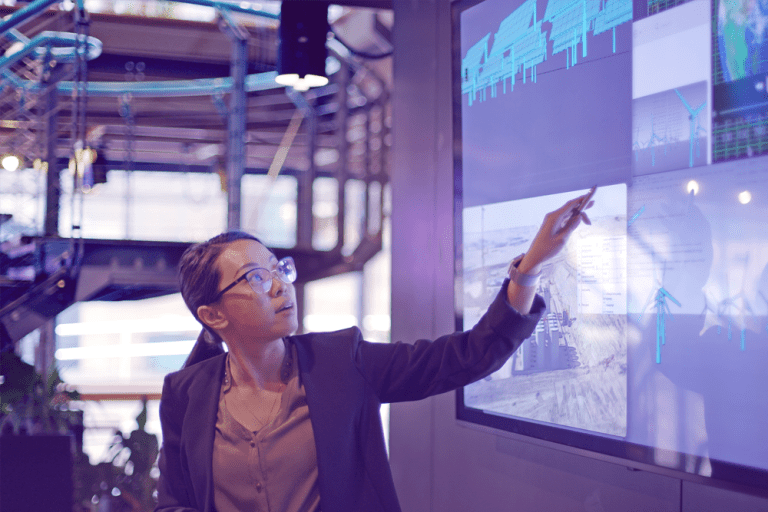
From “Junk Data” to Data Integrity
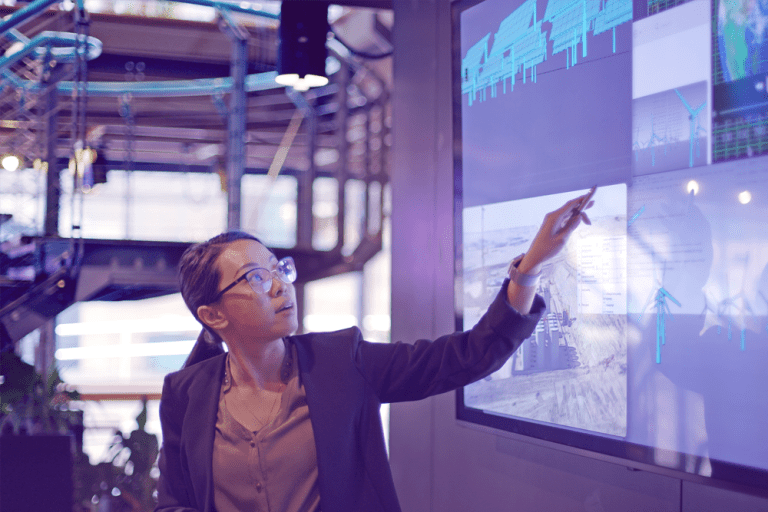
Businesses around the world are creating new data at a faster pace than ever before. According to IDC, the amount of new and replicated data grew even more in 2020 due to the sudden increase in digital interactions. A vast number of people shifted to remote work and remote learning, and they increased the percentage of shopping they do online. Consequently, they generated a lot more data. It’s predicted that the total amount of data generated over the next five years will more than double the total volume of data ever created in the past.
But what should you do with all of that data? It is useful, or is it merely a growing collection of “junk” that serves no distinct purpose? The answer depends on how organizations are actually using the data. Those that approach data integrity from a strategic perspective will find value. In fact, if they look hard enough, they’re likely to find game-changing value in it. Those who do a poor job managing their data, or who simply gather and store it without any definite sense of purpose, will find that they might as well be collecting junk.
What Exactly Is Junk Data?
Let’s begin by framing this conversation, that is, by defining what we actually mean by “junk data”. Perhaps more importantly, we should begin by explaining what junk data is not. Original data, that is, data generated internally, but is not junk.This includes the information stored in transactional systems such as ERP, or from products, devices, and other sources. Typically, this kind of information is governed to some extent, even if your company doesn’t have formal data governance systems in place.
Junk data, in contrast, is not governed. It often emerges when someone makes a copy of data from some primary source, then manipulates it for a particular purpose. In this instance, there’s no attempt to write back to the original database with corrections or additions to the source information. This is a bit like having multiple copies of files that accumulate on your hard drive.The problem with junk data, though, is that it happens at scale. When multiple users make copies, then manipulate that information without syncing it back to its source, you can end up with a fairly large collection of junk.
Read our Report
Data Integrity Trends: Chief Data Officer Perspectives
To learn more about how your organization can build a foundation for trusted data, download the free analyst report.
Imagine that you keep an official list of customers and customer addresses in your ERP system. Your VP of Sales pulls a subset of those customers, filtering out only those that are based in the Denver area. The regional sales team updates that data, but never writes their changes back to the source ERP system. You now have junk data. The end result may be missing information, inaccurate information, outdated data, and/or duplicate records.
With junk data, you have no visibility to its lineage; you don’t know who changed it, when they changed it, or why. It can’t easily be accessed by users across the organization. Worse yet, your organization is forced to grapple with multiple one-off datasets; you end up with “multiple versions of the truth and no one is ever quite sure which one is correct.
Why Is Junk Data Such a Big Problem?
Just as importantly, you’ll undoubtedly face some of the many problems that junk data brings with it:
Inconsistent conclusions: Imagine that you’re analyzing some basic customer profiles for a particular region. How do they break down by state, or perhaps by metro area? Now imagine that some of that data is missing from your original source. Someone makes a copy of the data, adds a field for “metro area”, populates most of that information based on zip codes, then fills in any missing values manually. Then you analyze that information to determine the best media strategy for reaching a lookalike audience in the same region.If you run the same report from your source data, using zip codes to map locations to metro areas, you’ll get a different result. Which one is correct? When you have multiple versions of the truth, it’s hard to have confidence in your data. You’ll end up with different match rates, potential operational failures, and even bad customer experiences, depending on how you’re using the data.
Inaccurate results: Now imagine the same scenario, except in this case the marketing team relies on data that was exported several months ago. The old datasets fail to reflect some important new developments, including the launch of a new product line that appeals to a more cost-conscious audience. Because they’re working with an outdated dataset, they don’t have a complete, up-to-date view of the customer base. That means any conclusions that they draw from the data will be inaccurate.
Privacy concerns: When users make copies of data that contain confidential information, your organization is at risk of running afoul of regulatory agencies. GDPR, CCPA, and other privacy regulations lay out strict requirements with respect to the retention of personally identifiable information. If you’re out of compliance, it can lead to fines and/or reputational damage. Top management is usually blind to this kind of risk exposure until after a problem has occurred.
Information security: Wherever there is a possibility for junk data to be created, there is a risk that information security will be compromised. Security breaches commonly occur from the inside. That often includes the theft of customer lists, proprietary product information, or the disclosure of personally identifiable information.
Financial implications: Whenever junk data emerges within your organization, it creates risks and inefficiencies. That can lead to poor business decisions, fines and penalties, reputational damage, and more. All of the above have negative financial consequences.
An erosion of trust: Perhaps the biggest problem that emerges as a result of junk data is the erosion of trust in data-driven insights. When data integrity is lacking, business users lose confidence in their capacity to drive smarter business decisions from it.
On the other hand, when an organization proactively builds data integrity, it can empower its users with accurate, consistent, contextual data that allows for better decisions and drives competitive advantage. Data integrity is built on four key pillars: integration, data quality, location intelligence, and data enrichment. Together, these provide a foundation for trust. Information is complete, accurate, consistent, and available. It provides rich context that helps business users fully understand its meaning.
From Junk Data to Data Integrity
The best way to deal with junk data is to eliminate the need to produce it in the first place. When data integrity exists at the outset, business users no longer need to generate standalone copies. By investing in data integrity from the outset, organizations can ensure that their data assets are secure, available to the right users, and are adding strategic value to the business.
To learn more about how your organization can build a foundation for trusted data, download the free analyst report, Data Integrity Trends: Chief Data Officer Perspectives.