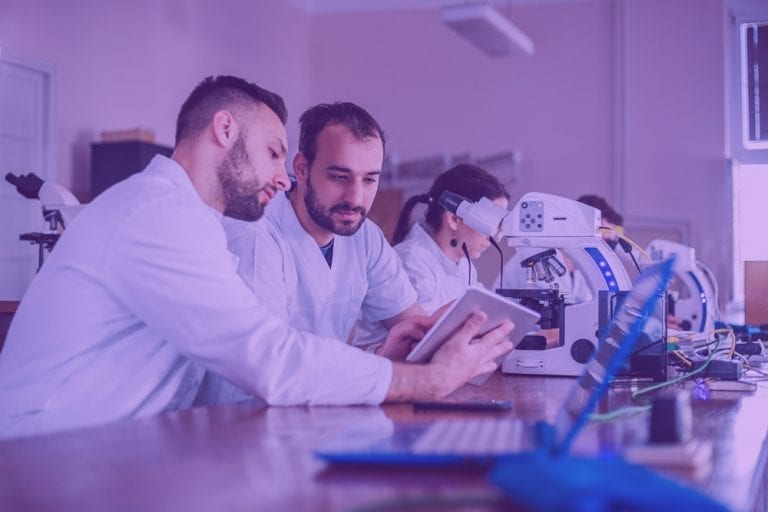
Big Data Calls for Robust Verification Processes
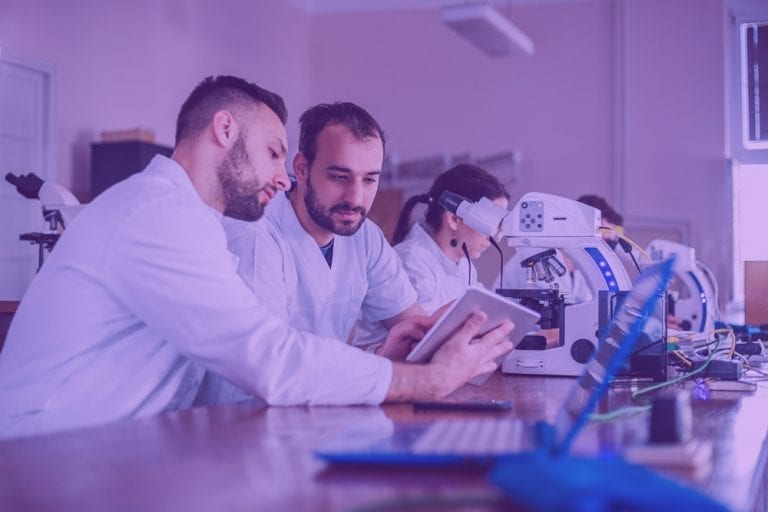
Big data has arrived, and companies seeking a long-term competitive advantage are acutely aware of that fact. A decade ago, businesses that wanted to draw strategic insights from their data were forced to narrow the scope of the questions they were asking because there were hard limits on how much data could be processed and analyzed at one time.
Today, things have changed. Armed with significantly greater computing power, data-driven organizations are able to store ever larger amounts of information and to organize and make sense of that information with increasingly powerful business intelligence tools. Artificial intelligence and machine learning have matured, enabling companies to extract value from their data and put it to use in concrete, meaningful ways.
At the same time, the world is being flooded with more data than ever before. Mobile devices are everywhere, and they can provide a wealth of information about consumer behavior. Machines are equipped with feedback mechanisms that inform technicians when service or repairs are needed.
Product shipments equipped with Internet of Things (IoT) sensors can report their location, temperature, humidity, and condition. High-value shipments may report whether a container has been tilted, subjected to a shock, or whether it has been opened or tampered with.
With so much data available, the task of managing it all is daunting. Data quality is a critically important piece of the big data puzzle.
The data quality imperative
We have all heard the old expression “garbage in, garbage out”. In the world of big data, however, the stakes are much higher. Organizations that fully understand the value of data are incorporating data-driven insights into strategic planning and decisions. When that’s the case, “garbage in, garbage out” can result in flawed decisions with significant negative repercussions.
Enterprise data quality management programs must be capable of accommodating a vast array of different data sources (often in different languages and region-specific formats), and must provide a robust framework for establishing data quality rules and tracking performance.
Consider a simple example. Let’s say we’re consolidating some customer lists from a number of different business units so that we can better understand where our current customers are located, and how we might better serve them with a new store location. We load all of our customer records from several different software systems into a single database table. We have decided to bring over a handful of key customer fields including street address, city, state/province, and zip code.
eBook
Governing Volume: Ensuring Trust and Quality in Big Data
As the volume and variety of big data sources continue to grow, the level of trust in that data remains troublingly low. Ensuring quality in big data is the challenge. Discover how a strong focus on data quality spanning the people, processes and technology of your organization will help ensure quality and trust in your analytics that drive business decisions.
In one of our source data systems, though, a number of customer records have zip codes that are missing a leading zero; so “07515” ended up in our new database as “7515”, for example. What happens when we perform a location analysis of our existing customers? We will undercount customers in certain locations, while other customers may be omitted from the report, or may even be reported as living in a nonexistent location.
Would you want to use that as a basis for deciding where a new store location should be? Clearly not.
It’s a simple example, but it illustrates how a seemingly small data quality problem can have serious implications.
The problem of duplicate records
Consider another challenge. When a single customer appears multiple times in your database, it distorts your understanding of consumer buying habits and leads to errors. When customer John Smith calls to update his address, a duplicate record (still associated with the old address) can lead to redundant mailings or shipments to the wrong location.
A world leader in luxury goods known for its innovation in brand development had challenges with duplicate customer records, and its existing data quality processes were ill-equipped to manage the job. In addition to creating a poor customer experience, duplicate records were costing the company money. Every time the company sent out a marketing mailer, money was being wasted sending duplicate copies to the same customer address.
With Europe’s General Data Protection Regulation (GDPR) and similar privacy laws emerging around the world, duplicate records can also result in compliance issues. If a consumer’s data is deleted based on a GDPR request, while duplicate instances of personal records are overlooked, it could result in a violation and penalties.
The luxury goods company looked to Precisely to help the company solve its de-duplication problem, as well as to ensure the quality and consistency of data as the company embarked on a strategic initiative to use data more effectively in support of business decisions. The result is a better customer experience, cost savings, and stronger GDPR compliance.
Building your data quality strategy
Companies that understand the strategic value of data will have a clear competitive advantage in the 2020s and beyond. A sound data strategy, however, requires a firm commitment to verifying data and managing data quality on an ongoing basis.
As part of a larger data governance initiative, organizations must be prepared to establish clear business rules based on their overall objectives, to apply those rules consistently and efficiently, and to continuously monitor data quality. Data quality scorecarding empowers data analysts to define key performance indicators and monitor those KPIs at a macro level (such as for an entire dataset) or in fine-grained detail (for example, at the individual field level).
Enterprise-grade data quality solutions serve as a foundational building block within the entire data management lifecycle, enabling a data scientist, for example, to investigate the lineage of a data asset and ferret out problems at their source.
The challenges of big data are only likely to get even bigger and more challenging as the volume of data continues to expand, as the number of data sources increases, and as unstructured data plays a larger role in analytics and AI/machine learning. Organizations that wish to embark on a strategic big data initiative, however, should not wait to make data quality a priority. By putting systems in place now, data analytics leaders can get a head start on ensuring the quality of one of their most valuable assets.
To learn more about how you can achieve high standards of data quality in your organization, read out eBook: Governing Volume: Ensuring Trust and Quality in Big Data.