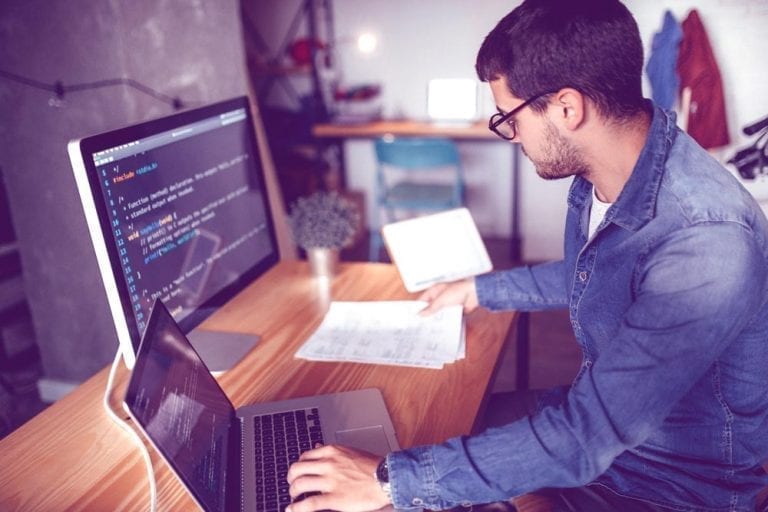
ETL vs. Data Preparation: What Are the Differences?
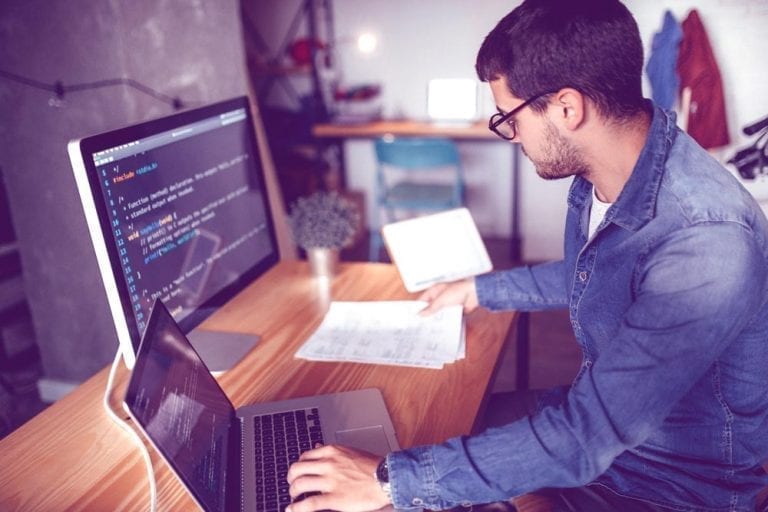
ETL data (extract, transform and load) has for years been a workhorse technology for enabling analysis of business information. But now it’s being joined by a new approach, called data preparation or data wrangling. The two techniques are similar in purpose, but distinct in function and application. Let’s take a look a how the two compare.
How data preparation differs from ETL
Both data wrangling and ETL address the same issue: how to convert data that comes from different sources and in a variety of formats into a common structure for analysis and reporting.
ETL does it, as the name implies, by extracting data from various sources, transforming it into a predefined format, then loading it into a data lake or warehouse where it can be accessed by business intelligence or report generator applications. A key distinctive of ETL procedures and tools is that they are designed as elements of a formal workflow intended for use by IT professionals. Because the organization’s ability to access and use its most critical data depends on the reliable functioning of the ETL system, there is typically a focus on highly structured policies and procedures, along with active monitoring by skilled workers.
Data wrangling, on the other hand, is intended for use not by IT professionals, but by end users, such as executives, business analysts, and line managers, who are seeking answers to specific questions. The idea is that the people who are closest to the data, and therefore understand it best, should be the ones extracting and analyzing it. Historically, these workers often performed their tasks manually using informal processes and employing general-purpose tools such as spreadsheets. Today, data wrangling is seen as fulfilling a critical need in making data more widely accessible in an organization. The title of Self-Serve Data Preparation has now dignified it, and automated tools to facilitate its use by non-IT professionals are beginning to appear.
Read the Whitepaper
A Practical Guide to Analytics and AI in the Cloud with Legacy Data
Read this whitepaper and learn how to avoid the common challenges that organization face when integrating legacy data into their cloud data platforms.
When to use ETL vs. data wrangling
With its formal processes for accessing, transporting, vetting and cleansing data, ETL is best suited for handling well-structured data from sources such as databases and ERP, SAS, or CRM applications. Because ETL systems are normally implemented and managed by highly skilled professionals, they reliably produce data of the highest quality. On the other hand, the very structural rigidity that guarantees data quality can significantly reduce an ETL system’s ability to service new analytics use cases in a timely fashion.
In contrast, the strength of the data wrangling technique is its agility and flexibility. While an ETL system will often be the key component in a company’s data warehouse production environment, data wrangling shines at allowing individual users to get the answers they need quickly. For example, a small team of analysts could employ data wrangling to rapidly evaluate a series of “what-if” scenarios by extracting and analyzing various combinations of data from disparate sources. In such situations, absolute precision may be less important than the ability to access “ballpark” information quickly.
ETL and data wrangling work together
In today’s corporate environment, where the amount of useful data increases daily, combining ETL with self-serve data preparation can help companies maximize the value of the information available to them. And tools to do it are already available. For example, Precisely Connect allows users to create their own data blends without manual coding or tuning.
Learn best practices for integrating legacy data sources, such as mainframe and IBM i, into modern data analytics platforms such as Cloudera, Databricks, and Snowflake. Watch our webinar: Making the Case for Legacy Data in Modern Data Analytics Platforms
Read our whitepaper A Practical Guide to Analytics and AI in the Cloud with Legacy Data and learn how to avoid the common challenges that organization face when integrating legacy data into their cloud data platforms.