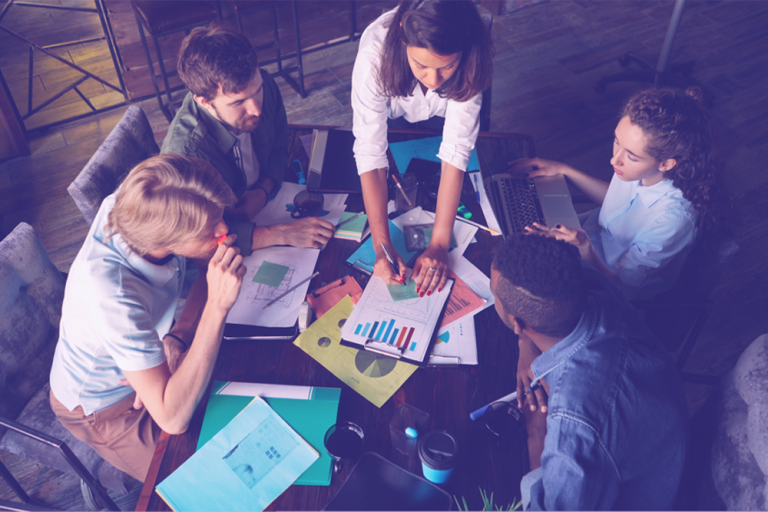
Data Strategy SOS: Signs Your Data Initiatives Need Help
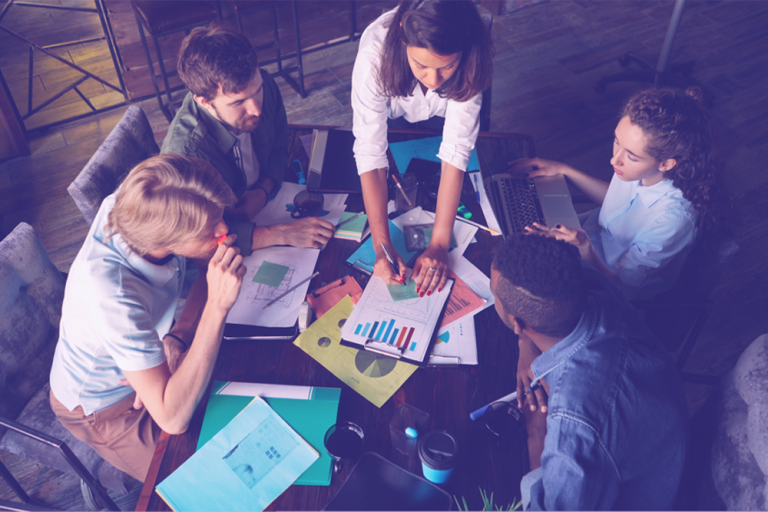
It’s indisputable that in today’s business environment, data comprises a valuable organizational asset, providing the keys to competitive differentiation and strategic advantage. In that context, data governance has emerged as a critical discipline to enable business success and a data strategy.
To elicit value from their data, organizations must ensure that it is accurate and reliable. They must understand where it is located, where it came from, and how it is being used. To achieve that, enterprises need a structured and deliberate approach to managing data assets and ensuring high data quality.
In this respect, data governance has become imperative. To be effective and see positive ROI, governance must be business-driven and quality-focused. Companies that fail to address these objectives effectively will ultimately flounder or fail to capitalize on the business opportunities before them.
It’s no surprise that enterprises across virtually every industry are investing in a data strategy with the hope of gaining the upper hand over their competition. To succeed, those programs must be designed and developed on a foundation of purpose, focus, accountability, and organizational engagement.
Without those factors, data initiatives will ultimately fail. That means losing the confidence of users initially, but eventually, it means lower budgets and loss of executive sponsorship and ultimately defunding of the program. If you’re not getting the results you want from your data initiatives, look for these critical signs that your programs may need help.
Read our eBook
Building a Data Governance Use Case to Get Budget and Buy-in
To learn more about building a successful data governance program in your organization, read our free ebook.
1. Failure to Link Data Assets to Business Objectives
Every data strategy must begin with a sound business rationale. There are some obvious reasons for this, including the need to secure budget and executive support. Typically this involves some cost-benefit analysis and a broadly defined pitch outlining the benefits of your data project. Many data initiatives lose momentum shortly after launch because they fail to deliver on those initial promises. Instead, they lose focus on the business case that justified the program in the first place.
Data governance for its own sake is destined to underperform. Instead, documenting business value and metrics of governed assets must be a primary and constant focus. Program owners can then must evangelize the value of the initiative and back it up with demonstrable ROI that delineates the benefits of high data quality, reduced costs and risks, increased revenue, improved compliance that is delivered by well governed data.
That means defining specific use cases, prioritizing opportunities to add value, and delineating the specific ways in which data governance and data quality will help the organization meet its business objectives.
2. Failure to Prioritize Data
The second critical sign that your data initiative may be in trouble is that the program owners have failed to prioritize the datasets with which are linked to high value projects. Many organizations feel that governing all of your corporate assets is imperative – when in reality, as little as 5% of data directly contributes to the most important corporate KPI’s and initiatives. Data programs that prioritize critical data and measure its impact achieve value five times faster, on average, than those that do not.
It’s important to use the right criteria when defining which data is critical. It can be tempting, for example, to simply ask users which datasets are most important. This approach usually results in a multitude of different answers, and no consensus with which to drive a decision.
Instead, Precisely recommends asking which business goals and objectives are most critical, then determining which data assets are vital to those business processes. A good way to determine this is to identify data that drives high value results in one business area, but also directly impacts other business results. For instance a key business objective may to be create more personalized offerings to customers or members. Governing data assets that help achieve this KPI can also impact organizational goals to improve customer service response time and meet compliance requirements around privacy or other regulatory requirements. By identifying those programs that contribute to the goals of multiple teams, you increase visibility and value of your data initiative across the organization.
Inventory master records, vendor performance data, and stock levels, for example, are essential to effective operations. Through analytics, customer purchase history and enriched demographic data can provide a valuable understanding of the people and organizations that the company serves. Financial transactions, labor and safety reporting, and quality assurance data are clearly all essential as well.
3. Lack of Meaningful Performance Measures
With a clear business case and prioritized datasets, data teams can begin to set clear measurable objectives with other stakeholders throughout the organization. This ultimately enables them to demonstrate the value these programs provide. The combination of prioritized data sets and performance measures yields results roughly five times faster than when data teams fail to define these parameters.
Keep in mind that you may need to define KPIs differently based on the specific business cases and datasets you have prioritized. If a key goal is to improve customer analytics, then your performance measurement will be based on data quality in the CRM system and other databases that contain customer information. That’s likely to also generate value for the sales and finance teams, as the incidence of outdated or duplicate customer records is reduced. Define and report KPIs that are meaningful to the stakeholders whose pain points you are addressing.
4. Inadequate Stakeholder Engagement
Finally, it’s important to create alignment across the three levels of the organization, including strategic management, operational teams, and those who manage data on a more tactical level. These three groups often see business value in very different ways. They may have different views as to what determines success or failure. Effective engagement means understanding each group’s needs and concerns as they relate to any data initiative. Documenting value should focus on the unique value proposition for each affected group, in terms that will make the most sense to them.
It’s also important to keep in mind that users may initially be reluctant to change. To overcome organizational inertia, enlist data governance advocates within the various departments with which you’re working. Communicate often, celebrate your successes, and plan on taking an iterative approach to building value for your organization.
Quick wins or so-called “low-hanging fruit” can be an invaluable strategy for building organizational commitment. Be careful, though, to ensure that the use cases to which you apply this strategy are meaningful to a large enough group within your organization that your success will be seen and understood by a critical mass of stakeholders.
By avoiding these four pitfalls, you can significantly improve the ROI of your data strategy. To learn more about building a successful data program in your organization, read our free ebook, Building a Data Governance Use Case to Get Budget and Buy-in.