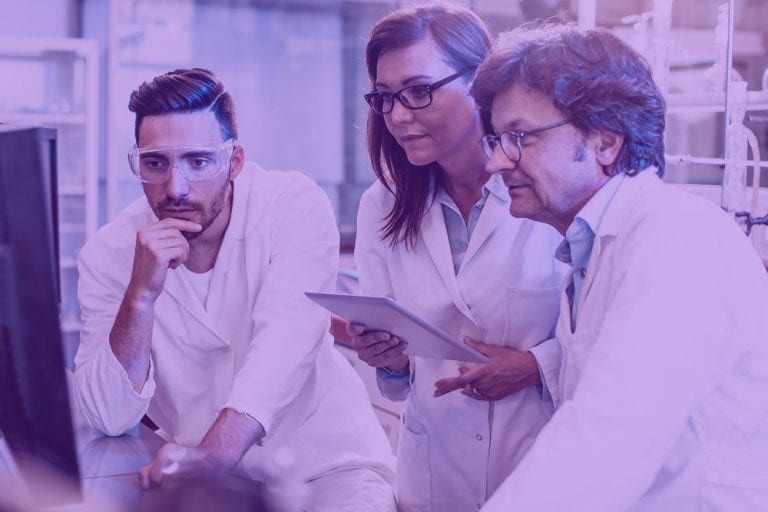
5 Data Quality Best Practices
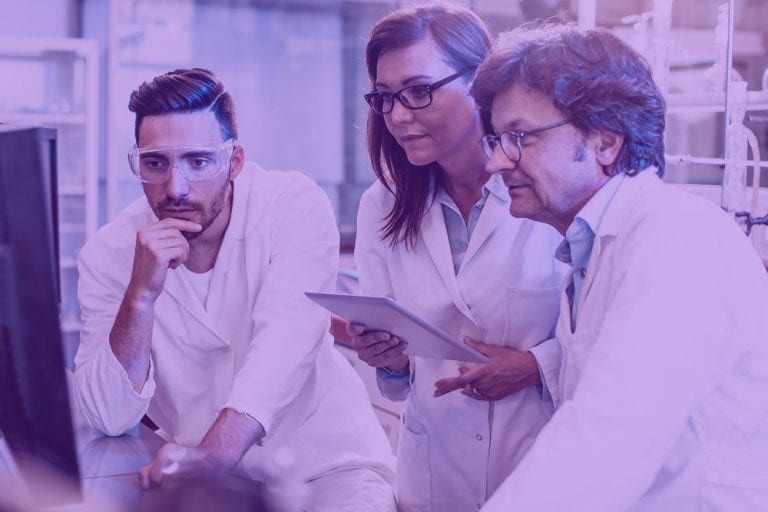
Key Takeaways
- By deploying technologies that can learn and improve over time, companies that embrace AI and machine learning can achieve significantly better results from their data quality initiatives.
- Data enrichment adds context to existing information, enabling business leaders to draw valuable new insights that would otherwise not have been possible.
- Companies should implement a data governance program to ensure the comprehensive application of best practices in data management
Artificial intelligence and machine learning have matured, and cloud technologies are providing the robust on-demand computing power, scalability, and integration necessary to support advanced analytics.
As these trends expand our capabilities, the volume and velocity of information available to organizations today continues to increase rapidly and companies across all industries are looking to improve data quality.
While these trends open the door to vast new possibilities for value creation, they also come with some significant challenges.
- Managing an increasingly complex array of data sources requires a disciplined approach to integration, API management, and data security.
- Growing regulatory scrutiny from government agencies dictates that business leaders allocate attention and resources to data governance.
- Finally, there is the challenge of data quality, ensuring that business users throughout the organization can trust information to be accurate, complete, consistent, contextually relevant, and timely.
Here are five data quality best practices which business leaders should focus.
1. Think holistically: Address the entire data pipeline
Data quality should not simply be focused on finding and fixing existing problems within static data. Data quality programs should be designed to proactively address every step along the data pipeline, treating data quality as a process that begins the moment a data element is first introduced to the organization.
When a user enters bogus credentials on your website to access a download or webinar replay, for example, that anomaly should be detected before it enters your operational systems. Waiting until later risks sending a bogus “lead” to inside sales for follow up. In that scenario, a conventional “find and fix” process fails to deliver value in the same way that a more proactive approach could.
Data quality has historically been perceived as a routine maintenance and repair process – an after-the-fact procedure designed to periodically detect problems with data already stored somewhere in an organization’s databases. Although that can be helpful, it fails to achieve the same levels of data quality that a more holistic approach offers.
Read our eBook
Data Quality 101: Build Business Value with Data You Can Trust
Do you want the insight needed to make business decisions more confidently? Read this ebook and learn more about common data quality errors, characteristics of quality data, and how to start improving data in 5 steps.
2. Embrace AI & machine learning
AI and machine learning have arrived, and they are gaining momentum. There will undoubtedly be growing experimentation and adoption of these technologies in the near-term, and numerous AI/ML use cases are delivering tangible business benefits already, including in the area of data quality.
While some see it as a fundamentally rule-based exercise, many of the associated tasks call for a more sophisticated algorithmic approach. Given the volume of information available and the complexity of modern data sources (including unstructured data), it is impossible (or at the very least impractical) for a human workforce to keep up.
By deploying technologies that can learn and improve over time, companies that embrace AI and machine learning can achieve significantly better results from their data quality initiatives.
3. Add context through data enrichment
Data enrichment adds context to existing information, enabling business leaders to draw valuable new insights that would otherwise not have been possible.
In the insurance industry, for example, a single individual might be classified as the holder of a homeowner’s policy, without reference to their status as the owner of a small business. The same individual may also have family members on a shared automobile policy. A company that understands those kinds of relationships will be in a better position to understand their customers’ needs, identify buying triggers, and compete for their business.
Location context, likewise, adds significant value, including opportunities to validate existing data against external sources. Address verification is a very simple (but important) example. Location intelligence can likewise be applied to verify that proposed mortgage or insurance valuations for specific properties fall within an expected range.
4. Get ahead of the problem
Businesses are relying more than ever on data to add value to business processes and strategic decisions. Unfortunately, most large organizations already recognize that poor quality data is undermining their efforts to leverage data as a strategic asset.
Contact data, for example, degrades quite rapidly, as customers, leads, and suppliers change their names, addresses, or contact information. Human error introduces problems at a fairly predictable rate and even machine-generated data can suffer from anomalies due to malfunctions or gaps in data communication.
Data quality best practices call for business leaders to get ahead of the problem, to take a proactive approach to finding and fixing errors, as well as putting measures in place to prevent issues from arising in the first place.
5. Implement a data governance program
Finally, companies should implement a data governance program to ensure the comprehensive application of best practices in data management. The emergence of data privacy regulations such as Europe’s General Data Protection Regulation (GDPR) and the California Consumer Privacy Act (CCPA) call for a unified company-wide approach to managing data assets.
Heightened data security concerns call for a comprehensive strategy. Perhaps most important of all, organizations must prioritize data as a strategic asset if they are to compete effectively in the coming decade. Those that fail to achieve mastery in data governance will undoubtedly lose ground to more savvy competitors.
Business users across all departments have a considerable stake in data quality and governance. In recent years, we have seen a shift away from traditional IT personnel being the exclusive owners of data quality processes. This is known as the democratization of data quality, as businesses look for tools and technologies that can be used by less technical users.
As a leader in data integrity, Precisely helps organizations to achieve a strategic advantage in an increasingly data-driven world.
To learn more about how Precisely can help your organization achieve better results with data quality, read out eBook Data Quality 101: Build Business Value with Data You Can Trust