eBook
Creating an Agile Data Quality Strategy for Effective Regulatory Compliance
Read this eBook to explore how an agile, iterative data quality strategy can help your organization streamline compliance and proactively face new regulations with confidence.
Introduction
As both individuals and nations become more concerned about privacy and security, it is certain that data-focused regulations will continue to grow in number, breadth, and depth. This has many implications for businesses.
Some companies put in place technology and mechanisms specific to each regulation with which they must comply. While this approach does bring organizations into compliance, it is a narrowly focused approach with limited benefits, and puts organizations in a position of playing catch-up as additional regulations are passed.
A broader approach that offers wider benefits is to invest in new ways to catalog, understand, measure and monitor your data so that you can make confident assertions that can be proven to regulators consistently over time. This paper argues that data quality is perhaps the most productive way to invest in a compliance architecture and get off the expensive whack-a-mole compliance treadmill. With an approach that prioritizes data quality, companies can measure how well they’re complying with regulations at a granular level while at the same time driving greater business value from their data.
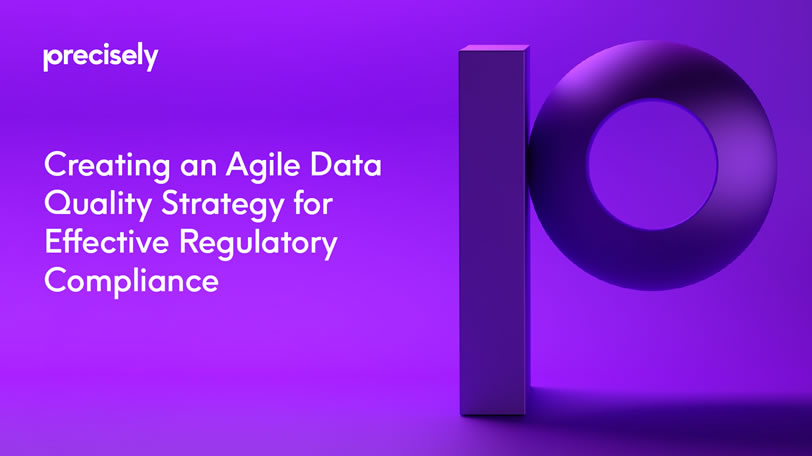
The general characteristics of compliance and data regulations
When companies think of compliance, they usually think first about government regulations like the European Union’s GDPR. Other regulations apply to particular industries, such as the Comprehensive Capital Analysis and Review (CCAR) and Dodd-Frank Annual Stress Testing (DFAST) for financial services or HIPAA for healthcare. These regulations each have their own compliance requirements.
To work with their business partners, companies must also conform to industry standards like electronic data interchange (EDI) or SWIFT messages. While not compliance from a legal standpoint, these standards require that the data exchanged all have certain agreed-upon characteristics for interactions with business partners.
Finally, there are often internal policies within a company, which may not be prescribed at a governmental level but that are part of what the business is really concerned with monitoring. These could be financial targets like recurring revenue or quarterly sales, which also rely on key or critical data elements.
Given the myriad regulations and policies companies must address, to streamline compliance, companies need to be able to figure out a way to create a process that is more universal rather than setting up processes to comply with each individual regulation.
A broader view of compliance focuses on data and data quality. Thus, at all of these levels, what a company needs to examine from a compliance standpoint includes determining what critical pieces of data are required and whether each of those elements is at a fine-grained level or a summarized level. Further, examine what data the business is generating that’s going to be measured or processed from a compliance perspective and what must be done to report on it. There should always be a connection between what the company needs to report on and the data it is collecting to do so.
Why does data quality matter with respect to streaming compliance?
Data quality is an essential component of streamlining compliance because companies have to ensure their reporting satisfies the regulations — especially when it comes to external regulations like GDPR or the California Consumer Privacy Act. Failure to comply with such regulations could result in hefty fines, so if companies do not have quality data and information, they’ll be unable to truly assess whether or not they’ve met the compliance thresholds.
But data quality helps in more ways than providing companies with the confidence that what they’re reporting is accurate. Data quality is about understanding content, whatever it happens to be, and applying rules about the expectations for a particular piece of data. From those rules, measurements or metrics can be developed and serve as evidence that the requirements of compliance have been satisfied. These metrics around data quality can become part of a larger compliance framework.
How can focusing on data quality fuel a better approach to compliance?
An agile, iterative approach that starts from the data up creates a foundation for compliance across regulations. Start by identifying the range of regulations the company must currently comply with. Review which key data elements are required to comply with each of those regulations and build out a matrix that shows where each element appears across regulations. Determine the kinds of rules and checks that need to be put in place to ensure compliance.
Data quality tools provide profiling capabilities that enable you to drill into a particular data element and see what types of values are there, comparing those values to what is expected. Such tools enable you to create rules that assess the completeness of the data, its consistency and its validity. These rules can then be vetted with subject matter experts to ensure that they accurately reflect what’s needed for compliance. Once vetted, a process can be put in place to monitor and measure the quality of critical data elements on an ongoing basis.
With such data quality metrics in hand, the effectiveness of the overall compliance effort can be evaluated. Is the organization as a whole on top of compliance? Are there particular lines of business that are not keeping up with their reporting?
Data quality tools can also help identify more complex problems in aggregate data. Take healthcare, for instance. If an insurance company is expecting a particular claim process, certain sequences of events should occur. For example, an encounter between the client and an outpatient clinic should occur before the client goes into inpatient care or for outpatient surgery. While these events often span multiple records, data quality tools can validate these types of required sequences and identify anomalies.
By measuring data quality using rules and metrics including trends over time, data quality can be improved and evidence of compliance can be provided. By starting with the data, that evidence serves not just one regulation but any regulation that looks at that data element.
Furthermore, data quality information can be incorporated into a broader environment, feeding issue management systems or BI dashboards.
Conclusion
When dealing with compliance, companies should always return to the question of how the data and their data governance and compliance processes can provide them with continuous value. The effort should not be hyper-focused on a particular regulation. Instead, companies should push beyond this and recognize how they can use the same high-quality data to fuel a variety of use cases.
A data quality-focused center of excellence enables companies to get ongoing continuous value out of their tooling and capabilities and provides examples for people within the organization to recognize how the solution to one compliance issue could be applied to other challenges and opportunities.
This rethinking of how companies approach compliance and data quality has applications not only to external regulations but also to internal policies and the way the organization’s leaders think about the management of their lines of business.
Such an approach fuels a fresh way of thinking about data quality and compliance, with an eye toward equipping the business with capabilities to address compliance at broader level and making the entire business more data literate. The more people who learn to understand data more deeply and focus in on particular metrics of value, the better. Such an approach benefits both individuals looking to succeed at their jobs and the company as a whole.
By adopting an agile, iterative approach to data quality, organizations can move from being overwhelmed by compliance with yet another regulation to confidence in the data they have and its quality to meet whatever compliance demands they face.