eBook
Adding Data Governance Insights with Data Quality
Why data governance is top of mind
The amount of data in the world is exploding along with the intricacy of it. Where we used to have internal sources, we are now working with data links or cloud data warehouses. Each source needs to be governed because we are responsible for the data that is used to make decisions and drive business. As data grows, we also see broader and deeper privacy and AI regulations emerging.
55% say data governance results in improved data quality
–2024 Data Integrity Trends and Insights Report
Data governance needs data quality
For compliance reasons, we need to meet regulatory requirements to make business decisions. We need to make sure that we can trust the data and that our data policy will create a unique customer view.
Recent studies have shown that 27% of practitioners completely trust their data, and of those who struggle to trust their data, 70% say data quality is their biggest issue.
With data governance, you have trust that your data is in the right hands and the ability to provide insights across the organization.
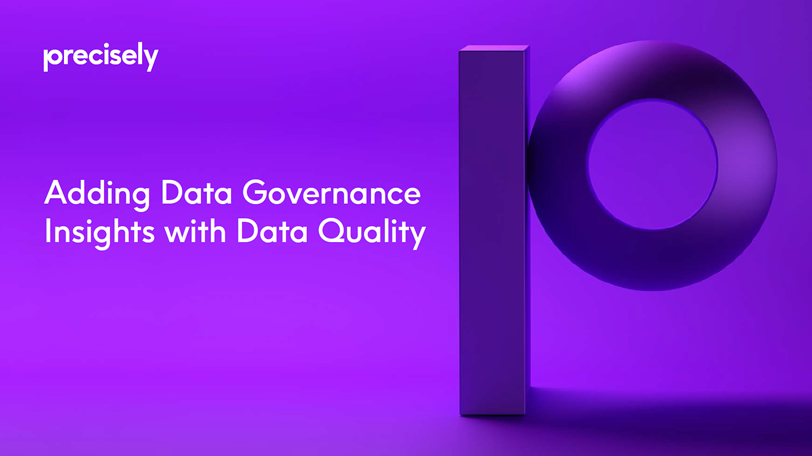
Data governance
The set of policies, processes, rules, roles, and responsibilities that help organizations manage data as a corporate asset.
- Ensures the availability, usability, integrity, accuracy, compliance, and security of data by:
- Putting trusted data assets in the right hands
- Providing insight across the organization
- Streamlining data management with repeatable practices
Data quality
The processes and rules that help ensure that data is “fit for use” in its intended operational and decision-making contexts.
- Provides accuracy, completeness, consistency, relevance, timeliness, and validity of data by:
- Assessing the current state of data quality
- Putting rules in place to validate data in a constant form
- Delivering insights on data to those who need it
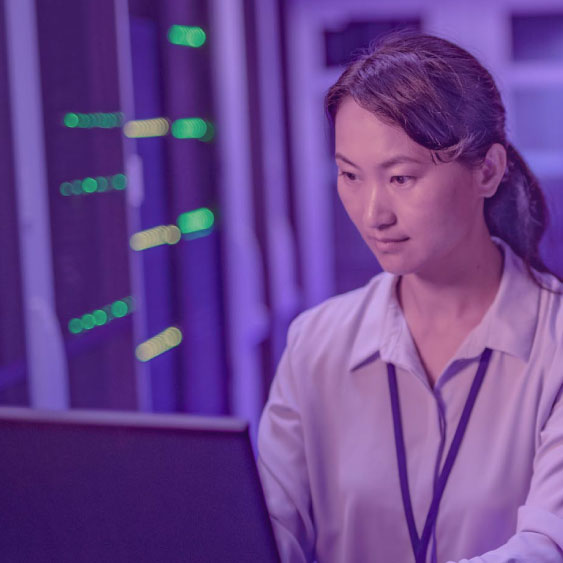
Symbiotic relationship between data governance and data quality
Data governance needs appropriate data quality and data observability tools that not-only clean the raw data, but also illustrates data errors, peculiarities, and issues to help compile the best standards and monitor data quality over time, including timely identification of outliers and anomalies.
Data quality needs appropriate data governance tools to ensure the data is cleaned and maintained within an appropriate data framework that is relevant and pertinent to business needs.
Streamlined data management with repeatable practices ensures the availability of data to those that need it. Data quality and data observability combined with data governance can make sure that the data is fit for use and that it’s not only functional but also compliant, with the appropriate accuracy, completeness, consistency, and relevance. We have access to all these sources that can validate business rules, put the rules in place, and monitor them. Over time, data quality will deliver insights from the combined rules and metrics.
Executing a business rule on source data not only describes it functionally within the data governance refinements but also makes it actionable to test its validity. When those holes are filled, we can measure the data to determine compliance. Trials can be put in place and those rules can be executed over time.
How the Integration of data governance, data quality, and data observability adds insight and value
Data governance, data quality, and data observability need each other, or they will not survive. Data governance delivers high quality data by allowing us to define rules and policies, and then evaluate the data by looking at metrics. With data quality and data observability, we can execute those rules, but we need an appropriate framework to let someone know if there is an issue and that it can be addressed within the organization.
Data governance tools
- Help business users define rules to govern the level of data quality that is acceptable
- Analyze metrics to understand trends, risks, and costs
- Provide reports for common insights into data across the organization
- Show data lineage to enhance trust in data and identify impacts downstream
Data quality tools
- Profile the data to determine the current state of quality, distribution of data, relationships between data series
- Express business rules in valid technical syntax so they can be evaluated against actual data
- Measure data to determine compliance with business rules and thresholds on an ongoing basis
- Proactively uncover data anomalies before they become costly downstream issues
- Correct data to make it more usable
Maximizing business value for data use case
To drive real value, organizations must empower every data citizen to find the right information, assess its quality and trustworthiness and use it confidently to make better decisions.
Make it discoverable.
Help data users find data that is fit-for-purpose, discover new datasets crowd sourced by their peers, or tag relevant data
Make it understandable.
Give data users and clear picture of who owns the data, where it comes from, what it means, and how reliable it is
Make it trustworthy.
Help data users know what data they can use, how to use it and when to share it
Conclusion
The need for data governance is growing, as demands to drive business value from data are increasing, and the fines associated with the regulations are becoming more frequent, costly and publicized. Precisely’s data quality and data observability solutions provide a robust set of capabilities to ensure confidence in your data, integrated with the Data Governance service in Precisely’s Data Integrity Suite to support a broad range of data governance needs.