eBook
4 Keys to Improving Data Quality
The hidden barriers to becoming data-driven
It is reasonable to expect that data-driven decision making would follow the usual pattern of development and adoption: early adopters start out with centralized, carefully managed projects which eventually move into limited production. Then as the value of the technology becomes clear, implementations begin to proliferate, resulting in more distributed use.
What drives this ‘standard’ technology adoption pattern? Trust. Or more correctly, the lack thereof. Concerns about the complexity and cost of the required technologies and business processes undoubtedly play a part in slowing the adoption of data-driven decision making. But efforts to become a more data-driven organization actually suffer from an even deeper layer of mistrust. The problem here is not just the technology and systems involved. It is the deeply rooted lack of trust in the data itself.
At this point, the science and technologies for data analysis are already quite powerful and well proven. In fact, the current trend is rapid advancement of Business Intelligence (BI) and analytics into the realms of Artificial Intelligence (AI) and Machine Learning (ML). But it is exactly such advancements which are now making the underlying problem of poor data quality painfully obvious. When you apply advanced analytics, and especially when you unleash AI and machine learning, the impacts of bad data are magnified.
Data quality issues have often been considered unavoidable and uncontrollable, and so living with bad data simply became normalized as just another cost of business. But the unavoidable reality for any organization looking to adopt data-driven decision making is that it cannot succeed in doing so without fully and actively addressing the age-old scourge of poor data quality.
This eBook will guide and inform you regarding how to overcome the root problems of data quality, not just identifying specific types and patterns of quality problems, but also clarifying the roles of data quality management and data governance in resolving them.
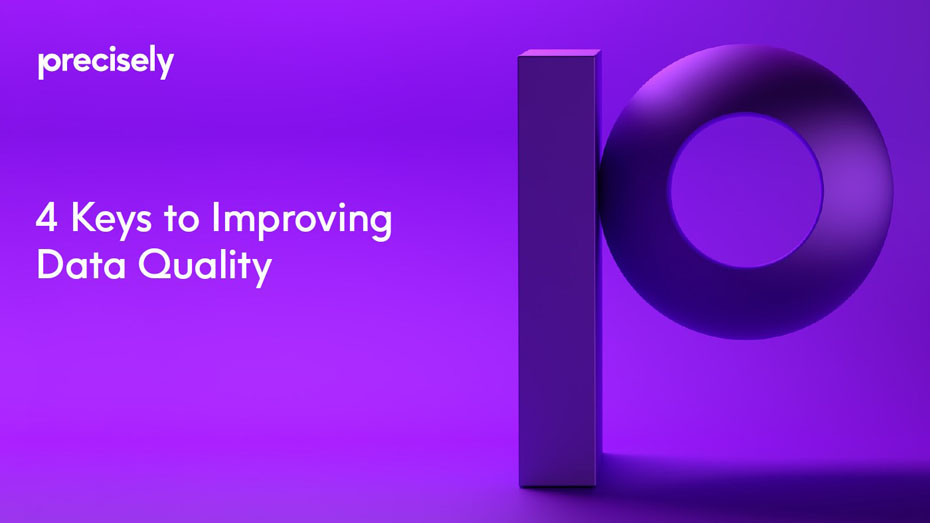
Key #1: Value alignment
Focusing investments and work efforts on goals that actually matter to the business is basic common sense. In other words, there has to be a valid business case. As noted earlier, data quality management efforts have suffered in many organizations because pulling together a really compelling business case for dealing with the problem is not easy.
Just identifying the sources of quality issues can be a maddening chase to hunt down a moving target. Then figuring out steps to remediate the cause can be elusive, especially in cases where the data issues seem to occur almost randomly or sporadically. And finally, there is the challenge of getting people and departments to take on responsibility for cleaning up and maintaining data quality.
The first key to driving improvements in data quality is to approach the problem by identifying the impacts of data quality problems and make them clear to everyone involved. The critical path in this case runs through the alignment of any data quality initiative with the currently measured and reported business results that are most fully impacted. In other words, it is about getting to agreement on “why” resolving data quality problems is not just a side issue but is in fact critically important to key, highly visible business metrics.
So, the first step is a coordinated effort to capture the impacts, rather than the causes, and to pull those impacts together into an understandable narrative. Often, this is something sponsored and/or driven by C-level executives, whose job performance is measured by overall organizational performance and results. Then, from within that broader set of impacts, identify those that are likely to be causing the most damage financially. Doing so sets the stage for everything that follows.
Once there is awareness and agreement at the executive level, the same core messages need to be delivered across all functions in the organization, with attention to presenting the nature and costs of the impacts in terms which are relevant to their jobs and responsibilities.
The key result needed from all these efforts is gaining informed buy-in at multiple levels, not just for the dream of better data quality, but more importantly for the commitment to getting it done.
The goals of getting everyone on the same page about data quality and gaining buy-in to actively drive improvements are also core tenets of data governance. And they are not the only ways that data quality and data governance mirror each other. In the simplest terms, data governance involves building a framework of policies, processes, and standards for how an organization manages its data for the purpose of delivering better business outcomes.
So, whether or not your organization has an official data governance program in place, it is helpful to adopt a bit of a structured data governance mindset when addressing data quality problems.
Key #2: Visibility and accountability
Having gained agreement that, in fact, data quality is a real and costly problem, the next step is to make the sources and impacts of poor data quality clearly visible. In other words, work on identifying specific examples of data quality problems and assessing how serious or costly they truly are. As before, it is critical to avoid the ‘blame game,’ working instead to reward, and even celebrate the discovery and evaluation of example data quality problems.
Encouraging and rewarding such discoveries helps to set a pattern for the future. Your efforts now to “clean up” data will be wasted if it is regarded as a one-time, fix-it-and-forget-it program. Once you clean up your data and adopt data-driven decision making as your standard way of doing business, you cannot allow data quality to slip or degrade over time.
Instead, following data governance best practices, data quality management needs to be fully operationalized, baked into your business processes and the habits of everyone in the organization. If you hope to get everyone to commit to maintaining data quality over the long term, the initial efforts need to be a net positive experience and the ongoing processes and tasks need to be wrapped up in a positive feedback/reward loop.
On a practical level, it is most efficient and effective to fully involve the people most familiar with the data, the ones who work with it on a daily basis. They may not be able to evaluate how costly or deeply rooted any given quality problem is, but they can point your data management specialists to the problems that cause them the most grief and give them better insight into the real impacts of the issues. In the end, there is no more powerful positive reinforcement than seeing one’s concerns being addressed.
It then becomes the responsibility of data management specialists and departmental leaders to evaluate those findings and to determine two things: How much each issue is probably costing the organization and what the definition of “fixed” would look like. But the key at this juncture is to get everyone actively involved in the process of identifying truly problematic issues and making that process a positive experience.
Key #3: Empowerment
One of the core tenets for successful data-driven decision making is data democratization. Simply put, data democratization means virtually anyone within your organization has the ability to access and use data to inform their decisions. This requires that, within reasonable limits, regardless of their role within the company, anyone charged with making business decisions has access to the data and the tools they need to do so.
Of course, it is neither wise nor feasible to just simply give employees unrestricted access to data and expect them to make good decisions. Yet maintaining completely centralized control and responsibility for managing data quality only perpetuates the same legacy data management drags and impediments to democratized analytics. There has to be a middle ground, where people have the right kinds of tools at hand to enable data access and analysis, without relinquishing control over the quality and trustworthiness of the data they are using.
The answer is to enable collaborative data quality management. The most effective and powerful solutions include data profiling and data quality management modules which integrate with the organization’s data governance systems. This allows end users to examine the data they are working with, evaluate it against the data quality standards and records maintained in the data governance systems, and bring questions or concerns to the responsible data manager.
Huge benefits can accrue when data users who are subject matter experts are empowered and enabled to identify, evaluate, and communicate data issues to the data managers ultimately responsible for data governance.
When you start by enlisting everyone in the organization to help with identifying clear examples of data quality issues, and then leverage that momentum by enabling them to contribute to the ongoing data quality management process, you set yourself up for long-term success.
Key #4: Strategic and tactical priorities
Your industry and the types of products and services you provide certainly shape the general nature, scope, and sources of your data. But more to the point, your data sets are absolutely unique in the details of their formats, where they are maintained and exactly how they flow between your systems. So, it follows that the details of your most common or most problematic data quality issues will likely be just as unique.
Especially in the early stages of your data quality improvement project, it is vital to balance your efforts between dealing with the resolution of your unique but clearly urgent data quality issues and your goal of building up sustainable and effective data quality management practices. Be careful not to lose perspective, to get into data quality management routines that are too narrowly focused on tactical efforts aimed at resolving this morning’s newest problem.
Instead, maintain a data governance mindset, dedicating some time to look into and evaluate the most common sources and patterns of poor data quality, even as you prioritize addressing your most glaringly obvious and unacceptable problems. Because as unique as your business and your data may be, they will also surely suffer from many of the same ailments as any other organization.
To help get you started, here are some areas where pervasive data quality problems commonly arise. It is likely that as you review these you will find yourself recognizing themes and patterns appearing within the specific issues your organization is uncovering.
Data Validation
Maintaining data quality standards when handling high volumes of data is as difficult as it is imperative. Basic data format compliance, consistency of data residing in multiple locations or systems, and even details as granular as defining and managing null values within data sets must be continuously checked and controlled.
Threshold & Reasonability Checks
It is possible for data to be clean, accurate, and complete, but still be complete nonsense. Automated statistical controls are needed to validate all new or updated data against real-world criteria before being accepted. Accepting and using data that is outside of a strictly specified range or that is just wildly different from normal or recently validated values can cause very serious business problems.
Data Freshness
Your most valuable and important business data is rarely static. Change is constant, especially for data related to customers, logistics, ecommerce, etc. While the rate of change varies by data type and source, the business impacts of relying on out-of-date data are similar. Capturing data changes in a timely manner generally requires integration with external, third-party systems and data sources as well as between internal systems and data lakes. Here again, automation for data quality checks and controls is a requirement for just about every business.Your most valuable and important business data is rarely static. Change is constant, especially for data related to customers, logistics, ecommerce, etc. While the rate of change varies by data type and source, the business impacts of relying on out-of-date data are similar. Capturing data changes in a timely manner generally requires integration with external, third-party systems and data sources as well as between internal systems and data lakes. Here again, automation for data quality checks and controls is a requirement for just about every business.
Anomalies and Outliers
Unexpected data changes can wreak havoc on your business. If these changes continue to go unnoticed they can cause severe impacts and data downtime. Using AI and machine learning, data observability capabilities automatically detect anomalies, outliers and other unexpected changes in the data. The appropriate users are notified of possible problems that have the potential to disrupt the business and create costly downstream problems.
Deduplication and Entity Resolution
The unavoidable mix of data stored across clouds, mobile devices, social media and traditional repositories makes it extremely difficult to avoid the problems of duplicate data. Rules, tools, and automation for deduplication and entity resolution are vital for ensuring that customer data and other critical data sets are always accurate, valid, and complete.
Reconciliation
Data sets can get out of synch whenever new data is added. This is especially true when data sets are purchased from third-party sources and when receiving data streamed from connected resources. Connected resources could include CRM solutions, credit validation systems, trade systems and others, depending on your industry. It is vital to ensure that such data is checked and conformed to standards and that it is properly reconciled with your existing data before it is ever used.
Data in Motion
As data moves between systems and processes within an organization, previously valid data has the potential to become inaccurate. It is important to ensure that at each step, any required data transformations are performed correctly and that the business rules being applied are working as intended. And it is just as important to ensure that no data is lost or corrupted during the basic process of moving between systems. An example of this is a set of claims entering an insurance organization. The claims data must move through a sequence of operational systems before being finalized. There are critical data elements in each transaction that need to be monitored for accuracy and completeness at every step along the way.
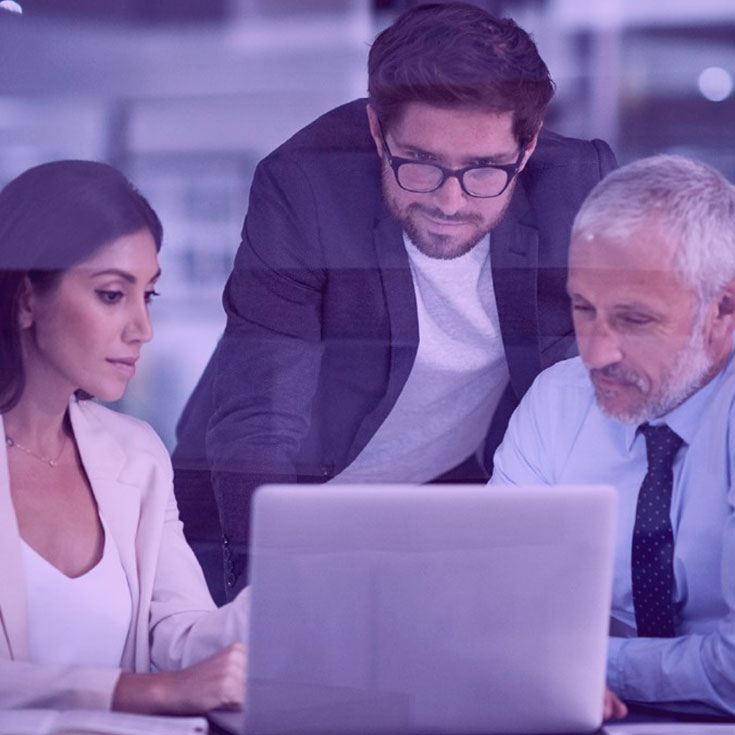
High quality, data-driven decisions
In the end, successfully adopting and leveraging artificial intelligence and data-driven decision-making requires trustworthy and democratized data. But that cannot be done without addressing data quality. The best results are achieved by automating and enabling collaborative data quality management, within an active, well thought out data governance framework.
Such a paradigm shift can’t be achieved by just implementing advanced software packages and training a core team of data scientists to manage it. Instead, to reap the exceedingly valuable benefits of data-driven decision making, your entire organization must be educated, involved, and empowered to manage the ongoing, mission-critical work of managing the quality of the data upon which it depends.
Data quality solutions from Precisely can help you find, understand and trust in data.