
This post will address practical steps for launching a data governance program, including tips to ensure success. This post assumes that the reader (or their organization) has created a business case to secure sponsorship, funding and resources to at least initiate a pilot program – perhaps even a fully formalized program.
Dipping a Toe
Just as each business is unique, so is each data governance program. Once your organization has established leadership support and funding, the next step is to define a data governance methodology and select the tools needed to support the initiative.
An effective data governance methodology must be repeatable and must include drivers that benefit both the IT and business teams. Seeing the value that data governance can bring to their day-to-day processes will motivate members of the joint team to participate and collaborate.
The methodology you employ depends on your business priorities. Do you need improved data quality to make better business decisions? Is a business glossary your most pressing need to increase enterprise-wide understanding of the data? Do you have a hard time tracking data issues and their status? Is there an urgent need to document lineage to create transparency? The answer may be yes to all of the above, but recognize that while all these goals are achievable, some will take more time than others. Whatever the critical objectives, it’s important to take a value-based approach to build support and foster collaboration within the team to ensure your data governance approach is successful and sustainable.
Bridging the Divide
Data governance requires the business and IT to bridge a classic organizational divide. Building a culture of collaboration can be a challenge. Two approaches must work in parallel for IT, data owners, stakeholders and consumers for all data to come together: the directive and the value.
- The directive refers to an executive leadership mandate that teams work together and produce specific deliverable(s) by a specific date. In some cases, tying the progress of the data governance program to team member performance reviews is a necessary motivator.
- The value is more personal and speaks to benefits of data governance. In this approach, people are driven to join teams and solve their collective, daily pain points. People are constantly being asked to do more with less so showing how data governance can help them become more efficient will capture their attention and ease the adoption of the program.
Moreover, a balance between these two approaches is necessary or the program will suffer from a lack of commitment or attention. Similar to other large initiatives like implementing a new financial accounting system or building an enterprise data warehouse, clearly defined milestones and accountability for execution are necessary. In addition to the directive, the people must understand that the ultimate purpose of the initiative is to help make their jobs easier.
Often, the best person to push the program and promote both the directive and the value is the data governance sponsor. This champion understands the goals of the initiative as well as the organizational limitations or barriers. This person can facilitate bridging the divide and can work to promote accountability, teamwork and momentum.
Parting Advice
Data governance is a set of ongoing, iterative activities that must be continuously maintained and updated. Don’t wait for the perfect methodology, the perfect team, or perfect tool before mobilizing around a data governance program. Rather, roll out the program in increments. Use lessons learned from each roll out to refine the approach and roadmap. Preview upcoming data governance releases to encourage broader participation across their enterprise. Get employees excited and engaged in the new activities and capabilities.
Starting a data governance program need not be intimidating or challenging. Identify how data governance can benefit your business, move it to the top of the priority list and motivate your team to collaborate in a seamless manner.
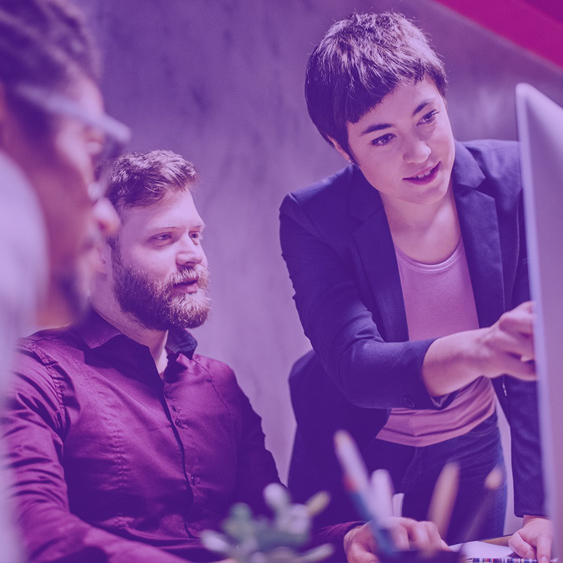
Building The Framework For Successful Data Management
Learn what experts are needed to drive data management success
Data-driven organizations know how to leverage their data as a strategic asset to optimize business processes, improve decision making, enhance the customer experience and increase revenue. But leveraging these assets is about far more than just managing data, it’s about building a culture committed to maximizing data value, where stakeholders are engaged and business users are empowered to seek out data to augment business strategies and objectives.
A comprehensive data management strategy should include a foundation of data governance and metadata management to promote data understanding, accessibility, usability and utilization. The underlying culture that makes such a strategy successful requires a partnership between business and IT, accountability among data owners and stewards and cooperation and collaboration across an enterprise to gather and maintain critical information such as conceptual metadata. All of this should enable business users to easily locate and apply data to business problems, turning that raw data into actionable insights.
However, building this culture and becoming a data-driven organization doesn’t happen overnight. There are many steps business leaders must take to implement metadata management. In a previous chapter we focused on the building blocks of metadata management. In this chapter we’ll examine how organizations can cultivate a data-driven culture.
Solid data management must begin with the right tools, but it is the combination of technology, people and processes that enable enterprise-level excellence. The world’s finest hammer may as well be a paperweight without a skilled craftsman to wield it, which is why data management success starts with the right team.
Creating a Data-Driven Culture Starts at the Top
As anyone who has tried to implement a data management solution will tell you, without executive buy-in and budget, the project is dead in the water. Senior management needs to understand and promote the value of data assets and the importance building a data-driven culture, and demonstrate that commitment as a data management team is built. Leadership needs to hire the right expert who can evangelize and oversee the data strategy for the entire enterprise. Many organizations today tap a Chief Data Officer (CDO), while others leverage data consultants to act as a de facto CDO.
Immediately under the CDO should be a senior director to act as the head of enterprise adoption. Their responsibilities include integration, adoption and compliance for new data policies, standards, analytical methods and various capabilities recommended by the CDO. Success in this position requires extensive experience in change management, and a deep understanding of technology development lifecycles.
Next, organizations should enlist a technically savvy senior manager to take on the role of data engineering manager. This role is responsible for leading teams that build high performance, scalable data solutions to meet the needs of data creators, managers and consumers. This person may also manage the data platform and work with a variety of teams and individuals, including product engineers, product managers, designers, analysts and data scientists to understand their data supply chain needs and develop innovative solutions for data ingestion, preparation and delivery.
To round out the team is the data evangelist. This position can be filled by a data analyst experienced in publicizing and energizing the work of others. This job is essential in driving data knowledge participation to discuss, organize and define data from diverging lines of business. There are a variety of approaches the data evangelist can take, but the main goal is to spread broad data knowledge and encourage participation from and collaboration among different departments.
Facilitating Data Knowledge Participation
Among the data team, the data evangelist is essential to creating a data-driven organization, because they are likely spear-heading organizational efforts to gather conceptual metadata. The data evangelist, or their designated team members, is tasked with gathering that data which resides within the minds of employees across varied departments and lines of business. To gather this conceptual metadata across business functions, they must interview subject matter experts and work cross-functionally with various business and technology teams.
There are, however, many barriers to gathering this knowledge. First, there is simply a matter of logistics. Gathering experts from various departments together in the same room at the same time, in order to share their expertise, is no simple task. Then there is the general level of skepticism that comes with the introduction of any new process or technology. There will be change averse employees, and those who doubt the efficacy of the new procedures. It can take time to create converts among employees. Lastly, there are those employees who feel threatened by shared knowledge. They guard the knowledge they have as a sort of shield protecting them and their position. These employees will not readily give up what they perceive as a source of their power.
However, knowing these obstacles exist is the first step to overcoming them. The data team needs to find innovative ways to engage users and encourage their involvement. One approach a data evangelist may utilize to motivate participation is by implementing an internal marketing campaign to get people excited about data and gain their buy-in. For example, the data evangelist can craft various marketing materials to connect people with data insights to show how data specifically benefits their team. They can also take a more entertaining approach and use “gamification” or competitions to increase participation and interest, or train the team using real-world examples to demonstrate how data knowledge is relevant to their work.
If internal marketing doesn’t do the trick, data evangelists can work with the human resources (HR) department to further engage employees. HR can provide special recognition for data engagement, like acknowledgements on ID tags and email signatures, prestige giveaways or exclusive perks like time off, bonuses, free lunches, etc. to help spur participation.
In a data-driven culture where participation is encouraged, rewarded and respected, teams are more likely to take an active role in data analysis to make to smarter business decisions, enhance strategies and fine-tune objectives to gain a competitive advantage and increase revenue.