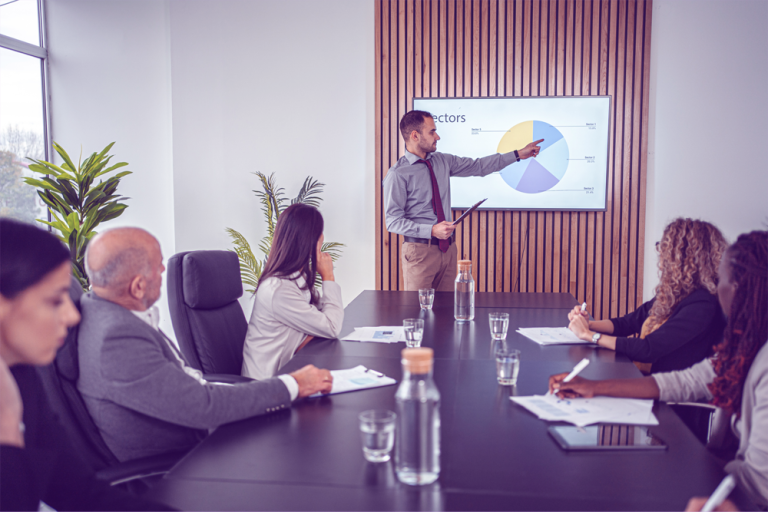
Modern Data Challenges: 4 Key Considerations in Financial Services
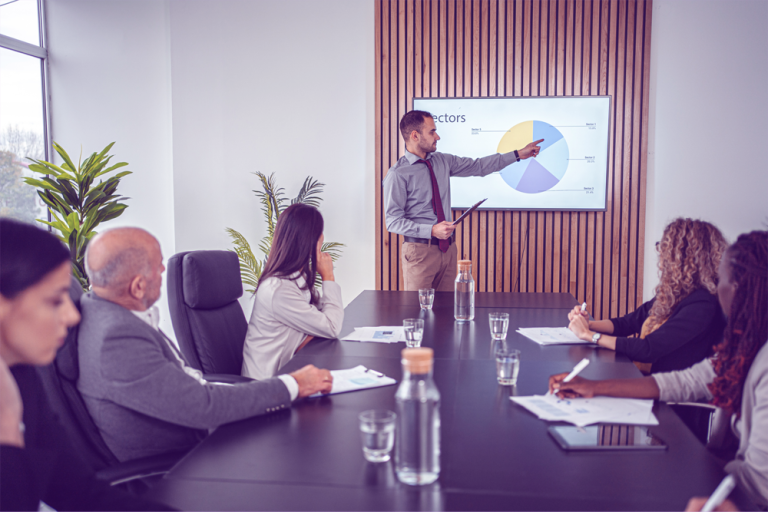
The financial services industry faces an array of challenges in adapting legacy technology to the rapidly evolving technology environment. Data analytics and other technologies have emerged as integral elements of most businesses. Until recently, organizations were forced to choose between the cost and disruption involved with migrating to greenfield systems versus missing the boat on cutting-edge technologies that add value for business users, customers, and shareholders.
Over the past decade, however, speed, scale, and connectivity improved dramatically, opening the door to new approaches that enable companies to accelerate digital transformation while preserving their investments in systems that continue to serve them well.
In a recent webinar hosted by Fintech Nexus, Doug Lopp, CTO of Bend by FNBO, remarked on the role of technology in today’s financial services organizations: “Business and technology are starting to align much more closely than they ever have before, to the point where they are indistinguishable. Understanding why that’s happening, and how that merge is occurring, is a good learning opportunity.”
IT departments are no longer ancillary service providers within the business, sitting alongside accounting and human resources. Corporate technology teams, especially in the financial services sector, are increasingly populated by business-savvy MBAs who understand how technology can truly drive the business forward.
Read our eBook
TDWI Checklist Report: Best Practices for Data Integrity in Financial Services
To learn more about driving meaningful transformation in the financial service industry, download our free ebook.
That inevitably raises the question of how to innovate rapidly and build sustainable systems that can serve the company well today, and in the future. Lopp asks: “How do we go about moving forward and leveraging technology and data in a way that can take us into the next decade?”
Within this context, financial services industry experts point to four critical challenges that business and technology leaders must address:
- Lack of understanding and collaboration across new data teams
- Struggles with building and trusting a single view of critical data
- Risk around drift, shift, and data on the move
- Inefficient operational processes to realize maximum value
Lack of Understanding and Collaboration
Many organizations initially approach data analytics and integration projects strictly from a technology perspective. They seem to believe, as the old movie quote says, that “If we build it, they will come.”
In practice, transformational projects require close attention to the human element. Corporate culture plays an essential role in this respect. Successful projects require strong executive buy-in, but they also demand that everyone in the organization be willing to engage in cross-functional collaboration, built around a common understanding of business objectives, key challenges, and proposed solutions.
Doug Lopp recounts his experience rolling out a transformational project at First National Bank of Omaha. “For us, the key to success was a ‘no fear’ approach. It calls for a team of teams. We started to learn the importance of data and integration, and how to use that data to better run our business. At the end of the day, we realized what made us successful–all of these teams coming together.”
You must have a common vision around which those teams can come together–a common understanding of strategic priorities and near-term business objectives.
Building a Trusted Single View of Critical Data
Most organizations are at least somewhat aware of problems with data quality and accuracy. As they mature, technology teams tend to shift from a narrow focus on data quality to a big-picture aspiration to build trust in their data.
Data integrity begins with integration, which eliminates silos and provides a unified perspective on the business. Without that single view, business users struggle with disjointed information that gives them an obstructed picture of reality.
Automated data quality tools offer a means of correcting errors and inconsistencies at scale, but trust does not end there. Data enrichment and location intelligence provide much deeper context. Together, these tools significantly enhance the overall business value of any data analytics program.
The Risks of Drift, Shift, and Data on the Move
Technology leaders must be ever mindful of Doug Lopp’s assertion that systems should be designed to serve the organization’s interests for years (or even decades) to come. Building a trusted single view of critical data is complicated by the fact that in many organizations, new systems come online with increasing frequency, just as old ones are being mothballed.
That creates new challenges in data management and analytics. Each new system comes with its own schema, which must be mapped and normalized alongside other data. The best integration tools make it easy to build and deploy data pipelines to accommodate the ever-changing needs of modern financial services organizations.
Data quality is challenging in the context of frequently changing systems. To successfully manage data quality at scale, financial services organizations need automated tools to catalog and profile data, define clear business rules, and alert users to issues as soon as they emerge.
Inefficient Operational Processes
Siloed data and disjointed processes inevitably produce friction, leading to inefficient processes, manual workarounds, poorly informed decisions, or simply a lack of visibility. The end result is inefficiency in the organization’s operational processes.
Twenty years ago, top-performing organizations were using “extract, transform, load” (ETL) processes to normalize and aggregate data for business analytics. Transfer speeds of 2,000 transactions per minute might have been considered fast in those days, but today’s IT experts would almost certainly consider such performance to be unacceptably slow. Real-time data is the goal.
Cloud computing technology improves the speed and scale at which organizations can process data. Streaming data pipelines replicate database activity in real time, vastly increasing the speed at which the business can operate.
Modernization projects in the financial services industry sector require data management solutions to improve collaboration and understanding between data consumers, data producers, and data managers. As these organizations set out to implement game-changing technologies, challenges in data integrity require focused attention.
To learn more about driving meaningful transformation in the financial services industry, download the TDWI Checklist Report: Best Practices for Data Integrity in Financial Services.