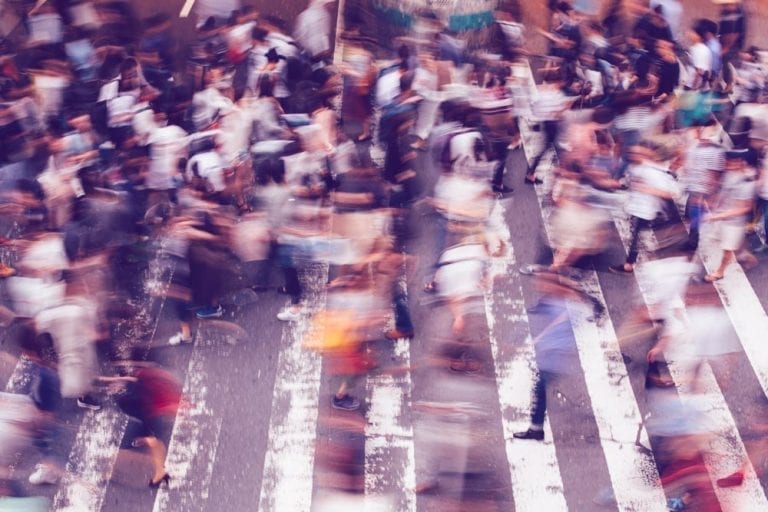
Just How Big is Big Data, Anyway?
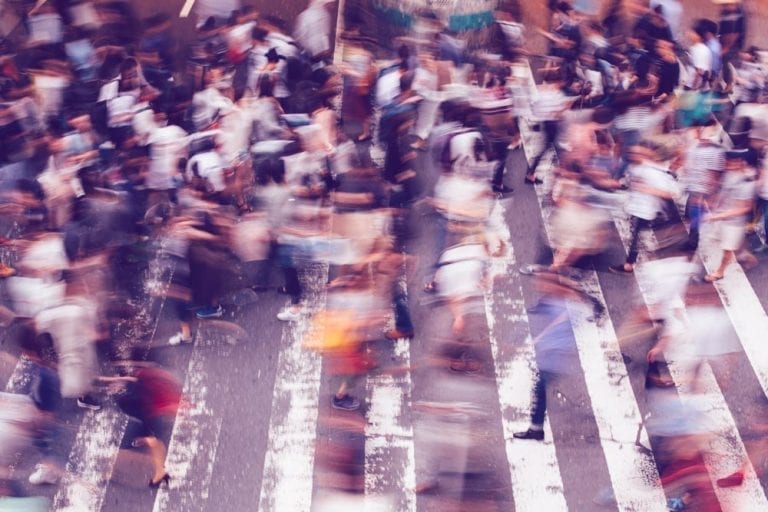
You know that big data involves lots of information. But have you ever stopped to think about just how much, exactly, goes into it? In other words, how big is big data, actually?
Defining big data
Before delving into the question, let’s discuss the difficulty of defining what big data actually means.
There is no official definition, of course. What one person considers big data may just be a traditional dataset in another person’s eyes.
That doesn’t mean that people don’t offer up various definitions for it, however. For example, some would define it as any type of information that is distributed across multiple systems.
In some respects, that’s a good definition. Distributed systems tend to produce much more information than localized ones because distributed systems involve more machines, more services, and more applications, all of which generate more logs containing more information.
On the other hand, you can have a distributed system that doesn’t involve much. For instance, if you mount your laptop’s 500-gigabyte hard disk over the network so that you can share it with other computers in your house, you would technically be creating a distributed data environment. But most people wouldn’t consider this an example of big data.
Another way to try to define it is to compare it to “little data.” In this definition, it is any type of information that is processed using advanced analytics tools, while little data is interpreted in less sophisticated ways. The actual size isn’t important in this definition.
This is also a valid way of thinking about what it means. The problem with this approach, however, is that there’s no clear line separating advanced analytics tools from basic software scripts. If you define it only as information that is analyzed on a complex analytics platform, you run the risk of excluding from your definition datasets that are processed using R instead, for instance.
So, there’s no universal definition, but there are multiple ways to think about it. That’s an important point to recognize because it highlights the fact that we can’t define it in quantifiable terms alone.
Examples of big data
However, we can gain a sense of just how much information the average organization has to store and analyze today. Toward that end, here are some metrics that help put hard numbers on the scale:
- IDC predicts that by 2025, the world’s data will grow to 175 Zettabytes. (To put that in perspective, if you attempted to download 175ZB at the average current internet connection speed, it would take you 1.8 billion years to download!)
- On average, there are about 500 million tweets sent every day.
- According to Nerdwallet, the average smartphone owner uses 2 to 5 GB on his or her cell phone plan each month.
- Walmart processes one million customer transactions per hour.
- On average, office workers each receive 110 to 120 emails per day, equaling approximately 124 billion emails on any given day.
- According to the 2019 Federal Reserve Payments Study, total card payment transactions reached 131.2 billion with a value of $7.08 trillion in 2018, representing growth of 8.9 percent in volume year-over-year.
All of the above are examples of sources of big data, no matter how you define it. Whether you analyze this type of information using a platform like Hadoop, and regardless of whether the systems that generate and store the information are distributed, it’s a safe bet that datasets like those described above would count as big data in most people’s books.
Read the Report
Data Integrity Trends: Chief Data Officer Perspectives
Learn how 300+ C-Level data executives in the Americas, EMEA and Asia Pacific are managing enterprise data assets to fuel reliable data-driven business transformations.
The challenge
It’s also clear that the datasets represented above are huge. Even if your organization doesn’t work with the specific types of information described above, they provide a sense of just how much information various industries are generating today.
To work with it effectively, you need a streamlined approach. You need not just powerful analytics tools, but also a way to move it from its source to an analytics platform quickly. With so much information to process, you can’t waste time converting it between different formats or offloading it manually from an environment like a mainframe (where lots of those banking and other transactions take place) into a platform like Hadoop.
That’s where solutions like Precisely’s shine. Our data integration solutions automate the process of accessing and integrating information from legacy environments to next-generation platforms, to prepare it for analysis using modern tools.
When it comes to successful big data projects, the reality is your business is relying on you to get it right. Understanding that data is a strategic corporate asset, smart business leaders are establishing clear frameworks for ensuring data integrity.
To learn how 300+ C-Level data executives in the Americas, EMEA and Asia Pacific are managing enterprise data assets to fuel reliable data-driven business transformations, read the research report from Corinium Intelligence, Data Integrity Trends: Chief Data Officer Perspectives.